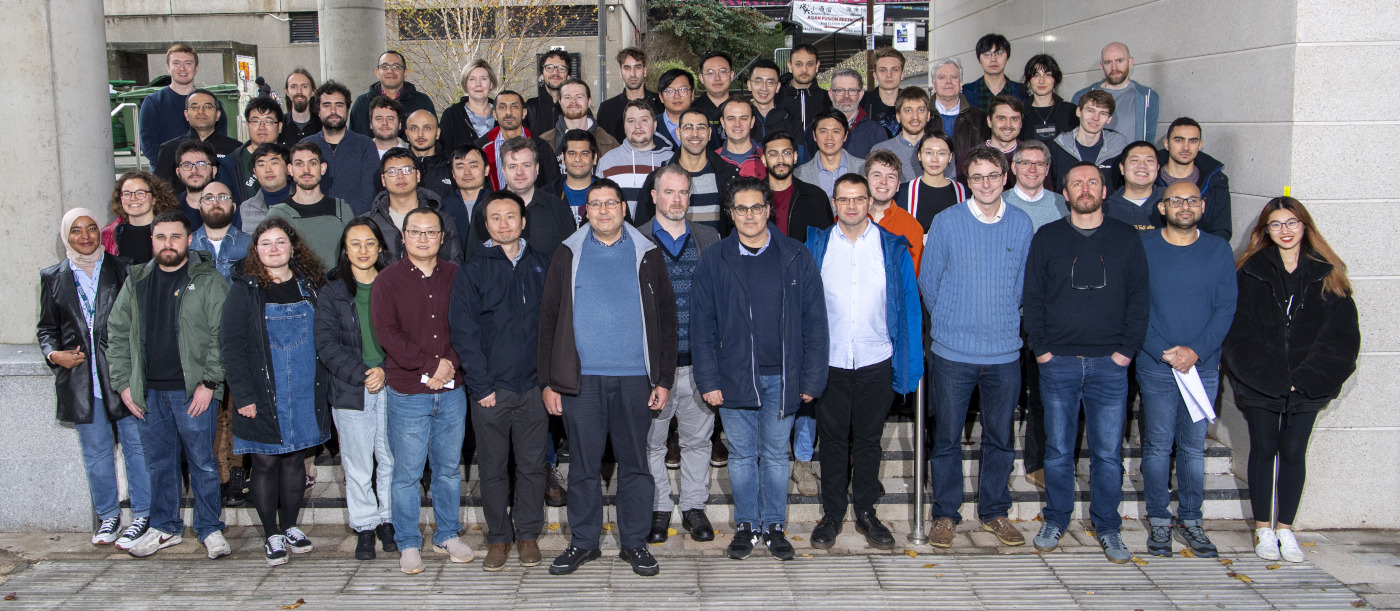
Information, Data & Analysis (IDA) Section
Overview
Technological advances in sensing, data acquisition, mobile devices and the impact of the Internet are leading to increasing amounts of data sampled more rapidly and comprehensively than ever before. If we are to acquire novel insights and knowledge from this data, it needs to be matched by innovations in data management, storage and retrieval and ultimately in data analytics. The many forms of data, their complexity and variations present challenges from information and data systems, to algorithms and inference about patterns through modelling, leading to visualisation, communication and human-computer interaction.
The Information, Data and Analysis Section is led by Professor Iadh Ounis, and has 31 academics, and 53 Post-Doctoral Fellows, Research Assistants and Ph.D. students active in this area. Our research is organised in four world-leading groups in data systems, human-computer interaction & machine learning, information retrieval, and computer vision & autonomous systems:
- KDES - Knowledge & Data Engineering Systems Group
(Dr Nikos Ntarmos, Dr Christos Anagnostopoulos) - IDI - Information, Dynamics and Interaction
(Prof. Roderick Murray-Smith, Dr John Williamson, Dr Ke Yuan, Dr Fani Deligianni, Dr Nicolas Pugeault, Dr Jonathan Grizou, Dr Chris McCaig, Dr Kevin Bryson, Dr Paul Henderson, Dr Ali Gooya, Dr Hang Dai) - IR - Information Retrieval
(Prof. Iadh Ounis, Prof. Joemon Jose, Prof. Craig MacDonald, Dr Jeff Dalton, Dr Richard McCreadie, Dr Graham McDonald, Dr Chris McCaig, Dr Debasis Ganguly, Dr Jake Lever, Dr Sean MacAvaney, Dr Zaiqaio Meng) - CVAS - Computer Vision and Autonomous Systems
(Dr Paul Siebert, Dr Gerardo Aragon Camarasa, Dr Nicolas Pugeault, Dr Paul Henderson, Dr Ali Gooya, Dr Edmond Ho, Dr Hang Dai)
News
February 2013: The Tony Kent Strix Award – 8th Annual Memorial Lecture
Professor Iadh Ounis will present his award lecture on Thursday 23rd February 2023.
Register now to attend a free afternoon of Zoom presentations from 2.30pm to 5.30 p.m.
Registration link: https://www.cilip.org.uk/events/EventDetails.aspx?id=1710573&group=201314
Dr. Ryen White (Glasgow IR Group Alumni) will also be presenting his award presentation.
December 2022: Professor Iadh Ounis was awarded the prestigious international 2022 Tony Kent Strix Memorial Award in recognition of his significant contributions to the field of information retrieval research and development.
Professor Ounis is noted for his sustained contributions to advances in information retrieval, his inspirational leadership, commitment to PhD education, research and contributions to R&D through open-source software and information retrieval tools. The highly valued Terrier and PyTerrier platforms have been utilised extensively across the information retrieval community and advanced research significantly. His work on many information retrieval tasks including expert search models, search results diversification, search ranking, recommendation, fake news detection and query performance prediction has furthered the community’s understanding of some of the most fundamental information retrieval questions.
October 2022: Dr. Graham McDonald (PI), Dr. Jake Lever and Prof. Iadh Ounis secured an EPSRC New Horizons award for their project SIEPH: Safe Information Extraction from Patient Histories. The project will develop machine learning methods for safely extracting useful information from patients’ medical records for medical researchers while maintaining patient privacy.
June 2022: A team of students, led by Dr Jeff Dalton, are the winners of a major international competition to develop next-generation conversational assistants. The GRILL team (grilllab.ai) took first place in Amazon’s Alexa Prize TaskBot Challenge, beating out nine leading research teams worldwide. The competition, launched in June 2021, challenged university students to develop conversational agents that assist people in completing cooking and do-it-yourself home improvement tasks that required multiple steps and complex decisions.
April 2022: Dr. Fani Deligianni (PI) secured an three year (2022-2025) EPSRC New Investigator Award for the project Privacy-Preserved Human Motion Analysis for Healthcare Applications, which will develop artificial intelligence algorithms for human motion analysis in healthcare applications that preserve users' privacy.
November 2020: Dr Jeff Dalton has recieved a prestigious Turing AI Acceleration Fellowship supported by a £20 million government investment (UKRI) to develop smarter, more helpful virtual personal assistants over the next five years. Read more about the award here.
Section members
Academic Staff:
- Professor Iadh Ounis - (Professor of Information Retrieval, Section Leader) (IR)
- Professor Roderick Murray-Smith - (Professor) (IDI)
- Professor Joemon Jose - (Professor of Information Retrieval) (IR)
- Professor Craig MacDonald - (Professor of Information Retrieval) (IR)
- Dr Nicolas Pugeault (Reader) (IDI, CVAS)
- Dr Paul Siebert - (Reader) (CVAS)
- Dr Nikos Ntarmos - (Senior Lecturer) (KDE)
- Dr Christos Anagnostopoulos - (Senior Lecturer in Distributed & Pervasive Computing) (KDE)
- Dr Gerardo Aragon Camarasa - (Lecturer in Autonomous and Socially Intelligent Robotics) (CVAS)
- Dr Jeff Dalton - (Senior Lecturer in Information Retrieval) (IR)
- Dr Richard McCreadie - (Senior Lecturer) (IR)
- Dr John Williamson - (Senior Lecturer) (IDI)
- Dr Ke Yuan - (Senior Lecturer in Machine Learning and Computational Biology) (IDI)
- Dr Graham McDonald - (Lecturer in Information Retrieval) (IR)
- Dr Fani Deligianni (Senior Lecturer in Healthcare & Computing) (IDI)
- Dr Chris McCaig (Lecturer) (IDI, IR)
- Dr Kevin Bryson (Senior Lecturer) (IDI)
- Dr Jonathan Grizou (Lecturer) (IDI)
- Dr Debasis Ganguly (Lecturer in Data Science) (IR)
- Dr Jake Lever (Lecturer) (IR)
- Dr Sean MacAvaney (Lecturer in Machine Learning) (IR)
- Dr Paul Henderson (Lecturer in Machine Learning) (IDI, CVAS)
- Dr Zaiqaio Meng (Lecturer) (IR)
- Dr Ali Gooya (Senior Lecturer) (CVAS, IDI)
- Dr Edmond Ho (Senior Lecturer) (CVAS)
- Dr Hang Dai (Lecturer) (IDI, CVAS)
Research Staff:
- Catherine Higham
- Chaitanya Kaul
- Sham Puthiya
- Andrew Ramsay
- Javier Sanz-Cruzado Puig
- Alessandro Speggiorin
- Sebastian Stein
- Aurelien Nioche
- Richa Agarwal
- David Jasek
- Omar Elnaggar
- Erlend Frayling
- Mingcan Tang
- Valentin Charvet
- Shubham Chatterjee
Honorary Research Fellow:
- Professor John Soldatos
Research Students:
- Maha Al-Anqoudi
- Ali AlQallaf
- Florent Audonnet
- Marco Aversa
- Talha Enes Ayranci
- Tanatta Chaichakan
- Andreas Chari
- Valentin Charvet
- Li Duan
- Xuri Ge
- Carlos Gemmell
- Ozgu Goksu
- Zhuo He
- JingMinn Huang
- Thomas Jänich
- Sarawoot Kongyoung
- Zijun Long
- Zeyuan Meng
- Richard Menzies
- Josh Mitton
- Hitarth Narvala
- Paul Ogbonoko Owoicho
- Piotr Ozimek
- Aleksandr Petrov
- Nikos Pitsillos
- Anith Ravindran
- Federico Rossetto
- Martin Sablotny
- Dominik Szczepaniak
- Evdoxia Taka
- Xiao Wang
- Yaxiong Wu
- Songpei Xu
Events this week
Enhancing the Search Experience on Complex Search Scenarios
Group: Information Retrieval (IR)
Speaker: Jorge Gabin, University of A Coruña
Date: 29 April, 2024
Time: 15:00 - 16:00
Location: Room 422, SAWB
Title:
Enhancing the Search Experience on Complex Search Scenarios
Abstract:
Search experience (SX) is fundamental to succeed in complex search tasks. However, achieving a great SX in advanced search engines is extremely difficult. The reason behind this is that, on the one hand, the types of users, tasks and information needs are disparate, and, on the other hand, the nature of the information in these search engines and the search possibilities are diverse and complex. In this talk, I will describe our two first works towards enhancing the search experience in complex search scenarios. First, I will present docT5keywords, a keyword generation model based on text-to-text transfer transformers (T5). This model generates descriptive keywords directly from academic documents, offering a fresh perspective on keyword labelling. We compare its performance with the EmbedRank model and manual keyword assignments by authors, highlighting its ability to produce unseen labels and its suitability for exploratory search tasks. Then, regarding our second work, we will introduce two models for the keyword suggestion task trained on scientific literature. These models adapt the architecture of Word2Vec and FastText to generate keyword embeddings, leveraging keyword co-occurrence patterns in academic publications. Alongside these models, we will present a specially tailored negative sampling approach that enhances keyword suggestion accuracy. Our evaluation methodology includes ranking-based assessments in both known-item and ad-hoc search scenarios, demonstrating significant improvements over existing word and sentence embedding models.
Bio:
I am a third-year PhD candidate at the Information Retrieval Lab, University of A Coruña, concurrently engaged as a researcher at Linknovate. My primary focus is enhancing search and user experience within complex search scenarios. Specifically, my research involves developing models to aid users in query formulation and refining ranking algorithms with keyphrase search scenarios. Before diving into research, I also worked as a software engineer at a multinational textile company.
Upcoming events
Enhancing the Search Experience on Complex Search Scenarios
Group: Information Retrieval (IR)
Speaker: Jorge Gabin, University of A Coruña
Date: 29 April, 2024
Time: 15:00 - 16:00
Location: Room 422, SAWB
Title:
Enhancing the Search Experience on Complex Search Scenarios
Abstract:
Search experience (SX) is fundamental to succeed in complex search tasks. However, achieving a great SX in advanced search engines is extremely difficult. The reason behind this is that, on the one hand, the types of users, tasks and information needs are disparate, and, on the other hand, the nature of the information in these search engines and the search possibilities are diverse and complex. In this talk, I will describe our two first works towards enhancing the search experience in complex search scenarios. First, I will present docT5keywords, a keyword generation model based on text-to-text transfer transformers (T5). This model generates descriptive keywords directly from academic documents, offering a fresh perspective on keyword labelling. We compare its performance with the EmbedRank model and manual keyword assignments by authors, highlighting its ability to produce unseen labels and its suitability for exploratory search tasks. Then, regarding our second work, we will introduce two models for the keyword suggestion task trained on scientific literature. These models adapt the architecture of Word2Vec and FastText to generate keyword embeddings, leveraging keyword co-occurrence patterns in academic publications. Alongside these models, we will present a specially tailored negative sampling approach that enhances keyword suggestion accuracy. Our evaluation methodology includes ranking-based assessments in both known-item and ad-hoc search scenarios, demonstrating significant improvements over existing word and sentence embedding models.
Bio:
I am a third-year PhD candidate at the Information Retrieval Lab, University of A Coruña, concurrently engaged as a researcher at Linknovate. My primary focus is enhancing search and user experience within complex search scenarios. Specifically, my research involves developing models to aid users in query formulation and refining ranking algorithms with keyphrase search scenarios. Before diving into research, I also worked as a software engineer at a multinational textile company.
Francesco Busolin IR Seminar
Group: Information Retrieval (IR)
Speaker: Francesco Busolin, Università Ca' Foscari Venezia
Date: 13 May, 2024
Time: 15:00 - 16:00
Location: Room 422, SAWB
Title:
TBC
Abstract:
TBC
Bio:
TBC
Ferdinand Schlatt IR Seminar
Group: Information Retrieval (IR)
Speaker: Ferdinand Schlatt, Friedrich Schiller University Jena
Date: 03 June, 2024
Time: 15:00 - 16:00
Location: Room 422, SAWB
Title:
TBC
Abstract:
TBC
Bio:
TBC
Yanran Tang IR Seminar
Group: Information Retrieval (IR)
Speaker: Yanran Tang, University of Queensland
Date: 17 June, 2024
Time: 15:00 - 16:00
Location: Room 422, SAWB
Title:
TBC
Abstract:
TBC
Bio:
TBC
Past events
On diffusion models generating 3D objects and scenes (26 April, 2024)
Speaker: Dr Paul Henderson
Abstract:
I will talk about how to build diffusion models that generate 3D objects and scenes, and how to train these from 2D image data alone. This will be part research talk (presenting some of my state-of-the-art results in this area), and part tutorial (explaining the design decisions we must make when designing such models).
Bio:
Paul is a Lecturer in Machine Learning, specialising in probabilistic methods for computer vision, particularly generative models and 3D reconstruction.
Grounded and Transparent Response Generation for Conversational Information-Seeking Systems (22 April, 2024)
Speaker: Weronika Lajewska
Title:
Grounded and Transparent Response Generation for Conversational Information-Seeking Systems
Abstract:
While previous conversational information-seeking (CIS) research has focused on passage retrieval, reranking, and query rewriting, the challenge of synthesizing retrieved information into coherent responses remains. My research delves into the intricacies of response generation in CIS systems. Open-ended information-seeking dialogues introduce multiple challenges that may lead to potential pitfalls in system responses. The presented studies focus on generating responses grounded in the retrieved passages and being transparent about the system's limitations. Specific research questions revolve around obtaining confidence-enriched information nuggets, automatic detection of incomplete or incorrect responses, generating responses communicating the system's limitations, and evaluating enhanced responses. By addressing these research tasks, the presented work aspires to contribute to the advancement of conversational response generation, fostering more trustworthy interactions in CIS dialogues, and paving the way for grounded and transparent systems to meet users’ needs in an information-driven world.
Bio:
I am a third year Ph.D. student at The Information Access and Artificial Intelligence Research Group at the University of Stavanger, supervised by Krisztian Balog. My research interests lie in the intersection of information retrieval, natural language processing and human-computer interactions. I'm particularly interested in explainable and transparent conversational search.
Physics Properties Estimation of Deformable Objects (19 April, 2024)
Speaker: Yingdong Ru
Abstract:
Deformable object manipulation is a challenging task for robotic systems as the objects can change their form, size, and position during manipulation, and reinforcement learning is the best tool now to solve this task. However, the model needs to be trained in simulation at first so the input of the physical parameter of the deformable object in the simulation needs to be as same as possible compared with the real object, therefore closing the sim-to-real gap. Many previous papers in this field have mentioned the difficulty of predicting the physics parameter of real fabrics and garments. In this presentation, I will give a basic overview of these papers and my current work.
Speaker Bio:
Yingdong Ru is a second-year Ph.D. student supervised by Dr. Gerardo Aragon Camarasa. His research focuses on physics parameter estimation of garments and Robotic Manipulation.
Learning from Reformulations for Conversational Question Answering (15 April, 2024)
Speaker: Magdalena Kaiser
Title:
Learning from Reformulations for Conversational Question Answering
Abstract:
Models for conversational question answering (ConvQA) are usually trained and tested on benchmarks of gold QA pairs. In our first contribution, we take a step towards a more natural learning paradigm – from noisy and implicit feedback via question reformulations. A reformulation is likely to be triggered by an incorrect system response, whereas a new follow-up question could be a positive signal on the previous turn’s answer. We present a reinforcement learning model, termed CONQUER, that can learn from a conversational stream of questions and reformulations. Experiments show that CONQUER successfully learns from noisy rewards, significantly improving over a state-of-the-art baseline. In our second contribution, we propose a framework, termed REIGN, which takes several steps to remedy this restricted learning setup where training is limited to surface forms seen in the respective datasets and evaluation is on a small set of held-out questions. We systematically generate reformulations of training questions to increase robustness of models to surface form variations. Then, we guide ConvQA models towards higher performance by feeding it only those reformulations that help improve their answering quality, using deep reinforcement learning. Finally, for a rigorous evaluation of robustness for trained models, we use and release large numbers of diverse reformulations generated by prompting GPT for benchmark test sets (resulting in 20x increase in sizes). Our findings show that ConvQA models with robust training via reformulations significantly outperform those with standard training from gold QA pairs only.
Bio:
Magdalena Kaiser is a PhD Student in the Databases and Information Systems Group at the Max Planck Institute for Informatics (MPII), Saarbrücken, Germany, under the supervision of Prof. Gerhard Weikum and Dr. Rishiraj Saha Roy. Her research focuses on conversational question answering. In particular, she is interested in leveraging feedback to improve conversational systems. In her work, she applies techniques from Information Retrieval, Natural Language Processing and Machine Learning, particularly Reinforcement Learning. Prior to joining MPII, she worked as a researcher at the German Research Center for Artificial Intelligence (DFKI) for two years. She obtained her Master's degree from Saarland University and her Bachelor's degree from University Erlangen-Nürnberg, Germany.
A Deep Learning Solution to Optimise the Control of Tidal Stream Conversion Devices (12 April, 2024)
Speaker: Oliver Summerell
Abstract:
Around the coast of the UK there is an estimated 30-50GW of energy that goes untapped in our tides. However, despite the fact that it is far more reliable than any other form of renewable energy, it contributes to less that 3% of our total energy production, predominantly due to the cost of technology attributed to having to survive the underwater conditions. Many projects don't get past the initial stages of design due to the time required, which is where this project comes in. The aim of my PhD is to explore the potential of the use of Deep Learning to streamline the initial design process of a Tidal Stream Turbine farm to lower cost and get more turbines out and contributing to the grid. To do this, the current focuses are Physics Informed Neural Networks (PINNs) and Graph Neural Networks (GNNs), both of which will be covered in this presentation.
Speaker Bio:
Oliver Summerell is a first year PhD student with a background in Aero-Mechanical Engineering (MEng) from Strathclyde, originally from Leeds.
An encoder-centric view of retrieval (08 April, 2024)
Speaker: Andrew Yates
Title
An encoder-centric view of retrieval
Bio
Andrew Yates is an Assistant Professor at the University of Amsterdam, where his research focuses on developing content-based neural ranking methods and leveraging them to improve search and downstream tasks. He has co-authored a variety of papers on neural ranking methods as well as a book on transformer-based neural methods: "Pretrained Transformers for Text Ranking: BERT and Beyond". Previously, Andrew was a post-doctoral researcher and senior researcher at the Max Planck Institute for Informatics. Andrew received his Ph.D. in Computer Science from Georgetown University, where he worked on information retrieval and extraction in the medical domain.
Presenting TELESIM and Feedback on my Japan Internship (05 April, 2024)
Speaker: Florent Audonnet
Abstract:
I will be first presenting my paper TELESIM which has been accepted at ICRA 2024 and then expand on the research I did in Japan as well as the lifestyle and places I explored.
Teleoperating robotic arms can be a challenging task for non-experts, particularly when using complex control devices or interfaces. To address the limitations and challenges of existing teleoperation frameworks, such as cognitive strain, control complexity, robot compatibility, and user evaluation, we propose TELESIM, a modular and plug-and-play framework that enables direct teleoperation of any robotic arm using a digital twin as the interface between users and the robotic system. Due to TELESIM's modular design, it is possible to control the digital twin using any device that outputs a 3D pose, such as a virtual reality controller or a finger-mapping hardware controller. To evaluate the efficacy and user-friendliness of TELESIM, we conducted a user study with 37 participants. The study involved a simple pick-and-place task, which was performed using two different robots equipped with two different control modalities. Our experimental results show that most users were able to succeed by building at least a tower of 3 cubes in 10 minutes, with only 5 minutes of training beforehand, regardless of the control modality or robot used, demonstrating the usability and user-friendliness of TELESIM.
Bio:
I am a 3rd year PhD student under the supervision of Dr. Gerardo Aragon-Camarasa. My research is focused on robotic manipulation and VR interaction, especially related to teleoperation.
How to Survive a PhD (22 March, 2024)
Speaker: Piotr Ozimek
Abstract:
The journey of a PhD student is notorious for being difficult and confusing. Having recently completed my PhD, I will use this talk to share high-level and practical advice on how to survive one’s PhD. I will talk about the critical skills most students struggle with, how to improve them, how to deal with your supervisor, and hopefully, how to enjoy your PhD.
Bio:
Piotr Ozimek has an MSci and a PhD in Computer Science, both from the University of Glasgow. He was co-supervised by Paul Siebert and Gerardo Aragon Camarasa and finished his studies in 2023. He dedicated his PhD to studying active vision systems in deep learning. Currently, he is working in the industry as a Senior Research Engineer at Speech Graphics, where he develops deep learning systems for sound event detection and emotion recognition from human speech audio. His research interests include active attention, complexity, state space models and predictive coding.
Product reviews on the web or the struggle of search engines with affiliate spam (18 March, 2024)
Speaker: Janek Bevendorff
Title:
Product reviews on the web or the struggle of search engines with affiliate spam
Automated use of 3D data in forensic odontology identification (15 March, 2024)
Speaker: Anika Kofod Petersen
Abstract:
Forensic odontology identification (comparative dental analysis) is one of the three primary identifiers in disaster victim identification. With the addition of intraoral 3D photo scans to dental records, a new level of detail awaits implementation in the identification process. Such implementation requires development of a matching algorithm. For such an algorithm to be useful in a forensic setting, distinguishment between 3D photo scans from the same individual, opposed to scans from different individuals, is fundamental. But how can such matching algorithms be made? What methods would suit the world of forensics, and what techniques can distinguish between dentitions, even when the dentition have been subject to trauma?
Bio:
I have a B.sc. in Human Life Science Engineering from the Technical University of Denmark (DTU) where I developed algorithms for identifying biosynthetic gene clusters in bacteriophage DNA. I have a double M.sc. shared between DTU (M.Sc. Life Science Engineering and informatics) and the University of Chinese Academy of Sciences (UCAS) (M.Sc. Biochemistry and Molecular Biology). This combination is also known as the M.sc. of Omics. Now, as a PhD student at the department of Forensic Medicine, Aarhus University, I am working on exploring more advanced data structures than sequence data, 3D dental surfaces. This allows an exploration of multidimensional data representation and data preparation for machine learning and sets larger demands for computational power management. I work with (and live by) 3 keywords: automation, optimization, and creativity.
Learned Sparse Retrieval (11 March, 2024)
Speaker: Sean MacAvaney
Title: Learned Sparse Retrieval
Abstract: Learned Sparse Retrieval methods, such as DeepCT, EPIC, and SPLADE, represent highly effective and efficient alternatives to the dense retrieval paradigm. In this talk, I introduce the key techniques and key components that the methods use. I then break down results from our recent paper to disentangle the effects of each technique on final retrieval effectiveness and efficiency -- and how they relate to one another.
Bio: Sean is a Lecturer in Machine Learning at the University of Glasgow and a member of the Terrier Team. His research primarily focuses on effective and efficient neural retrieval. He completed his PhD at Georgetown University in 2021, where he was a member of the IR Lab and an ARCS Endowed Scholar. He was a co-recipient of the SIGIR 2023 Best Paper Award and the ECIR 2023 Best Short Paper Award.
Self-supervised Federated Learning in Vision (08 March, 2024)
Speaker: Ozgu Goksu
Abstract:
This presentation highlights the combination of self-supervised learning and federated learning, unlocking their collective potential for advancing computer vision tasks. Inspired by https://arxiv.org/pdf/1602.05629.pdf, we explore how this approach leverages the abundance of unlabelled data while maintaining robust data privacy. Self-supervised learning allows us to extract valuable knowledge from this unannotated data, while federated learning enables collaborative training across models without sharing data. This presentation gives insights into the core concepts, fundamental keywords and how I am planning to use them in my research.
Bio:
Ozgu is currently in the third year of her PhD, under the supervision of Dr. Nicolas Pugeault. Her research focuses on the development of methodologies for self-supervised and unsupervised batch curation.
Uncertainty in Deep Neural Networks (01 March, 2024)
Speaker: Dr Edmond S. L Ho
Abstract:
Deep neural networks have been widely used in most of the ongoing research in Computer Vision and the areas we, the CVAS group, are interested in. While we usually focus on evaluation metrics such as classification accuracy to compare the performance of different approaches, for some serious applications (for example, medical image analysis) we would also like to know if we can/should trust the output of the model. In this talk, I will give an introduction to Uncertainty in Deep Neural Networks with some recent work published at CVPR and ICCV.
Bio:
Edmond Shu-lim Ho is currently a Senior Lecturer (Associate Professor) in the School of Computing Science (IDA-Section) at the University of Glasgow, Scotland, UK. Prior to joining the University of Glasgow in 2022, he was an Associate Professor in the Department of Computer and Information Sciences at Northumbria University, Newcastle upon Tyne, UK (2016-2022) and a Research Assistant Professor in the Department of Computer Science at Hong Kong Baptist University (2011-2016). He has been an Associate Editor of Computer Graphics Forum (CGF) since 2023. He received the BSc degree in Computer Science from the Hong Kong Baptist University, the MPhil degree from the City University of Hong Kong, and the PhD degree from the University of Edinburgh.
His research interests include Computer Graphics, Computer Vision, Biomedical Engineering, and Machine Learning.
Advancing Legal Intelligence: Conversational Models, Expertise Discovery, and Question Answering in Legal QA Systems (26 February, 2024)
Speaker: Arian Askari
Title:
Advancing Legal Intelligence: Conversational Models, Expertise Discovery, and Question Answering in Legal QA Systems
Abstract:
In this talk, I delve into our recent works focused on the legal community question answering systems. These projects tackle tasks such as conversational legal search, legal answer retrieval, and legal expert finding tasks. I will start with CLosER, short for Conversational Legal Longformer with Expertise-Aware Passage Response Ranker. This method, which we introduced at CIKM 2023, is designed to address conversational legal search effectively by handling the long legal dialogues and bridging the knowledge gap between legal professionals and laypersons. Following this, I will talk about our most recent work accepted at ECIR 2024 on improving legal answer retrieval through a cross-encoder re-ranker that leverages structured inputs to dramatically improve the process of identifying relevant legal advice from the collection of advice written by authorized lawyers. Finally, I will talk about our work on expert finding in the legal domain that has been accepted at ECIR 2022, where I introduce our methodology for creating dynamic, query-dependent profiles, resulting in an improvement in the effectiveness of identifying the right legal expert for specific queries. As future work, inspired by our recent works for utilizing LLMs for general information retrieval (IR) and NLP, I will discuss my perspective on the potential future direction of legal IR in the era of large language models.
Bio:
Arian Askari is a Ph.D. candidate and Marie Skłodowska-Curie Research Fellow at Leiden University. His research centers on large language models, emphasizing their role in information retrieval. He has published multiple papers presented at prestigious conferences during his Ph.D. and MSc. Previously, his focus was on developing effective Transformer-Based retrievers for both domain-specific and web search. Currently, his passion lies in pushing the boundaries of information retrieval through the capabilities of LLMs, translating advancements into practical applications for real-world issues.
Exploring Medical Image Segmentation with Fully Convolutional Vision Transformers (23 February, 2024)
Speaker: Chaitanya Kaul
Abstract:
Vision Transformers have been applied to various domains of computer vision applications. Challenges posed by the fine-grained nature of medical image analysis mean that the adaptation of the transformer for their analysis is still at nascent stages. The overwhelming success of the encoder decoder architecture like UNet, lay in its ability to appreciate the fine-grained nature of the segmentation task, an ability which most existing transformer based models do not currently implicitly posses. In this talk, I will go through our recent works [1] [2] [3] to address this shortcoming of transformer models for medical image segmentation tasks showing how inductive bias towards images can be introduced to transformers to learn long range semantic dependencies inside them, and how such feature dependencies can be processed for effective, faster, segmentation of CT, MRI and RGB modalities.
References:
[1] Liu, Q., Kaul, C., Wang, J., Anagnostopoulos, C., Murray-Smith, R. and Deligianni, F., 2023, June. Optimizing Vision Transformers for Medical Image Segmentation. In ICASSP 2023-2023 IEEE International Conference on Acoustics, Speech and Signal Processing (ICASSP) (pp. 1-5). IEEE.
[3] GLFNet: Global-Local (Frequency) Filter Networks for efficient Medical Image Segmentation. (Accepted at, ISBI 2024)
Bio:
Dr. Chaitanya Kaul is a Research Associate in the Inference Dynamics and Interaction Group, at School of Computing Science, University of Glasgow, working under Prof. Roderick Murray-Smith. He is currently funded by Google, and QuantIC, working on 3D Computational Imaging problems where he investigates how unconvential imaging sensors like radars and SPADs can be used for 3D scene understanding and 3D scene interaction. He was previously funded by iCAIRD where he investigated adversarial testing of machine learning algorithms to understand feature leakage in medical imaging applications. His research interests are in Computational Imaging, Medical Image Segmentation and 3D Shape Analysis.
Halting Climate Change (19 February, 2024)
Speaker: Professor Carl Rasmussen
Addressing climate change is essentially a problem of international cooperation. The necessary properties of successful cooperative schemes are well understood, but our main current international approaches, such as the Paris Agreement, have none of these properties, and are consequently extremely unlikely to succeed. You may think that the whole problem is simply completely intractable, but I think not. I’ll discuss a simple proposal eliminating the main shortcomings of the Paris Agreement, and aspects of how it might be implemented in practice.
This is a very informal talk, which will hopefully generate a lot of discussion. Some related ideas are discussed here: https://mlg.eng.cam.ac.uk/carl/climate/
Avoid the Avoidable when Ranking (19 February, 2024)
Speaker: Francesco Busolin
Title:
Avoid the Avoidable when Ranking
Abstract:
Ranking is a crucial step in many information retrieval tasks. In recent years, machine learning has been heavily integrated into large-scale search platforms to solve the ranking problem. Unfortunately, state-of-the-art solutions often have a high computational cost, and their deployment in production environments has a considerable impact on response time and throughput.
Almost all the known techniques devised to reduce the cost of using such large ranking models try to reduce the rankers' size or limit the volume of data to be processed by the model. In this talk, we will discuss the Early Exit technique, where during the evaluation, we decide whether to continue or interrupt the scoring of a document if it is deemed irrelevant to the given query. We present the opportunities and drawbacks of such approaches, and some learned and non-learned proposals in the literature.
Bio:
Francesco Busolin is a Ph.D. student at Ca Foscari University of Venice under the supervision of Professor Salvatore Orlando and a Research Associate with the Italian National Research Council (CNR) in which he collaborates with the High-Performance Computing Laboratory (HPC Lab) inside the Institute of Information Science and Technologies "Alessandro Faedo."
His primary research field is Information Retrieval, particularly Efficient Learning To Rank. His doctoral research aims to reduce the effort ranking pipelines need to perform to obtain adequate results to present to the final users.
EPSRC Impact Acceleration Account (IAA) – Funding Success Sharing Session @ IDA (16 February, 2024)
Speaker: Ali Gooya, Richard McCreadie and Javier Sanz-Cruzado Puig
EPSRC Impact Acceleration Account (IAA) – Funding Success Sharing Session @ IDA
TL;DR Sharing Session on Fri 16 Feb 14:30-15:30 @ SAWB 422
Do you want to learn more about the recent success in EPSRC IAA funding @ IDA? Have you ever thought about applying for IAA funding to support your Impact and Engagement activities? All Academics and RAs are welcome!
The Engineering and Physical Sciences Research Council (EPSRC) has awarded the University of Glasgow a £3.19m Impact Acceleration Account (IAA) for the period from April 2022 to March 2025. The University uses these funds to increase the global impact through greater levels of external engagement and entrepreneurship (https://www.gla.ac.uk/myglasgow/ris/knowledgeexchange/knowledgeexchangefunding/impactaccelerationaccounts/epsrciaa2022-2025/).
We are pleased to have our colleagues share their experiences and recent success with EPSRC IAA funds (ordered by surname) on the standard and RA-led calls:
- Ali Gooya – “Cloud AI for Cardiac Motion Abnormality Assessment Using Magnetic Resonance Imaging”
- Richard McCreadie – “FAR-Market: Exploration of the Technology Frontier in Financial Asset Recommendation”
- Javier Sanz-Cruzado Puig – “PPC-FI: Personalized Portfolio Construction for Financial Investments” and “FAR-AI: Deployment of AI-based Financial Asset Recommendation System”
We will start with short presentations (10-15 mins) from our colleagues to share their experiences and there will be interactive discussions and Q&A at the end.
Date: Friday 16 Feb 2024
Time: 14:30-15:30
Venue: SAWB 422
See you there!
Representation and Generation within Motion Field Space (16 February, 2024)
Speaker: Shiyu Fan
Abstract:
In the field of data-driven motion generation, encoding motion sequences into a latent space for sampling is a common practice. In this presentation, Shiyu Fan will delve into the various representations of motion within latent spaces and the process of generation from them. In addition, based on his research project, he will also discuss the limitations of these representations and why it is necessary to employ latent diffusion models in neural motion field.
Bio:
Shiyu Fan is currently a second-year PhD student, supervised by Dr Edmond Ho. His research interests lie in motion generation within neural motion field space and multi-person motion synthesis.
A Study of Pre-processing Fairness Intervention Methods for Ranking People (12 February, 2024)
Speaker: Clara Rus
Title:
A Study of Pre-processing Fairness Intervention Methods for Ranking People
Abstract:
Fairness interventions are hard to use in practice when ranking people due to legal constraints that limit access to sensitive information. Pre-processing fairness interventions, however, can be used in practice to create more fair training data that encourage the model to generate fair predictions without having access to sensitive information during inference. Little is known about the performance of pre-processing fairness interventions in a recruitment setting. To simulate a real scenario, we train a ranking model on pre-processed representations, while access to sensitive information is limited during inference. We evaluate pre-processing fairness intervention methods in terms of individual fairness and group fairness. On two real-world datasets, the pre-processing methods are found to improve the diversity of rankings with respect to gender, while individual fairness is not affected. Moreover, we discuss advantages and disadvantages of using pre-processing fairness interventions in practice for ranking people.
Bio:
Clara Rus is a PhD student at University of Amsterdam. She is part of the IRLab and part of the FINDHR project, a European project with a focus on fairness and intersectional non-discrimination in recruitment. Therefore, her research focuses on fairness-aware learning to rank for algorithmic hiring.
Neural Architecture Search: An Introduction (09 February, 2024)
Speaker: Richard Menzies
Abstract:
Neural Architecture Search has seen a great increase in popularity in recent years and while the idea of using machine learning to design a neural network sounds promising, there has been limited uptake of Neural Architecture Search methods, with many novel neural networks simply being deigned by hand: Why is that? Following on from my previous talk, I will summarise the origins of Neural Architecture Search, the developments on the original method and evolutionary architecture search. I will then discuss gradient-based architecture search and the applications, limitations and future work of Neural Architecture Search.
Bio:
Richard is a 2nd year PhD student studying gradient-based Neural Network Topology Optimisation, a sub-field of Neural Architecture Search, supervised by Dr Paul Siebert.
A Topology-aware Analysis of Graph Collaborative Filtering (05 February, 2024)
Speaker: Daniele Malitesta
Title
A Topology-aware Analysis of Graph Collaborative Filtering
TinyFaces: Real-time Detection and Clustering of Small Faces in Videos (02 February, 2024)
Speaker: Ozan Bahadir
Abstract:
This project endeavours to advance real-time detection and clustering methods for individuals in CCTV videos characterized by tiny and noisy images without labels. To achieve precise tiny face detection, we leverage an Integral Fisher Score (IFS)-based approach. Additionally, we introduce an innovative online clustering algorithm that sequentially processes data in short segments of variable length. Faces detected in each segment are dynamically assigned to existing clusters or contribute to the creation of new clusters. This dual methodology ensures the effective clustering of individuals in challenging video scenarios with noisy visual information.
Bio:
Ozan Bahadir is a postdoctoral researcher, focusing on exploring online clustering methodologies tailored for the intricate domain of CCTV videos under the supervision of Dr Tanaya Guha.
Promoting and sustaining accountability in artificial intelligence applications (01 February, 2024)
Speaker: Leonardo Bezerra
Title:
Promoting and sustaining accountability in artificial intelligence applications
Abstract:
Technology has been the catalyst for major revolutions societies have gone through, and each new revolution brings social challenges that governments must address. In turn, regulation acts as a form of feedback that directs how the breakthrough technology of the time will have to be adapted. Currently, the most pressing technology revolution is being powered by social media, big data, and artificial intelligence (AI). Though this revolution has been taking place for over a decade now, recent years have seen an astounding increase in the pace with which these applications are being developed and deployed. Not surprisingly, regulatory agencies around the world have been unable to cope with this speed and have just recently started to move from a data-centred to an AI-centred concern. More importantly, governments are still beginning to mature their understanding of AI applications in general, let alone discuss AI ethics and how to promote and sustain accountability in AI applications. In turn, companies that use AI in their applications have also begun to display some public level of awareness, even if often vague and not substantiated by concrete actions. In this talk, we will briefly overview efforts and challenges regarding AI accountability and how major AI players are addressing it. The goal of the talk is to stir future project collaborations from a multidisciplinary perspective.
Bio:
Dr Leonardo Bezerra joined the University of Stirling as a Lecturer in Artificial Intelligence (AI)/Data Science in 2023, after having been a Lecturer in Brazil for the past 7 years. He received his Ph.D. degree from Université Libre de Bruxelles (Belgium) in 2016, having defended a thesis on the automated design of multi-objective evolutionary algorithms. His research experience spans from applied data science projects with public and private institutions to supervising theses on automated and deep machine learning. Recently, his research has concentrated on the social impact of AI applications, such as disinformation through social media recommendation algorithms and the disruptive potential of generative AI.
Understanding and Managing Uncertainty in Learning from User Interactions for Recommendation (29 January, 2024)
Speaker: Norman Knyazev
Abstract
Uncertainty in learned user preferences due to noisy user feedback can pose a challenge in various recommendation tasks. We start off by looking at the problem of determining item relevance in the presence of the bandwagon effect - a setting where users can see and are influenced by the earlier feedback from other users. Leveraging a previously proposed model of the bandwagon effect, we examine the statistical nature and the impact of the bandwagon effect on relevance estimation, and explore several approaches for mitigating these issues. In the second part of the talk we turn to the classical problem of rating prediction, where most commonly used models do not provide an indication of confidence in their individual predictions. We discuss why it is important to explicitly capture model confidence in addition to user preference, and review our recently proposed simple and lightweight recommendation approach based on Learned Beta Distributions.
Neural Architecture Search: An Introduction (26 January, 2024)
Speaker: Richard Menzies
Abstract:
Neural Architecture Search has seen a great increase in popularity in recent years and while the idea of using machine learning to design a neural network sounds promising, there has been limited uptake of Neural Architecture Search methods, with many novel neural networks simply being deigned by hand: Why is that? In this talk, I will discuss the origins of Neural Architecture Search, the developments on the original method and various alternative techniques which have been proposed, including evolutionary and gradient-based architecture search. I will also highlight some of the limitations of Neural Architecture Search. There are no papers to read for this talk.
Bio:
Richard is a 2nd year PhD student studying gradient-based Neural Network Topology Optimisation, a sub-field of Neural Archiecture Search, supervised by Dr Paul Siebert.
Advances in Sentiment Analysis of the Large Mass-Media Documents (22 January, 2024)
Speaker: Nicolay Rusnachenko
Title
Advances in Sentiment Analysis of the Large Mass-Media Documents
Abstract
Sentiment analysis is a task of authors' opinion extraction towards objects mentioned in text. The constant and rapid growth of information makes manual analysis practically impossible. Initial approaches originating from X/Twitter short text analysis, with texts that usually convey a single opinion towards product or service. However, switching to the larger texts requires advances in more granular analysis. In this talk we overview the advances of machine learning (ML) approaches in sentiment analysis of large mass-media documents. Besides the appearance of the task, we cover the evolution of (i) target-oriented ML architectures, and (ii) training and inference techniques. We highlight the capabilities of the conventional methods and neural networks, followed by the application of the most-recent instructive Large Language Models.
Bio
My name is Nicolay Runsnachenko, and I am Research Assistant at Newcastle University, UK. I defend my PhD in Natural Language Processing. I am majoring in Information Retrieval (IR) with Language Models from large documents of any kind: mass-media articles, news, literature books. I contribute to advances in large document processing in such fields as: Sentiment Analysis, TextSummarization, Dialogue Assistants. At present I am focused on the domain of literature novel books and enhancing dialogue agents with a deeper understanding of fictional characters from literature novel books using Knowledge graph for Empathy Mapping, Personality traits.
Dealing with Typos for Pre-trained Language Model-Based Neural Rankers (18 December, 2023)
Speaker: Shengyao Zhuang
Abstract
The effectiveness of dense retrievers does not scale in presence of queries that contain typos. In this talk, I will show you the reason why this occurs. I will then present our solutions to enhance the robustness of dense retrievers to typos. These solutions consider the whole spectrum of how dense retrievers’ representations are created, with solutions spanning token representation backbone, pre-training, and fine-tuning. Finally, we show that combining our solutions creates a retrieval pipeline based on dense retrievers that is robust to typos and is simpler than integrating state-of-the-art spell-checkers into the retrieval pipeline. While our work has focused on queries that contain typos, we believe that the lessons learnt can generalise to other aspects affecting dense retrievers’ performance when dealing with out-of-distribution data.
Bio
Dr. Shengyao Zhuang is a postdoctoral researcher at CSIRO, Australian e-Health Research Centre, where he focuses on developing large language model-based search engine systems in the medical domain. Before joining CSIRO, Shengyao was a Ph.D. student at the ielab, EECS, The University of Queensland, Australia, supervised by Professor Guido Zuccon. His primary research interests lie in information retrieval, large language model-based neural rankers, and NLP in general.
Trustworthy Recommender Systems (27 November, 2023)
Speaker: Elisabeth Lex
Title
Trustworthy Recommender Systems
Abstract
Recommender systems play a pivotal role in shaping our digital experiences, influencing the content we see online, the products we consider purchasing, and the entertainment choices we make, such as which movies to watch. The increased adoption of deep learning technologies in recommender systems, while enhancing their effectiveness, has also raised substantial concerns regarding their transparency and trustworthiness. Critical issues such as bias, fairness, and privacy are increasingly coming under scrutiny, both in public discourse and academic research. In response, there's a growing momentum in developing recommender systems that are not only efficient but also uphold these ethical standards.
Resolving contradictions in biomedical information extraction (20 November, 2023)
Speaker: Jake Lever
Abstract
Building a knowledge graph is an important step for representing biomedical knowledge and making interesting inferences such as new uses for existing drugs. However, these graphs can often contain apparent contradictions. This conflicting information (e.g. that a drug both treats and causes a disease) can confuse machine learning analysis and inferences. This work looks to understand what was causing these in a text-mining derived knowledge graph and how NLP could be used to resolve them.
Bio
Jake is a lecturer in the School of Computing Science with a focus on biomedical text mining. He did his postdoctoral research at Stanford University and completed his Ph.D. at the University of British Columbia in Vancouver, Canada.
Exploring Medical Image Segmentation with Fully Convolutional Vision Transformers (17 November, 2023)
Speaker: Dr Chaitanya Kaul
Vision Transformers have been applied to various domains of computer vision applications. Challenges posed by the fine-grained nature of medical image analysis mean that the adaptation of the transformer for their analysis is still at nascent stages. The overwhelming success of the encoder decoder architecture like UNet, lay in its ability to appreciate the fine-grained nature of the segmentation task, an ability which most existing transformer based models do not currently posses. In this talk, I will go through our recent works [1] [2] [3] to address this shortcoming of transformer models for medical image segmentation tasks showing how inductive bias towards images can be introduced to transformers to learn long range semantic dependencies inside them, and how such feature dependencies can be processed for effective, faster, segmentation of CT, MRI and RGB modalities.
References
[1] Tragakis, A., Kaul, C., Murray-Smith, R. and Husmeier, D., 2023. The fully convolutional transformer for medical image segmentation. In Proceedings of the IEEE/CVF Winter Conference on Applications of Computer Vision (pp. 3660-3669).
[2] Liu, Q., Kaul, C., Wang, J., Anagnostopoulos, C., Murray-Smith, R. and Deligianni, F., 2023, June. Optimizing Vision Transformers for Medical Image Segmentation. In ICASSP 2023-2023 IEEE International Conference on Acoustics, Speech and Signal Processing (ICASSP) (pp. 1-5). IEEE.
[3] GLFNet: Global-Local (Frequency) Filter Networks for efficient Medical Image Segmentation. (Under Review, ISBI 2024)
Speaker: Dr. Chaitanya Kaul is a Research Associate in the Inference Dynamics and Interaction Group, at School of Computing Science, University of Glasgow, working under Prof. Roderick Murray-Smith. He is currently funded by Google, and QuantIC, working on 3D Computational Imaging problems where he investigates how unconvential imaging sensors like radars and SPADs can be used for 3D scene understanding and 3D scene interaction. He was previously funded by iCAIRD where he investigated adversarial testing of machine learning algorithms to understand feature leakage in medical imaging applications. His research interests are in Computational Imaging, Medical Image Segmentation and 3D Shape Analysis.
CVAS Seminar: DiffInfinite: Large Mask-Image Synthesis via Parallel Random Patch Diffusion in Histopathology (17 November, 2023)
Speaker: Marco Aversa
In this talk, I will present DiffInfinite, a hierarchical diffusion model that generates arbitrarily large histological images while preserving long-range correlation structural information. This approach first generates synthetic segmentation masks, subsequently used as conditions for the high-fidelity generative diffusion process. The proposed sampling method can be scaled up to any desired image size while only requiring small patches for fast training. Moreover, it can be parallelized more efficiently than previous large-content generation methods while avoiding tiling artifacts. The training leverages classifier-free guidance to augment a small, sparsely annotated dataset with unlabelled data. The method alleviates unique challenges in histopathological imaging practice: large-scale information, costly manual annotation, and protective data handling. The biological plausibility of DiffInfinite data is evaluated in a survey by ten experienced pathologists and a downstream classification and segmentation task. Samples from the model score strongly on anti-copying metrics which is relevant for the protection of patient data.
Information Extraction with (Multimodal) LLMs (13 November, 2023)
Speaker: Zaiqiao Meng
Abstract
Information extraction from diverse sources, such as scanned documents and biomedical texts, is a crucial and challenging task in various domains. In this talk, we will introduce some advanced methods for information extraction across varied domains. We will first introduce GenKIE, a multimodal generative model for extracting key details from scanned documents, adept at handling OCR errors without needing granular annotations. Shifting to the biomedical domain, we will present the Synonym Generalization (SynGen) framework, designed for the task of biomedical named entity recognition. This method tackles named entity recognition challenges by effectively addressing synonym generalization issues inherent in dictionary-based models. Together, these approaches showcase the forefront of information extraction using generative, discriminative, dictionary-based and their combined techniques.
Bio
Zaiqiao is a Lecturer of the University of Glasgow, based within the world-leading Information Retrieval Group and IDA section of School of Computing Science. He was previously working as a Postdoctoral Researcher at the Language Technology Laboratory of the University of Cambridge, and at the Terrier team of the University of Glasgow, respectively. Zaiqiao obtained his Ph.D. in computer science from Sun Yat-sen University in December 2018. His research interests include information retrieval, graph neural networks, knowledge graphs and NLP, with a current focus on the biomedical domain.
DiffInfinite: Large Mask-Image Synthesis via Parallel Random Patch Diffusion in Histopathology (10 November, 2023)
Speaker: Marco Aversa
We present DiffInfinite, a hierarchical diffusion model that generates arbitrarily large histological images while preserving long-range correlation structural information. Our approach first generates synthetic segmentation masks, subsequently used as conditions for the high-fidelity generative diffusion process. The proposed sampling method can be scaled up to any desired image size while only requiring small patches for fast training. Moreover, it can be parallelized more efficiently than previous large-content generation methods while avoiding tiling artifacts. The training leverages classifier-free guidance to augment a small, sparsely annotated dataset with unlabelled data. Our method alleviates unique challenges in histopathological imaging practice: large-scale information, costly manual annotation, and protective data handling. The biological plausibility of DiffInfinite data is evaluated in a survey by ten experienced pathologists as well as a downstream classification and segmentation task. Samples from the model score strongly on anti-copying metrics which is relevant for the protection of patient data.
Undesired Effects and Popularity Bias In Recommendation (06 November, 2023)
Speaker: Anastasia Klimashevskaia
Undesired Effects and Popularity Bias In Recommendation
Abstract
The rapid growth of the volume and variety of online media content has made it increasingly challenging for users to discover fresh content that meets their particular needs and tastes. Recommender Systems are digital tools that support users in navigating the plethora of available items. While these systems may offer several benefits, they may also create or reinforce certain undesired effects, including Popularity Bias, i.e., the tendency of a recommender system to excessively utilize the effect of recommending popular items to the user. In my research I focus on understanding the bias and the ways to mitigate it. In this talk I will explain about calibrated recommendation techniques and how it can be applied to a recommender system to promote diversity and novelty of recommendation.
Bio
Anastasia Klimashevskaia is a PhD student at the University of Bergen. Born in 1995 in Orel, Russia, she obtained a bachelor degree at Moscow State University for the Humanities with the main focus on Computational Linguistics, Natural Language Processing and Robotics. To broaden the horizons, Anastasia has moved to Graz, Austria and has completed a master program there at Graz University of Technology. Her master thesis was addressing the problem of creating automated summarisation system for American legislation committees' transcripts, in an attempt to create a news source utilizing the available legislation data and cater the facts in an accessible way to a wider audience minimising any bias in the new articles generated. This thesis was conducted in collaboration with California Polytechnic State University within a half-a-year research work in San Luis Obispo, California. Excited about solving such complicated tasks and make technology more user-friendly, fair and responsible, Anastasiia has decided to pursue further career in research and has been accepted to the University of Bergen and MediaFutures to a PhD position researching Recommender Systems. Apart from research, she is also passionate about painting and drawing, hiking, cooking and gaming.
From Brain Waves to Pixels: EEG-Driven GANs for Semantic Image Editing and Visual Cognition (03 November, 2023)
Speaker: Carlos de la Torre-Ortiz
Carlos de la Torre is a 3rd-year PhD student at the University of Helsinki focusing on brain-computer interfacing who is visiting our group for two months (mid-October to mid-December).
His talk will explore the applications of brain-computer interfaces (BCIs) and generative adversarial networks (GANs) in the domains of semantic image editing and visual cognition research. First, he will introduce a novel approach that employs electroencephalography (EEG) as implicit feedback for training GANs in semantic feature representation. Second, he will show how to use EEG-based feedback to guide the latent representation within GANs, enabling nuanced image editing. Lastly, he will investigate the relationship between EEG and image perception, quantifying the distance between a perceived and a target image in the GAN's latent space. He will conclude by arguing that this graded response mechanism sets the stage for future BCI research that moves beyond binary classifications (e.g., P3 spellers) to leverage graded relevance based on proximity to a target.
Towards Novel Query Performance Predictors: Bridging the Gap Between How We Retrieve Information and How We Predict Performance. (30 October, 2023)
Speaker: Guglielmo Faggioli
Bio:
Guglielmo Faggioli is a Post-Doc researcher at the University of Padua (UNIPD), Italy. He is Lecturer in Privacy Preserving Information Access. His main research interests regard Information Retrieval focusing on evaluation, performance modelling, query performance prediction, conversational search systems, and privacy-preserving IR.
Early Detection of Misinformation & Disinformation on Social Media (26 October, 2023)
Speaker: Prasenjit Mitra
Enhancing Conversational Techniques: the role of Synthetic Dialogue Generation (23 October, 2023)
Speaker: Xi Wang
Breaking Boundaries of Human-in-the-Loop Design Optimization (20 October, 2023)
Speaker: Yi-Chi Liao
Human-in-the-loop optimization (HILO) has emerged as a principled solution for design optimization, utilizing computational optimization to intelligently select designs for user testing. While HILO has demonstrated success within the human-computer interaction (HCI) domain, its application has faced various constraints. This talk explores computational augmentations that push the boundaries of HILO, enabling its deployment in diverse and realistic design tasks. The talk explores several enhancements for HILO, addressing its limitations and expanding its scope; it includes extensions of HILO to multi-objective design tasks, population-level optimization within HILO, and the application of HILO in designing physical interfaces. Additionally, the talk investigates the future potential of HILO, empowered by advanced user models and simulations. Overall, this talk aims to showcase HILO's progress, its capacity to tackle real-world design problems, and its role in shaping the future of design optimization.
Weekly CVAS seminar (20 October, 2023)
Speaker: George Killick
This week George Killick will talk about collaborating on research papers with other PhD students.
Abstract:
I will give an overview of my experience and what I learned as a second author on three research papers from Zijun Long, Richard McCreaddie, Gerardo Aragon-Camarasa, and Ziaqiao Meng. Finally, I will discuss the benefits of collaborating with other students and some advice for doing this successfully.
Gender Fairness in Information Retrieval Systems (16 October, 2023)
Speaker: Negar Arabzadeh
Title:
Gender Fairness in Information Retrieval Systems
Abstract:
Recent studies have shown that it is possible for stereotypical gender biases to find their way into representational and algorithmic aspects of retrieval methods; hence, exhibit themselves in retrieval outcomes. In this talk, we go over studies that have systematically reported the presence of stereotypical gender biases in Information Retrieval systems. We further classify existing work on gender biases in IR systems as being related to (1) relevance judgement datasets, (2) structure of retrieval methods, and (3) representations learnt for queries and documents. We present how each of these components can be impacted by or cause intensified biases during retrieval. Additionally, the evaluation metrics that can be used for measuring the level of bias and utility of the models, and de-biasing methods that can be leveraged to mitigate gender biases within those models would be covered to some extent.
Bio:
Negar is a PhD student at the University of Waterloo, supervised by Dr. Charles Clarke. She has been conducting research in Information Retrieval and Natural Language Processing for over 5 years as a graduate student and research assistant at Toronto Metropolitan University and the University of Waterloo. Her research interests are aligned with Ad-hoc Retrieval and fairness evaluation IR and NLP. Specifically, she presented tutorials on fairness and evaluation in information retrieval in SIGIR 2022, WSDM 2022, and ECIR 2023. Negar has also completed research-oriented internships at Microsoft Research, Spotify Research, and Google Brain. Additionally, she was one of the lead organizers of the NeurIPS IGLU competition on Interactive Grounded Language Understanding in a Collaborative Environment.
CVAS Reading Session-- 3D vision (13 October, 2023)
Speaker: Zhuo He and CVAS members
This week we will embark on an exciting exploration of 3D vision in our second reading session! Big thanks to Zhuo He for picking a key paper for us to discuss:
“3D vision is a long-standing problem and huge topic in computer vision, as the recent popularity of a combination of deep learning and computer graphics, people can model all needed information from the whole 3D scene, inspiring many downstream tasks including rendering, object detection, 3D reconstruction, etc. Due to the fast movement of this research area, it's difficult to cover all importance within an hour, so I plan to start from some fundamental points about problem setting, the connection between rendering and deep learning, current existing issues, etc. I've chosen the paper "GRAF: Generative Radiance Fields for 3D-Aware Image Synthesis" () for sharing some of my own insights, and welcome to discuss them together.”
Please try to skim or read these before Friday so we can chat about them. Looking forward to a stimulating session and exchange of ideas. We are meeting in SAWB 423 this week at 1 p.m.
An overview of 3 years of SPLADE (09 October, 2023)
Speaker: Carlos Lassance
Abstract:
Simulating Interaction Movements via Optimal Feedback Control and Deep Reinforcement Learning (06 October, 2023)
Speaker: Markus Klar
Extensive user studies are required for the development of interaction techniques, which can be both time-consuming and expensive. To keep pace with the growing market for VR/AR applications, the ability to predict user behaviour using in silico methods and apply this knowledge during the development process is crucial.
We formulate the interaction of humans with computers as an Optimal Control Problem and explore, how different Optimal Feedback Control (OFC) methods can predict user behaviour. In particular, we combine Model Predictive Control with a state-of-the-art biomechanical model, implemented in the fast physics engine MuJoCo. Comparing to real users performing mid-air pointing movements, our approach can produce end-effector trajectories as well as joint movements that are within the between-user variance.
In addition, we train agents to solve different interaction tasks, e.g., tracking or choice reaction, using Deep Reinforcement Learning (DRL). Unlike most OFC methods, DRL approaches can cope well with larger control/state spaces and therefore allow the integration of direct muscle control as well as visual and proprioceptive perception. The resulting simulations can help designers of interaction techniques to learn about possible impacts of design choices and to optimise interfaces in terms of ergonomics or efficiency.
In the future, it is possible that real-time predictions may enhance both the speed and precision of interactions, ultimately leading to seamless interactions with the virtual world.
CVAS Reading Session-- Generative AI (06 October, 2023)
Speaker: Daniela and CVAS members
This week we will embark on an exciting exploration of Generative AI in our very first reading session! Bigs thanks to Deniela for picking two key papers for us to discuss:
"Generative AI is a hugh topic and it's impossible to cover everything in one hour, so since this is the first paper reading session on the topic, i thought we should start with what i think are the most influential papers on text and image generation respectively. The papers I've chosen are "Language Models are Few-Shot Learners" (https://arxiv.org/abs/2112.10752) which is the paper that proposes Stable Diffusion. These papers are a great starting point for exploring the current wave of Generative AI models! "
Please try to skim or read these before Friday so we can chat about them. Looking forward to a stimulating session and exchange of ideas. We are meeting in SAWB 423 this week at 1 p.m.
What's my next investment? Automated recommendations for investors (05 October, 2023)
Speaker: Richard McCreadie & Javier Sanz-Cruzado Puig
As the amount of financial assets and information about them in the market increases, it becomes more challenging for investors and financial advisors to select relevant assets to add to financial portfolios. Financial asset recommendations alleviate this information overload by leveraging AI methods to identify a reduced set of assets of interest to the investor. In this event, researchers from the University of Glasgow will discuss how these technologies work, their current challenges and display and demonstrate recent advances in financial technologies.
This event is part of the Scottish Fintech Festival.
Search, Recommendation, and Sea Monsters (02 October, 2023)
Speaker: Michael D. Ekstrand
Title:
Search, Recommendation, and Sea Monsters
Abstract:
Ensuring that information access systems are “fair”, or that their benefits are
equitably experienced by everyone they affect, is a complex, multi-faceted
problem. Significant progress has been made in recent years on identifying and
measuring important forms of unfair recommendation and retrieval, but there are
still many ways that information systems can replicate, exacerbate, or mitigate
potentially discriminatory harms that need careful study. These harms can
affect different stakeholders — such as the producers and consumers of
information, among others — in many different ways, including denying them
access to the system's benefits, misrepresenting them, or reinforcing unhelpful
stereotypes.
In this talk, I will provide an overview of the landscape of fairness and
anti-discrimination in information access systems and their underlying theories,
discussing both the state of the art in measuring relatively well-understood
harms and new directions and open problems in defining and measuring fairness
problems.
Bio:
Michael Ekstrand is an assistant professor of information science at Drexel
University. His research blends information retrieval, human-computer
interaction, machine learning, and algorithmic fairness to try to make
information access systems, such as recommender systems and search engines, good
for everyone they affect. In 2018, he received the NSF CAREER award to study how
recommender systems respond to biases in input data and experimental protocols
and predict their future response under various technical and sociological
conditions.
Previously he was faculty at Boise State University, where he co-led the People
and Information Research Team, and earned his Ph.D in 2014 from the University of
Minnesota. He leads the LensKit open-source software project for enabling
high-velocity reproducible research in recommender systems and co-created the
Recommender Systems specialization on Coursera with Joseph A. Konstan from the
University of Minnesota. He is currently working to develop and support
communities studying fairness and accountability, both within information access
through the FATREC and FACTS-IR workshops and the Fair Ranking track at TREC,
and more broadly through the ACM FAccT community in various roles.
Re-Thinking Re-Ranking (25 September, 2023)
Speaker: Sean MacAvaney
Title:
Re-Thinking Re-Ranking
Abstract:
Re-ranking systems take a "cascading" approach, wherein an initial candidate pool of documents are ranked and filtered to produce a final result list. This approach exhibits a fundamental relevance misalignment problem: the most relevant documents may be filtered out by a prior stage as insufficiently relevant, ultimately reducing recall and limiting the potential effectiveness. In this talk, I challenge the cascading paradigm by proposing methods that efficiently pull in additional potentially-relevant documents during the re-ranking process, using the long-standing Cluster Hypothesis. I demonstrate that these methods can improve the efficiency and effectiveness of both bi-encoder and cross-encoder retrieval models at various operational points. Cascading is dead, long live re-ranking!
Bio:
Sean is a Lecturer in Machine Learning at the University of Glasgow and a member of the Terrier Team. His research primarily focuses on effective and efficient neural retrieval. He completed his PhD at Georgetown University in 2021, where he was a member of the IR Lab and an ARCS Endowed Scholar. He was a co-recipient of the SIGIR 2023 Best Paper Award and the ECIR 2023 Best Short Paper Award.
Very Deep VAEs -- a review and retrospective in the post-LLM era (14 September, 2023)
Speaker: Rewon Child,
Abstract:
In this talk, I will discuss very deep variational autoencoders. These models can be shown to include the family of autoregressive models, but also more efficient generative models. I'll review some experiments showing that these models can, in fact, learn better generative models in the domain of images, and tie this in to related work about diffusion models. Then I will reflect on the significance of these findings in the era of LLMs.
Bio:
Rewon Child is a member of the founding team and research scientist at Inflection AI. Previously, he worked at OpenAI on the Sparse Transformer architecture and contributed to work showing the emergent capabilities of large language models (GPT-2, GPT-3, Image GPT, and more). He also worked at Google Brain on PaLM. His area of research is unsupervised learning that scales,
Searching Large Collections of Papers (07 September, 2023)
Speaker: Douglas W. Oard
Abstract:
Information retrieval has for decades focused on finding digital documents, including documents that were born digital and documents that have been digitized. But there are also enormous collections of physical documents, on paper or microfilm, for example, that are not likely to be fully digitized in our lifetimes. For example, The U.S. National Archives and Records Administration (NARA) presently holds 11.7 billion pages, only about 2% of which is presently either in digital or digitized form. The National Archives (TNA) in the U.K. is a bit further along on this, with about 5% of its 13.3 billion pages presently in digital or digitized form. But these are just two among literally of thousands of repositories; there are more than 25,000 archival repositories in the United States alone. Access to the culturally important materials that these repositories curate is presently mediated largely through high-level descriptions of entire collections that have been written by archivists, along with detailed descriptions of how some of those collections are organized. In this talk, I will describe a project in which we seek to build on that descriptive work, both by leveraging the limited amount of digitization that has been performed and by assembling descriptions of archival content from published materials such as journal articles or books. I’ll describe two sets of experiments. In the first, for U.S. State Department documents stored in 35 boxes at NARA we asked whether we could guess which box to look in to satisfy a query based on digitizing just a few documents from each box. In the second, we asked whether we could find citations to archival materials in scholarly literature. I’ll use the results of these experiments to motivate a broader research program in which we seek to model the content of unseen documents based on multiple sources of evidence about other documents in the same collection, and in which we seek to enrich that evidence by helping scholars who are working in archives to expand what we know about the contents of those repositories. This is joint work with Tokinori Suzuki, Emi Ishita and Yoichi Tomiura at Kyushu University (Japan), David Doermann at the University at Buffalo (USA), and Katrina Fenlon and Diana Marsh at the University of Maryland (USA).
Bio:
Douglas W. Oard is a Professor at the University of Maryland, College Park (USA), with joint appointments in the College of Information Studies (the iSchool) and the University of Maryland Institute for Advanced Computer Studies (UMIACS). With a Ph.D. in Electrical Engineering, his research interests center around the use of emerging technologies to support information seeking. He is perhaps best known for his work on cross-language information retrieval.
Applying optical imaging techniques to develop new Space Situational Awareness capabilities (15 August, 2023)
Speaker: Dr George Brydon
Abstract:
Spaceborne imaging has been used for over seven decades for documenting space missions, conducting scientific observations of planetary bodies, monitoring spacecraft performance, and providing input to spacecraft navigation systems. Recently, the growing risk posed by space debris in Low Earth Orbit (LEO) has led to significant interest in a new use for spaceborne optical sensors: detecting, tracking and characterising space debris objects. Whilst these observations (knows as Space Situational Awareness, SSA) are traditionally performed from the ground, conducting them from space offers opportunities for entirely new and important capabilities, and will benefit from techniques spanning sensing hardware, computer vision, AI, 3D vision and low power computing. This talk will give an overview of SSA, the motivation for conducting it from space, the sensors involved, and the challenges/opportunities it presents for research and development of new sensing techniques.
Short Bio:
George is a research fellow in the Imaging Concepts group of the school of Physics and Astronomy, working on developing new approaches to spaceborne imaging. He previously worked as a space situational awareness engineer at Astroscale, leading the development of hardware and techniques for spaceborne imaging of space debris. Prior to that he was a member of the EnVisS camera hardware team for the European Space Agency’s Comet Interceptor mission. George completed his PhD in spaceborne imaging techniques at University College London’s Mullard Space Science Laboratory.
Query Automation for Systematic Reviews (10 July, 2023)
Speaker: Harry Scells
Abstract
Medical systematic reviews are at the heart of clinical practice and institutional policy-making, constituting the gold standard in evidence. Systematic reviews are created by searching for and synthesising literature such as randomised controlled trials for highly focused research questions. Given the comprehensiveness requirement for systematic reviews, they naturally are expensive and time-consuming: costing upwards of 250.000EUR and often taking longer than two years to complete. The screening process is arguably the most expensive and time-consuming aspect of systematic review creation, where all studies retrieved by a Boolean query are assessed for possible inclusion in the review.
While most information retrieval research in this space focuses on ranking the studies retrieved by the Boolean query, I instead take the approach that improving the queries themselves will yield far greater savings in cost and time. That is, queries that retrieve fewer documents require fewer documents to screen. This research direction is the main idea behind query automation: methods that seek to assist humans partially or fully in making their queries better at retrieving documents. In this talk, I will present several recent research developments that have tackled the problem of query automation for systematic review literature search.
Bio
Harry Scells is an Alexander von Humboldt Research Fellow at Leipzig University, Germany. His primary research interest is developing information retrieval methods that make retrieving documents with complex (e.g., Boolean) queries more effective. He received his PhD in 2021 from The University of Queensland, Australia under the joint supervision of Prof. Dr Guido Zuccon and Associate Prof. Dr Bevan Koopman.
Pessimistic Decision-Making for Recommender Systems (03 July, 2023)
Speaker: Olivier Jeunen
Abstract
The “bandit learning” paradigm is an attractive choice to recommendation practitioners, because it allows us to optimise a model directly for the outcomes driven by our recommendations, embracing the “sequential decision-making” view of the problem. Practical systems often adopt the “off-policy” learning paradigm, where we log the decisions and outcomes the deployed system has made — and use it to learn new and improved recommendation policies. Because of the selection bias that is induced by the so-called “logging policy”, it becomes very likely that we over-estimate the reward a context-action-pair will yield: a problem known as “The Optimiser’s Curse”.
Pessimistic decision-making policies have been proposed in the literature to deal with these issues, and have proven effective in reducing over-confidence and improving recommendation quality; leading to progress in general Reinforcement Learning scenarios as well. The talk will provide an overview of this general idea, drawing connections between instantiations of pessimism in policy- and value-based learning, and showing how pessimistic decision-making can yield empirical improvements.
Bio
Olivier Jeunen is a Lead Decision Scientist at ShareChat with a PhD from the University of Antwerp, who has previously held positions at Amazon, Spotify, Facebook and Criteo. His research focuses on applying ideas from causal and counterfactual inference to recommendation and advertising problems, which have led to 20+ peer reviewed contributions (i.a. NeurIPS, KDD, RecSys, ToRS), and two best paper awards. He is an active Program Committee member for KDD, RecSys, The WebConf, CIKM, WSDM and SIGIR, whilst reviewing for several journals and workshops—which has led to two outstanding reviewer awards. Olivier was an organising committee member at DIR ’20, CONSEQUENCES ’22-’23, RecSys ’22–’23 and will co-chair the Industry Day at ECIR ’24.
Honorary Degree Talk: Using Coopetition to Foster Research (27 June, 2023)
Speaker: Ellen M Voorhees
Coopetitions are activities in which competitors cooperate for a common good. The shared evaluation tasks that have proliferated in many artificial intelligence subfields are prototypical examples of coopetitions, and they drive the research in their respective areas. The Text REtrieval Conference (TREC, trec.nist.gov) is a long-running community evaluation that has been part of the information retrieval field for more than thirty years. This longevity suggests that, indeed, the net impact of community evaluations is positive.
This talk will describe how TREC supports the information retrieval community. Coopetitions can improve state-of-the-art effectiveness by establishing a research cohort and constructing the infrastructure necessary to make progress on the task. They can also facilitate technology transfer and amortize the infrastructure costs. The primary danger of coopetitions is for an entire research community to overfit to some peculiarity of the evaluation task. This risk can be minimized by building multiple test sets and regularly updating the evaluation tasks.
Biography: Ellen Voorhees is a Fellow at the US National Institute of Standards and Technology (NIST). Ellen has made considerable contributions and advances in information retrieval, such as query expansion, and clustering but particularly in relation to the evaluation of information retrieval system. Ellen was elected in 2018 as an ACM Fellow for "contributions in evaluation of information retrieval, question answering, and other language technologies". Ellen is best known as the programme manager of the Text REtrieval Conference (TREC) project, an international project initiated in 1992, which funds the infrastructure required for the large-scale evaluation. Ellen is an inaugural member of the ACM SIGIR Academy and has been awarded the U.S. Department of Commerce Gold Medal Award, 2021.
NB: This (in-person) talk will be followed by a drinks reception
Multi-Objective Recommender Systems (26 June, 2023)
Speaker: Dietmar Jannach
Abstract:
It is well known from the literature that optimizing recommendations for a single objective, e.g., prediction accuracy, may be too limiting in certain applications. Instead, it is often important not only to consider multiple quality factors of recommendations, e.g., diversity, but to also take the perspectives of multiple stakeholders into account. In this talk, we will review different approaches from the literature that aim to consider multiple objectives in the recommendation process. Furthermore, we will outline open challenges and future directions in this area.
Bio:
Dietmar Jannach is a professor of computer science at the University of Klagenfurt, Austria. His main research theme is related to the application of intelligent system technology to practical problems and the development of methods for building knowledge-intensive software applications. In recent years, he worked on various topics in the area of recommender systems. In this area, he also published the first international textbook on the topic.
Metrics for Measuring Normative Diversity in News Recommendations (19 June, 2023)
Speaker: Sanne Vrijenhoek
Abstract:
News recommenders have the potential to fulfill a crucial role in a democratic society, directing news readers towards the information that is most important to them. However, while much attention has been given to optimizing user engagement and enticing users to click, much less research has been done on incorporating editorial values in news recommender systems. I will talk about our interdisciplinary work on defining normative diversity for news recommender systems, challenges for implementation, and the way forward.
Bio
Sanne Vrijenhoek is a PhD candidate at the University of Amsterdam and a member of the AI, Media and Democracy Lab. Her work focuses on translating normative notions of diversity into quantifiable concepts that can be incorporated in news recommender system design.
Count Knowledge: Counting entities on the web (12 June, 2023)
Speaker: Shrestha Ghosh
Abstract:
Count information such as “number of songs by John Lennon” is relevant for many advanced question answering needs. Count information naturally co-exists with instance information (“Let it Be is by John Lennon”). Identifying count information in knowledge bases and text, and giving comprehensive answers to count questions, is an underresearched challenge. We will focus on extractors for count information, and on question answering systems that consolidate count information and ground numbers with explanatory instances.
Bio
Shrestha Ghosh is a PhD student in the Databases and Information Systems group at Max Planck Institute for Informatics in Saarbrücken, Germany. She is supervised by Simon Razniewski and Gerhard Weikum. She obtained her Master's degree in CS from Saarland University. Her interests lie in knowledge harvesting, information retrieval, question answering and web search. She is currently looking into answering count questions on the web.
Computer Vision and Pattern Recognition Challenges in the Energy & Healthcare Sectors (09 June, 2023)
Speaker: Dr Carlos Moreno-Garcia
Abstract:
In this talk, Dr Carlos Moreno-Garcia will share his experiences working in national and international projects related to pattern recognition and computer vision, mostly for the Oil & Gas and Healthcare sectors. He will present the main challenges faced, implemented solutions and possible research directions for the future.
Short bio:
Dr Carlos Moreno-Garcia is a Senior Lecturer and Research Degree Coordinator at RGU. He completed his PhD in 2016 at URV (Tarragona, Spain) and has been recipient of multiple funding streams from different funding bodies such as CONACyT, MINECO, Newton Fund, Data Lab, etc. He has worked with multiple partners including UNAM, EuDIF (EU), DNV GL, Intel and the NHS, amongst others. More info: http://cfmgcomputing.blogspot.com/p/research.html
From netnews to ethics: A Historical Overview of Recommender Systems (05 June, 2023)
Speaker: Alan Said
Abstract:
In this talk I give a historical overview of recommender systems. Starting from the modern definition of recommender system in the early 1990 till today's advanced recommendations methods and applications. The presentation bridges recommender systems to related fields, such as information retrieval, information systems, cognitive science, psychology, and machine learning.
Bio:
Alan Said is Associate Professor at University of Gothenburg. He holds a PhD from Technische Universität Berlin. Prior to joining the University of Gothenburg, Alan held positions in industry and academia. He was lecturer at University of Skövde (2016-2019), machine learning engineer 2014-2016 working on application of state of the art machine learning in a large scale production setting at Recorded Future. He was Senior Researcher (2014) working on recommender systems and evaluation in the Multimedia Computing research group at Delft University of Technology. He was awarded an MSCA Alain Bensoussan ERCIM Fellowship at Centrum Wiskunde & Informatica (2013-2014) for work on evaluation of recommender and personalization systems. Alan's research spans the fields of user modeling, personalization, recommender systems, evaluation, and reproducibility. He has worked in these fields in various national and international projects as researcher, leader, manager, PI, and proposal writer. He has published over 70 scientific works in top journals, conferences, workshops, and books. He has been nominated for and awarded several Best Paper and Poster awards for his research. Alan frequently serves on Program and Organization committees of top venues and journal such as ACM RecSys, WWW, ACM CIKM, ACM UMAP, ACM IUI, UMUAI, TWeb, TKDD
Query Performance Prediction for Conversational Search (22 May, 2023)
Speaker: Chuan Meng
Abstract:
Query performance prediction (QPP) is a core task in information retrieval. The QPP task is to predict the retrieval quality of a search system for a query without relevance judgments. Research has shown the effectiveness and usefulness of QPP for ad-hoc search. Recent years have witnessed considerable progress in conversational search (CS). Effective QPP could help a CS system to decide an appropriate action to be taken at the next turn. Despite its potential, QPP for CS has been little studied. While the task of document retrieval remains the same in ad-hoc search and CS, a user query in CS depends on the conversational history, introducing novel QPP challenges.
This talk will present two papers. The first paper got accepted at SIGIR 2023. In this paper, we take the first step at reproducing and studying the effectiveness of various existing QPP methods in CS. In particular, we seek to explore to what extent findings from QPP methods for ad-hoc search generalise to three CS settings: (i) estimating the retrieval quality of different query rewriting-based retrieval methods, (ii) estimating the retrieval quality of a conversational dense retrieval method, and (iii) estimating the retrieval quality for top ranks vs. deeper-ranked lists. The second paper got accepted at ECIR 2023 Workshop on Query Performance Prediction and Its Evaluation in New Tasks. In this paper, we propose a new QPP method for CS, which incorporates query rewriting quality (measured by perplexity) to improve the effectiveness of QPP methods for CS.
Bio
Chuan Meng is a second-year PhD student at the University of Amsterdam, supervised by Maarten de Rijke and Mohammad Aliannejadi. He is currently working on Conversational Search and Query Performance Prediction. To date, Chuan has published 4 SIGIR long papers, as the first author, reaching over 100 citations (Google Scholar). Moreover, he actively participates in the academic community and serves as a committee member for various conferences including ACL, WSDM, EMNLP, COLING, SIGKDD, AAAI, ICTIR, and ECML/PKDD. For more information, see https://chuanmeng.github.io/
Exit the Needle, Enter the Haystack: Supervised Machine Learning for Aggregate Data (15 May, 2023)
Speaker: Fabrizio Sebastiani
Abstract:
Learning to quantify (a.k.a. “quantification", or "class prior estimation”) is the task of using supervised learning for training “quantifiers”, i.e., estimators of class proportions in unlabelled data. In data science, learning to quantify is a task of its own, related to classification yet different from it, since estimating class proportions by simply classifying all data and counting the labels assigned by the classifier (the “classify and count” method) is known to often return inaccurate class proportion estimates. In this talk I will introduce learning to quantify by discussing applications of learning to quantify, by looking at the reasons why “classify and count” is a suboptimal quantification method, by illustrating some better quantification methods, and by discussing open problems in quantification research.
Bio
Fabrizio Sebastiani is a Director of Research, and leader of the Human Language Technologies group, in the Networked Multimedia Information Access Laboratory at the Institute for the Science and Technologies of Information of the Italian National Council of Research. The group's research interests include text classification, information extraction, quantification, sentiment classification, cross-lingual and cross-domain text classification, technology-assisted review, authorship analysis, and their applications.
Detecting and Countering Untrustworthy Artificial Intelligence (AI) (04 May, 2023)
Speaker: Nikola Banovic
The ability to distinguish trustworthy from untrustworthy Artificial Intelligence (AI) is critical for broader societal adoption of AI. Yet, the existing Explainable AI (XAI) methods attempt to persuade end-users that an AI is trustworthy by justifying its decisions. Here, we first show how untrustworthy AI can misuse such explanations to exaggerate its competence under the guise of transparency to deceive end-users—particularly those who are not savvy computer scientists. Then, we present fndings from the design and evaluation of two alternative XAI mechanism that help end-users form their own explanations about trustworthiness of AI. We use our findings to propose an alternative framing of XAI that helps end-users develop AI literacy they require to critically refect on AI to assess its trustworthiness. We conclude with implications for future AI development and testing, public education and investigative journalism about AI, and end-user advocacy to increase access to AI for a broader audience of end-users.
Additional Key Words and Phrases: Artificial Intelligence (AI); Explainable AI; Trustworthy AI, Responsible AI.
Knowledge-enhanced Task-oriented Dialogue Systems (24 April, 2023)
Speaker: Yue Feng
Abstract
Dialogue systems have achieved substantial progress due to recent success in language model pre-training. One major type of dialogue being studied is task-oriented dialogue (TOD), where the system aims to complete certain tasks. In this talk, Yue will first introduce the main frameworks of TOD systems. Yue will continue by how to utilize structured knowledge and unstructured knowledge to enhance natural language understanding and natural language generation ability of TOD systems. Finally, Yue will close the presentation with some future challenges in TOD.
Bio
Yue Feng is a Ph.D. student in the Department of Computer Science at the University of College London. She got her master degree from the University of Chinese Academy of Sciences, and bachelor degree from the Harbin Institute of Technology. Her research interests lie in information retrieval and natural language processing. She has published several papers in top conferences such as SIGIR, ACL, EMNLP, etc. Moreover, She has served as the PC member for top conferences including SIGIR, CIKM, ACL, EMNLP, etc.
Retrieve, Cluster, Summarize for Generating Relevant Articles (20 April, 2023)
Speaker: Laura Dietz
Abstract
Our goal is to answer complex information needs. We develop algorithms that automatically, and in a query-driven manner, retrieve materials from the Web and compose comprehensive articles that are akin to Wikipedia articles or text books. Especially for information needs, where the user has very little prior knowledge, the web search paradigm of ten blue hyperlinks is not sufficient. Instead, the goal is to recycle Web materials with the help of Knowledge graphs to produce a comprehensive overview. We discuss a retrieve-cluster-generate approach that is trained with coordinated benchmarks. When information is generated, most IR evaluation paradigms fail. We present the EXAM Answerability Metric, as an alternative means of defining relevance that is suitable to compare information access systems that integrate retrieval and generation.
Bio
Laura Dietz is a tenured professor at the University of New Hampshire, where she leads the lab for text retrieval, extraction, machine learning and analytics (TREMA). She presented the tutorial on Neuro-Symbolic Representations for IR, organized the KG4IR workshop and the TREC Complex Answer Retrieval Track. She received an NSF CAREER Award for utilizing fine-grained knowledge annotations in text understanding and retrieval. Previously, she was a research scientist at the Data and Web Science Group at Mannheim University and the Center for Intelligent Information Retrieval (CIIR) at UMass Amherst. She obtained her doctoral degree with a thesis on topic models for networked data from Max Planck Institute for Informatics.
UGRacing Driverless - Autonomous Design and Simulation Development (19 April, 2023)
Speaker: UGRacing team
Abstract: In this talk, the UGRacing Driverless team will deliver their Formula Student presentations, covering the design of their self-driving system and how simulation has been used to assist the development process. Topics covered will include the system’s vision pipeline, a neural network-based approach to the racing line, and the team’s approach to testing and validation. Additionally, they will go in-depth on the progress made on their new simulator and how this has been integrated with the rest of the team.
Bio: UGRacing has been running since 2007 and is the University of Glasgow’s Formula Student team. In 2020 a new sub-team – UGRacing Driverless – was started and began developing the driverless software needed for a car to compete in the Formula Student AI competition. Since then, Driverless has grown to nearly 40 members and is now in the early stages of developing an in-house autonomous driving platform.
Pretraining, Instruction Tuning, Alignment, Specialization: On the Source of Large Language Model Abilities (17 April, 2023)
Speaker: Yao Fu
Abstract
Recently, the field has been greatly impressed and inspired by Large Language Models (LLMs) like GPT-3.5. The LLMs' multi-dimensional abilities are significantly beyond many NLP researchers’ and practitioners’ expectations and thus reshaping the research paradigm of NLP. A natural question is how LLMs get there, and where these fantastic abilities come from. In this talk we try to dissect the strong LLM abilities and trace them to their sources, hoping to give a comprehensive roadmap about the evolution of LLMs.
Bio
Yao Fu is a Ph.D. student at the University of Edinburgh. Previously he finished his M.S. in Columbia University and B.S. in Peking University. Yao studies large scale probabilistic generative models for human language. His research interests include language model evolution, complex reasoning, and how to inject strong abilities to language models from first principles.
Special IR Seminar & Panel Discussion on LLMs in IR (31 March, 2023)
Speaker: Eugene Yang (Johns Hopkins University) and Maik Fröbe (University of Jena)
Mark your calendars! Dr. Eugene Yang and Maik Fröbe will be visiting us for a special IR Seminar. Each will give a talk on a timely topic in the field, and we'll wrap up with a panel discussion on the effect that large language models (like ChatGPT) will have on the field.
14:00-15:00 - Eugene Yang
From Monolingual Neural IR to Cross-Language and to Multi-Language IR
Eugene Yang is a postdoctoral fellow at the Human Language Technology Center of Excellence at Johns Hopkins University. He received his PhD from Georgetown University, where he specialized in High Recall Retrieval. Eugene's current research is focused on cross-language and multilingual retrieval. He is also a co-organizer of the TREC NeuCLIR track since 2022.
15:00-16:00 - Maik Fröbe
TIREx: The Information Retrieval Experiment Platform: Towards Reproducible Shared Tasks in IR
Maik Fröbe is a Ph.D. student under the supervision of Matthias Hagen (University of Halle: 2019 to 2022, University of Jena: 2022 till today) and part of the webis network. Maik received his Bachelor's and Masters's in computer science at the University of Leipzig. His current research interests lie in Information Retrieval, particularly learning to rank, web archive mining, and near-duplicate detection and its impact on IR evaluation. Maik is an active developer of the TIRA.io platform, which improved the reproducibility of a number of shared tasks and has an archive of more than 500 research prototypes.
16:00-16:30
Panel Discussion on large language models in IR
Generative Information Retrieval (20 March, 2023)
Speaker: Donald Metzler
Abstract
Generative language models have proven to be highly effective for a wide range of tasks. There has been growing interest and application of these models to traditional information retrieval problems. In this talk, I will provide an overview of so-called generative information retrieval models. I will then describe two specific applications of such models. The first application focuses on using language models to retrieve/rank documents by directly generating document identifiers from a model. These so-called differentiable search indexes are effective in various settings vs. sparse and dense retrieval models, but have their limitations, which leaves opportunity for future work. The second application is attributed question answering, which takes a question as input and generates a long-form natural language response with citations/attribution back to original sources. A range of different modeling strategies are proposed and evaluated using a novel suite of attribution-focused metrics.
Bio
Donald Metzler is a Senior Staff Research Scientist at Google Research, where he leads a group focused on problems at the intersection of information retrieval, natural language processing, and machine learning. Prior to that, he was a Research Assistant Professor at the University of Southern California (USC) and a Senior Research Scientist at Yahoo!. He has served as the Program Chair of the WSDM, ICTIR, and OAIR conferences and sat on the editorial boards of all the major journals in his field. He has published over 100 research papers, has been awarded 9 patents, and is a co-author of "Search Engines: Information Retrieval in Practice".
One-shot visual language understanding with cross-modal translation and LLMs (13 March, 2023)
Speaker: Fangyu Liu
Abstract
Visual language such as charts and plots is ubiquitous in the human world. Comprehending plots and charts requires strong reasoning skills. Prior state-of-the-art (SOTA) models require at least tens of thousands of training examples and their reasoning capabilities are still much limited, especially on complex human-written queries. We present the first one-shot solution to visual language reasoning. We decompose the challenge of visual language reasoning into two steps: (1) plot-to-text translation, and (2) reasoning over the translated text. The key in this method is a modality conversion module, named as DePlot, which translates the image of a plot or chart to a linearized table. The output of DePlot can then be directly used to prompt a pretrained large language model (LLM), exploiting the few-shot reasoning capabilities of LLMs. To obtain DePlot, we standardize the plot-to-table task by establishing unified task formats and metrics, and train DePlot end-to-end on this task. DePlot can then be used off-the-shelf together with LLMs in a plug-and-play fashion. Compared with a SOTA model finetuned on thousands of data points, DePlot+LLM with just one-shot prompting achieves a 29.4% improvement over finetuned SOTA on human-written queries from the task of chart QA.
Bio
Fangyu Liu is a third-year PhD student in NLP at the University of Cambridge supervised by Professor Nigel Collier and an incoming Research Scientist at Google Research based in Mountain View. His research centres around multi-modal NLP, large language models, self-supervised representation learning and model interpretability. His work has won the Best Long Paper Award at EMNLP 2021. Besides Cambridge, he interned at industrial research labs such as Google Research and Amazon Alexa during his PhD.
Biomedical Relation Extraction Combining External Sources of Knowledge (06 March, 2023)
Speaker: Diana F. Sousa
Abstract
Successful biomedical relation extraction (RE) can provide evidence to researchers about possible unknown associations between entities, advancing our current knowledge about those entities and their inherent processes. Multiple RE approaches have been proposed to identify relations between concepts in literature, namely using neural network algorithms. However, most systems lack external knowledge injection, which is more problematic in the biomedical area given the widespread usage and high quality of biomedical ontologies and other resources. Also, there is a generalized lack of datasets for evaluating biomedical RE approaches due to the high cost of obtaining domain expertise in multiple fields. With this in mind, this seminar has two goals. The first is to present the current work emerging from the field of biomedical RE as well as some recent work in the application of external knowledge. Finally, the second goal is to propose new ways of obtaining biomedical RE gold-standard datasets and discuss their feasibility.
Bio
Diana F. Sousa is a PhD student at LASIGE, Faculty of Sciences, University of Lisbon. Her current research interests focus on information extraction applied to the clinical and biomedical domains.
Towards Sentiment aware Multi-modal Dialogue Systems (27 February, 2023)
Speaker: Tulika Saha
Abstract
Conversational Natural Language Processing (NLP) is a rapidly growing field with applications ranging from virtual assistants for customer service to personalized assistants. The talk will explore dialogue systems in several domains and applications such as ecommerce, AI for Social Good such as mental health, educational NLP etc. In this process, the talk aims to highlight three primary modules of a dialogue system, namely Natural Language Understanding, Dialogue Policy Learning, and Natural Language Generation. As the eventual evaluators of any dialogue systems are users, sentiment of the user plays an important role and provides additional information apart from semantics to ensure a fulfilling conversational experience. Thus, this talk will also explore how sentiment and multi-modality is incorporated in every module of the dialogue systems to learn a richer representation of user needs and queries and to highlight its effect in task-fulfillment.
Bio
Tulika Saha is a Lecturer of Computer Science at the University of Liverpool, United Kingdom (UK). Her current research interests include NLP typically Dialogue Systems, AI for Social Good, Social Media Analysis, Deep Learning and Reinforcement Learning etc. She was a postdoctoral research fellow at the National Centre for Text Mining, University of Manchester, UK. Previously she earned her Ph.D. from the Indian Institute of Technology Patna, India. Her research articles are published in top-tier conferences such as ACL, ACM SIGIR, NAACL etc. and several peer-reviewed journals.
mmSense: AI Assisted Weapon Detection with millimeter wave radars (22 February, 2023)
Speaker: Chaitanya Kaul
Abstract:
Applied weapon detection systems for scene surveillance and security applications tend to be expensive, bulky, and non-privacy preserving. Currently, these systems broadly fall into one of two categories - Imaging based, which are accurate but lack privacy, and RF signal based which are privacy preserving but lack portability. For widespread adoption, public security and surveillance systems must be accurate, portable, compact, and real-time, without impeding the privacy of the individuals being observed. This talk introduces mmSense, our applied signal processing system based on millimetre wave radar technology, capable of detecting the presence of a weapon on a person in a scene, in a discrete, privacy-preserving modality. mmSense currently comprises of two systems - a mains powered highly accurate device that uses sensor fusion to learn correlations between RF signals and TOF images, as well as a highly portable and compact RF signal based USB powered device, both of which achieve high recognition rates on a diverse set of challenging scenes while running on standard laptop hardware, demonstrating a significant advancement towards creating portable, cost-effective real-time radar based surveillance systems.
Bio:
Reinforcement recommendation reasoning through knowledge graphs for explanation path quality (13 February, 2023)
Speaker: Giacomo Balloccu
Abstract
Explaining to users why certain results have been provided to them has become an essential property of modern Recommender Systems (RS). Regulations, such as the European General Data Protection Regulation (GDPR), call for a “right to explanation”, meaning that, under certain conditions, it is mandatory by law to generate awareness for the users on how a model behaves. Additionally, explanations have also been proven to have benefits from a business perspective by increasing trust in the system, helping the users make a decision faster, and persuading a user to try and buy. Existing RSs often act as black boxes, not offering the user any justification for the recommendations. Moreover, when explanations are produced, they are often suboptimal, and they do not consider any property related to user perception. In this talk, we will first briefly introduce the state-of-art for explainable recommendation considering dimensions such as explanation types and model families with particular focus on methods that leverage Knowledge Graphs (KGs). Then we will dive deep into a family of models that are able to provide explanations in form of paths that link previous user behaviour to the recommended item exploiting item relations with different entities. Later, justifying our findings with data, we will showcase a set of properties that measure aspects related to these paths and how these properties can be optimised to increase user perception of the explanations produced. Finally, we will wrap up by showing preliminary results about the reproducibility of these methods and how they compare in terms of a broad set of beyond-accuracy metrics against non-explainable knowledge-aware recommender systems.
Bio
Giacomo Balloccu is a PhD Student at the Department of Mathematics and Computer Science of the University of Cagliari (Italy). His research interests lay in social aspects of recommender systems and their impact on user experience and business perspectives. Currently, his efforts are directed towards knowledge-based and explainable recommender systems. In his last works, he focused on defining measures for properties of explanations and methods to optimise them while maintaining high recommendation quality. His works appeared in the proceedings of conferences such as ACM SIGIR 2022 and journals such as Elsevier Knowledge-Based Systems. He co-authored the tutorial "Hands-on Explainable Recommender Systems with Knowledge Graphs" at ACM RecSys 2022. He is also Teaching Assistant of "Algorithms and Data Structure" at the University of Cagliari and currently interning as Applied Scientist at Amazon.
Music Information Retrieval and its Application in Music Recommender Systems (06 February, 2023)
Speaker: Lorenzo Porcaro
Abstract
Music Information Retrieval (MIR) is an interdisciplinary field concerned with the extraction of information from music and its analysis. Starting to be acknowledged as a scientific field at the beginning of this century, today many of its applications have been transformed in world widespread technologies: from recommender systems helping us in finding music in streaming services, to automatic genre and mood recognition systems that generate tailored music playlists. In this talk, I will start by presenting the history and evolution of MIR as a field, then focusing on one of its most successful applications, music recommender systems.
Bio
Lorenzo Porcaro is a research scientist at the European Center of Algorithmic transparency (ECAT), part of the European Commission's Joint Research Centre. His work focus on assessing the impact that recommender systems have on their users, with a focus on human rights violation and discrimination. He holds a PhD in Information and Communication Technology, and Master's degrees in Sound and Music Computing (M.Sc.) and Intelligent Interactive Systems (M.Sc.) from Universitat Pompeu Fabra (Spain). His research interests include recommender systems, social computing, human-computer interaction, and music information retrieval.
The Fully Convolutional Transformer in Medical Imaging (01 February, 2023)
Speaker: Thanos Tragakis
Abstract:
In this talk I will discuss our WACV 2023 paper called The Fully Convolutional Transformer in Medical Imaging. I will talk about the current literature on creating transformers for medical image segmentation, moving on to our FCT model and why our model works better.
We propose a novel transformer model, capable of segmenting medical images of varying modalities. Challenges posed by the fine-grained nature of medical image analysis mean that the adaptation of the transformer for their analysis is still at nascent stages. The overwhelming success of the UNet lay in its ability to appreciate the fine-grained nature of the segmentation task, an ability which existing transformer based models do not currently posses. To address this shortcoming, we propose The Fully Convolutional Transformer (FCT), which builds on the proven ability of Convolutional Neural Networks to learn effective image representations, and combines them with the ability of Transformers to effectively capture long-term dependencies in its inputs. The FCT is the first fully convolutional Transformer model in medical imaging literature. It processes its input in two stages, where first, it learns to extract long range semantic dependencies from the input image, and then learns to capture hierarchical global attributes from the features. FCT is compact, accurate and robust. Our results show that it outperforms all existing transformer architectures by large margins across multiple medical image segmentation datasets of varying data modalities without the need for any pre-training. FCT outperforms its immediate competitor on the ACDC dataset by 1.3%, on the Synapse dataset by 4.4%, on the Spleen dataset by 1.2% and on ISIC 2017 dataset by 1.1% on the dice metric, with up to five times fewer parameters. On the ACDC Post-2017-MICCAI-Challenge online test set, our model sets a new state-of-the-art on unseen MRI test cases outperforming large ensemble models as well as nnUNet with considerably fewer parameters.
Bio:
Thanos earned his Master’s degree in Data Analytics from the University of Glasgow. His Master’s Thesis was in Medical Imaging which ended up being a published paper. Now he is a first year PhD student supervised by Dr. Faccio and Dr. Kaul developing AI solutions for computational imaging tasks. Specifically, the AI focuses on both classical and quantum technologies involving single photon cameras, time of flight cameras and also mmWave sensors alongside standard RGB cameras. Additionally, there is a focus on data fusion across multiple sensors in order to develop better imaging technologies for 3D imaging, face recognition, surveillance and bio-imaging.
CVAS Weekly Meeting (25 January, 2023)
Speaker: Richard Menzies
Abstract
Evaluation and privacy in recommender systems (23 January, 2023)
Speaker: Walid Krichene
Abstract:
Design Engineering for AI Engineering (19 January, 2023)
Speaker: Per Ola Kristensson
In this talk I will give an overview of some of our recent work on designing AI-infused interactive systems for a variety of applications, including efficient communication systems for augmentative and alternative communication and gesture-based systems for virtual and augmented reality. I will then discuss the challenges in AI engineering such and other systems and propose design engineering approaches that can help ensure AI-infused systems are designed to be effective, efficient, and safe.
Query-Specific Entity Representations for Answering Entity-Centric Queries on the Web (16 January, 2023)
Speaker: Shubham Chatterjee
Abstract
Often, many web search queries can be answered using entities, for example, questions such as “Who is the mayor of Berlin?'” or queries that seek a particular list of entities such as “Professional sports team in Philadelphia”. Given a query and a knowledge graph, the entity ranking task is to retrieve entities from the knowledge graph and order them by relevance to the query. Entity ranking has been of considerable interest to the IR community as is evidenced by a plethora of benchmarking campaigns which have addressed the task in some form, the earliest example being the TREC Enterprise track (2005-2008) and the latest being the TREC Product track (2023).
In the present times, neural methods have been demonstrated to be versatile and highly effective for IR tasks. Neural entity-oriented search systems often learn vector representations of entities via the introductory paragraph from the Wikipedia page of the entity. However, such representations are the same for every query (query-agnostic), and hence not ideal for IR tasks. In this talk, I will present my recent work from SIGIR 2021 on learning query-specific entity representations using BERT (BERT-ER). BERT-ER are query-specific vector representations of entities obtained from text that describes how an entity is relevant for a query. Then, I will describe my work from CIKM 2022 that utliizes BERT-ER for a fine-grained semantic annotations task: entity aspect linking.
Bio
Dr. Shubham Chatterjee is a Research Associate working with Dr. Jeff Dalton in the Glasgow Representation and Information Learning (GRILL) Lab in the School of Computing Science at the University of Glasgow. His research is in Information Retrieval, with an emphasis on Neural Entity-Oriented Information Retrieval and Extraction. The goal of his research is to develop novel algorithms that integrate information from text and entities present in the text to help search engines understand the meaning of the text more precisely. The larger goal of his work is to assist in the design of intelligent search systems which would one day respond to a user's open-ended and complex information needs with a complete answer instead of a ranked list of results, thus transforming the "search" engine into an "answering" engine.
Prior to this, he worked as a Postdoctoral Research Fellow with Dr. Laura Dietz at the University of New Hampshire, Durham, USA . This was also where he completed his PhD working with Dr. Dietz.
Research Interests: Entity-Oriented Search, Text Understanding, Neural IR, Conversational IR, Knowledge Graphs for IR, Representation Learning for IR.
System design for the UGRacing driverless project (11 January, 2023)
Speaker: UGRacing
Contrastive Search: The Current State-of-the-art Decoding Method For Neural Text Generation (09 January, 2023)
Speaker: Yixuan Su
Abstract
Neural text generation is indispensable for various NLP applications. Conventional approaches such as greedy search and beam search often leads to problem of model degeneration. On the other hand, stochastic methods like top-k sampling and nucleus sampling often causes the semantic incoherence and top drift in the generated text. In this talk, I wll introduce our newly proposed decoding method --- contrastive search --- which has been accepted to NeurIPS 2022. So far, contrastive search has been extensively validated in 16 languages. In addition, it has been deployed in the industrial projects like Tencent Effidit and has been integrated into the popular transformers library of HuggingFace.
Bio
Yixuan Su is a PhD student in Language Technology Lab at University of Cambridge. He is supervised by Professor Nigel Collier. Previously, he obtained his MPhil degree from University of Cambridge and Bachelor degree from Beijing Institute of Technology. He has broad interest in research areas including large language models, text generation, and representation learning. Up to now, he has published over 10 papers in several top-tier conferences such as NeurIPS, ACL, EMNLP, NAACL, EACL, etc.
From Gambles to User Interfaces: Simulating Decision-Making in the Real World (08 December, 2022)
Speaker: Aini Putkonen
Classical models of decision-making offer valuable insights about people's decision-making tendencies, for example, how they manage risk and uncertainty. This behaviour is often studied in tasks where individuals choose between uncertain outcomes, or gambles. Such tasks are also common when using interactive systems. However, applying models of decision-making in naturalistic settings can be a challenge as they were largely developed in controlled experiments. Experimental settings allow controlling the task design, whereas real-world user interfaces often lack this level of control. In this talk, I hypothesise that considering aspects of the human cognition is key in moving from modelling gambles to similar tasks on real information-rich user interfaces. Such aspects include the visual system, memory and cognitive capacity. I address how to model real-world user behaviour by combining understanding of cognition with reinforcement learning. In particular, theories of human decision-making and psychology are used to process information on displays, producing human-like observations for the learning problem. This problem is then solved using reinforcement learning. The advantages of this approach will be discussed, including construction of simulation models of users for applications like prototyping, recommender systems, and decision support.
Stability within Reinforcement Learning (30 November, 2022)
Speaker: Rory Young
Abstract
Despite recent progress in the field of deep reinforcement learning, classical control theory methods are still often favoured for real world systems due to their theoretical stability guarantees. We aim to address the instability of reinforcement learning controllers by establishing a link between Markov Decision Processes and controllable dynamical systems. Making this association allows us to optimise for stability as quantifying the level of chaos in a system is a well studied issue within the broader context of dynamical systems. In this presentation, we discuss the impact of improving short term and long term stability.
Bio
Rory Young is a second year PhD student focusing on stability within reinforcement learning. Before joining the department, Rory received his bachelor’s in mathematics and computer from the University of Glasgow.
Interplay between Upsampling and Regularization for Provider Fairness in Recommender Systems (28 November, 2022)
Speaker: Ludovico Boratto
Abstract
Considering the impact of recommendations on item providers is one of the duties of multi-sided recommender systems. Item providers are key stakeholders in online platforms, and their earnings and plans are influenced by the exposure their items receive in recommended lists. Prior work showed that certain minority groups of providers, characterized by a common sensitive attribute (e.g., gender or race), are being disproportionately affected by indirect and unintentional discrimination. However, there are situations where (i) the same provider is associated with multiple items of a list suggested to a user, (ii) an item is created by more than one provider jointly, and (iii) predicted user-item relevance scores are biasedly estimated for items of provider groups. In this talk, we assess disparities created by the state-of-the-art recommendation models in relevance, visibility, and exposure, by simulating diverse representations of the minority group in the catalog and the interactions. Based on emerged unfair outcomes, we devise a treatment that combines observation upsampling and loss regularization, while learning user-item relevance scores. Experiments on real-world data demonstrate that our treatment leads to lower disparate relevance. The resulting recommended lists show fairer visibility and exposure, higher minority item coverage, and negligible loss in recommendation utility.
Bio
Ludovico Boratto is a researcher at the Department of Mathematics and Computer Science of the University of Cagliari (Italy). His research interests focus on recommender systems and their impact on the different stakeholders, both considering accuracy and beyond-accuracy evaluation metrics. He has authored more than 60 papers and published his research in top-tier conferences and journals. His research activity also brought him to give talks and tutorials at top-tier conferences and research centers (Yahoo! Research). He is editor of the book “Group Recommender Systems: An Introduction”, published by Springer. He is an editorial board member of the “Information Processing & Management” journal (Elsevier) and “Journal of Intelligent Information Systems” (Springer), and guest editor of several journals’ special issues. He is regularly part of the program committees of the main Web conferences, where he received three outstanding contribution awards. In 2012, he got his Ph.D. at the University of Cagliari (Italy), where he was a research assistant until May 2016. From May 2016 to April 2021, he joined Eurecat as Senior Research Scientist in the Data Science and Big Data Analytics research group. In 2010 and 2014, he spent ten months at Yahoo! Research in Barcelona as a visiting researcher. He is a member of ACM and IEEE.
NeurIPS warm up talks: Bessel Equivariant Networks for Inversion of Transmission Effects in Multi-Mode Optical Fibres and Physical Data Models in Machine Learning Imaging Pipelines (24 November, 2022)
Speaker: Marco Aversa and Josh Mitton
Josh and Marco will give practice talks for their upcoming NeurIPS papers:
J. Mitton, S.P. Mekhail, M. Padgett, D. Faccio, M. Aversa, and R. Murray-Smith, Bessel Equivariant Networks for Inversion of Transmission Effects in Multi-Mode Optical Fibres, NeurIPS 2022. pdf
We develop a new type of model for solving the task of inverting the transmission effects of multi-mode optical fibres through the construction of an SO+(2, 1)- equivariant neural network. This model takes advantage of the of the azimuthal correlations known to exist in fibre speckle patterns and naturally accounts for the difference in spatial arrangement between input and speckle patterns. In addition, we use a second post-processing network to remove circular artifacts, fill gaps, and sharpen the images, which is required due to the nature of optical fibre transmission. This two stage approach allows for the inspection of the predicted images produced by the more robust physically motivated equivariant model, which could be useful in a safety-critical application, or by the output of both models, which produces high quality images. Further, this model can scale to previously unachievable resolutions of imaging with multi-mode optical fibres and is demonstrated on 256 × 256 pixel images. This is a result of improving the trainable parameter requirement from O(N4 ) to O(m), where N is pixel size and m is number of fibre modes. Finally, this model generalises to new images, outside of the set of training data classes, better than previous models.
Aversa, Marco*; Oala, Luis; Clausen, Christoph; Murray-Smith, Roderick; Sanguinetti, Bruno, Physical Data Models in Machine Learning Imaging Pipelines https://ml4physicalsciences.github.io/2022/
Light propagates from the object through the optics up to the sensor to create an image. Once the raw data is collected, it is processed through a complex image signal processing (ISP) pipeline to produce an image compatible with human perception. However, this processing is rarely considered in machine learning modelling because available benchmark data sets are generally not in raw format. This study shows how to embed the forward acquisition process into the machine learning model. We consider the optical system and the ISP separately. Following the acquisition process, we start from a drone and airship image dataset to emulate realistic satellite raw images with on-demand parameters. The end-to-end process is built to resemble the optics and sensor of the satellite setup. These parameters are satellite mirror size, focal length, pixel size and pattern, exposure time and atmospheric haze. After raw data collection, the ISP plays a crucial role in neural network robustness. We jointly optimize a parameterized differentiable image processing pipeline with a neural network model. This can lead to speed up and stabilization of classifier training at a margin of up to 20% in validation accuracy.
Sampling strategies with self-supervised learning for multi-label image classification (16 November, 2022)
Speaker: Ozgu Goksu
Abstract
Learning representations via self-supervised learning is a challenging and significant task in the computer vision community. Thus, numerous self-supervision methods are proposed to learn more robust image representations for any downstream job. However, most self-supervised approaches focus on single-instance data with a single label, and their learning relies on insufficient sampling and inaccurate negative sampling strategies. In this talk, I will focus on the aspect of my PhD which is to define a formal way to describe batch properties that predict better latent representations.
Bio
Ozgu Goksu is a first-year PhD in the department of computing science at the University of Glasgow. She received her bachelor's and master's degrees in the computer science and engineering department at Gebze Technical University. During her master, she worked as a research assistant at the same university, and her projects were related to remote sensing datasets and content-based image retrieval via deep learning. Her PhD research briefly aims at how formal ways can be defined for unsupervised/self-supervised training data curation.
Speaker Email: 2718886g@student.gla.ac.uk
The CVAS seminar happens every week and everyone is welcome to attend. This is an in-person only seminar
Rethink conversational recommendations and beyond (14 November, 2022)
Speaker: Hongning Wang
Abstract
Conversational recommender systems (CRS) dynamically obtain user preferences via multi-turn questions and answers. The existing CRS solutions are widely dominated by reinforcement learning algorithms. In this talk, I will introduce our group’s recent effort that demonstrates a simpler alternative based on decision trees can achieve comparable performance, under the standard attribute-focused CRS evaluation benchmarks. This urges us to consider whether we are making the right assumptions about the users in a recommender system.
If time allows, I would like to also introduce our group’s progress in a parallel direction where we focus on the users’ learning behaviors in a recommender system, i.e., a user also has to update her utility estimation based on observations collected from her consumed recommendations to make improved choices. Our findings suggest the intrinsic difficulty introduced by the user’s learning behavior and the possibility of efficient online learning algorithm design for the system.
Bio
Dr. Hongning Wang is now an Associate Professor in the Department of Computer Science at the University of Virginia. He received his PhD degree in computer science at the University of Illinois at Champaign-Urbana in 2014. His research generally lies in the intersection among machine learning, data mining and information retrieval, with a special focus on sequential decision optimization and computational user modeling. He is a recipient of 2016 National Science Foundation CAREER Award, 2020 Google Faculty Research Award, and SIGIR’2019 Best Paper Award.
Advances in Data Stream Mining with Concept Drift (09 November, 2022)
Speaker: Roberto Souto Maior de Barros
Abstract
In this talk, I provide an introduction to Data Stream Mining and Concept Drift, with a general view of the different approaches that have been tried in the area, a list of contributions made by my research group, with more in depth discussion of some of them, as well as topics of ongoing and future research.
Bio
Roberto Souto Maior de Barros received B.Sc. and M.Sc. degrees in Computer Science from Universidade Federal de Pernambuco (UFPE), Brazil, in 1985 and 1988, respectively, and Ph.D. degree in Computing Science from The University of Glasgow, in 1994. From 1985 to 1995 he worked as systems analyst at UFPE and he is a full time Professor and Researcher, also at UFPE, since 1995. His main research area is machine learning, with special interest in Concept Drift, though he has done work on Formal Methods, Software Engineering, Programming Languages and Databases in the past.
Combining Network Structures and Natural Language Processing for Fake News Detection (07 November, 2022)
Speaker: Gregor Donabauer
Abstract
Recent advances in NLP have led to impressive advances in addressing real-world problems that are highly relevant to society, as for example fake news detection and other forms of toxic content detection. Such problems are usually treated as classification tasks and models that are applied expect a single input type of information e.g., textual information only, which can lead to limitations in representing context around text documents or input entities in general. The reason for that is the lack of knowledge on relations between data of different sources that naturally can be modelled as linked data structures (graphs). Such graphs consist of nodes and edges, where nodes are considered as data points and edges representing interactions between pairs of them. For fake news detection this could for example mean that a post on social media has limited expressiveness if we only look at its text but tells us much more if we consider how it is linked to users interacting with it. Based on the example of fake news detection, this talk discusses how (social) network structures can improve classification tasks in NLP. In addition, an outlook on how such approaches can be projected to completely different domains will be provided.
Bio
Gregor Donabauer is a PhD student and research officer at the chair of Information Science at the University of Regensburg (Germany). In addition to that Gregor also works as a research assistant at the University of Milano-Bicocca (Italy). Before that Gregor completed his Bachelor degree in Information Science/Business Information Systems and his Master degree in Information Science, both at the University of Regensburg. Gregor's main interests are on Natural Language Processing and Machine Learning.
Brain-Machine Interface for Search (31 October, 2022)
Speaker: Yiqun Liu
Abstract
While search engines have reshaped how human beings learn and think, the interaction paradigm of search has remained relatively stable for decades. With the recent progresses in biomedical engineering, it is possible to build a direct communication pathway between a computing device and the human brain via Brain-machine Interfaces (BMIs), which may revolutionize the search paradigm in a predictable future. In this talk, I will discuss the possibility, benefits, and challenges in using BMI as a new interface for search. I will also introduce our recent efforts in constructing a prototype BMI search interface.
Bio
Yiqun Liu is working as professor of the Department of Computer Science and Technology, Director of the Office for Research, as well as Director of the Institute for Internet Judiciary in Tsinghua University. His major research interests are in Web Search, User Behavior Analysis, and Natural Language Processing. He is a distinguished member of ACM and China Computer Federation (CCF). He serves as the chair of the professional committee of information retrieval (CCIR) of Chinese Information Processing Society of China and co-Chair of the steering committee of SIGIR-AP.
Effectiveness and Efficiency Advancements in Conversational Search (24 October, 2022)
Speaker: Nicola Tonellotto
Abstract
In a conversational context, a user converses with a system through a sequence of natural-language questions, i.e., utterances. Starting from a given subject, the conversation evolves through sequences of user utterances and system replies. We aim at improving both the quality (effectiveness) of the replies and the processing time (efficiency) required to search those replies. We address the quality aspect by proposing an adaptive utterance rewriting strategy based on the current utterance and the dialogue evolution of the user with the system. Retrieving relevant documents for a question is difficult due to the informal use of natural language in speech and the complexity of understanding the semantic context coming from previous questions/utterances. In our system, a classifier identifies utterances lacking context information as well as the dependencies on the previous utterances. Our modular architecture performs: (i) automatic utterance understanding and rewriting, (ii) first-stage retrieval of candidate passages for the rewritten utterances, and (iii) neural re-ranking of candidate passages to retrieve the most relevant documents as replies. Rapid responses are fundamental in search applications; it is particularly so in interactive search sessions, such as those encountered in conversational settings. To address the efficiency aspect, we exploit the temporal & spatial locality of conversational queries and propose and evaluate a client-side document embedding cache to improve responsiveness. By leveraging state-of-the-art dense retrieval models to abstract document and query semantics, we cache the embeddings of documents retrieved for a topic introduced in the conversation, as they are likely relevant to successive queries. Our document embedding cache implements an efficient metric index answering nearest-neighbor similarity queries by estimating the approximate result sets returned. It significantly improves the responsiveness of conversational systems while likewise reducing the number of queries managed on the search back-end.
Bio
Prof. Nicola Tonellotto (male) is associate professor at the Information Engineering Department of the University of Pisa since 2019 and honorary research fellow in the College of Science & Engineering of the School of Computing Science of the University of Glasgow since 2020. From 2002 to 2019 he was researcher at the Information Science and Technologies Institute “A. Faedo” of the National Research Council of Italy. His main research interests include Cloud Computing, Web Search, and Information Retrieval, with a particular focus on efficient data processing in distributed computing architectures. He co-authored more than 80 papers on these topics in peer reviewed international journals and conferences. He is co-recipient of the ACM SIGIR 2015 Best Paper Award.
The road from Explainability in Recommender Systems to Visual XAI (17 October, 2022)
Speaker: Denis Parra
Abstract
Transparency and explainability have been studied for more than 20 years in the area of recommender systems (RecSys), due to its impact on the user experience of personalized systems. Only in recent years these topics have reached importance within Artificial Intelligence (AI) as a whole, under the term XAI (eXplainable AI). Some authors have shown that advances in XAI from different fields have not been integrated into a common body of knowledge due to lack of connection among these communities. This talk addresses this issue by showing how works on explainability, transparency, visualization, user interfaces and user control in recommender systems are significantly related to XAI and can inspire new ideas for further research.
Bio
Denis Parra is Associate Professor at the Department of Computer Science, in the School of Engineering at Pontificia Universidad Católica de Chile. He is also principal researcher at the excellence research centers CENIA (National Center for Research in Artificial Intelligence in Chile), iHealth (Millennium Institute for Intelligent Healthcare Engineering), and adjunct researcher at the IMFD (Millennium Institute for Research on Data Fundamentals). He earned a Fulbright scholarship to pursue his PhD studies between 2008-2013 at the University of Pittsburgh.
Prof. Parra research interests are Recommender Systems, Intelligent User Interfaces, Applications of Machine Learning (Healthcare, Creative AI) and Information Visualization.
Prof. Parra has published numerous articles in prestigious journals such as ACM TiiS, ACM CSUR, IJHCS, ESWA, and PloS ONE, as well as in conferences such ACM IUI, ACM RecSys, UMAP, ECIR, Hypertext, and EuroVis among others. Prof. Parra has been awarded a student best paper award at UMAP conference 2011, as well as candidate best paper awards twice at ACM IUI, in 2018 and 2019, for his research on intelligent user interfaces for recommender systems and on AI medical applications.
Retrieval-Enhanced Language Models and Semantic-Driven Summarization for Biomedical Domains (10 October, 2022)
Speaker: Giacomo Frisoni
Abstract
In the last decade, deep learning advancements have boosted the development of many neural solutions for effectively analyzing biomedical literature—widely accessible through repositories such as PubMed, PMC, and ScienceDirect. Large pre-trained language models (PLMs) have become the dominant NLP paradigm, achieving unprecedented results in a panoply of tasks, from named entity recognition and semantic parsing to information retrieval and document summarization. However, the latest batch of research has highlighted several weaknesses of PLMs, including a black-box knowledge limited by weight matrices' dimensions and the scarce ability to separate discrete semantic relations from surface language structures.
This talk presents two papers riding different promising trends to solve these issues and draw a complementary path to architectural scaling: (i) equipping PLMs with the ability to attend over relevant and factual information from non-parametric external sources; (ii) infusing semantic parsing graphs into PLMs.
Specifically, in (i) we will see a T5 model empowered by differentiable access towards a large-scale text memory grounded on PubMed, while in (ii) we will explore a BART model for biomedical abstractive summarization augmented by event and AMR graphs, as well as a semantic-driven reinforcement learning signal.
Bio
Giacomo Frisoni is a second-year Ph.D. student with competencies in Natural Language Understanding and Neuro-Symbolic Learning. He has a Bachelor's and Master's degree in Computer Science and Engineering from the University of Bologna, both with honors. He presented several original papers to journals and international peer-reviewed conferences—including top-tier venues like COLING, winning two Best Paper Awards. He participated in the Cornell, Maryland, Max Planck Pre-doctoral School 2020. In June 2022, he was selected as a member for the first HuggingFace Student Ambassador program.
Don’t recommend the obvious: estimate probability ratios (26 September, 2022)
Speaker: Wenjie Zhao
Abstract
Sequential recommender systems are becoming widespread in the online retail and streaming industry. These systems are often trained to predict the next item given a sequence of a user’s recent actions, and standard evaluation metrics reward systems that can identify the most probable items that might appear next. However, some recent papers instead evaluate recommendation systems with popularity-sampled metrics, which measure how well the model can find a user’s next item when hidden amongst generally-popular items. We argue that these popularity-sampled metrics are more appropriate for recommender systems, because the most probable items for a user often include generally-popular items. If the probability that a customer will watch Toy Story is not much more probable than for the average customer, then the movie isn’t especially relevant for them and we should not recommend it. This paper shows that optimizing popularity-sampled metrics is closely related to estimating point-wise mutual information (PMI). We propose and compare two techniques to fit PMI directly, which both improve popularity-sampled metrics for state-of-the-art recommender systems. The improvements are large compared to differences between recently-proposed model architectures.
Bio
Wenjie Zhao is an applied scientist at Amazon Scotland Development Centre. She works on various topics in the area of recommenders systems. Her main focus is to build and improve large-scale deep learning recommenders for Amazon retail website. She received her MS in AI from the University of Edinburgh and her BS in Maths and Information Engineering from the Chinese University of Hong Kong.
Machine Learning in Science Conference (27 July, 2022)
Speaker: Chris Williams, Michel Besserve, Brendan Tracey
Machine Learning in Science would like to invite you to take part in our first ever conference. We hope to bring researchers together to share knowledge on machine learning, foster interdisciplinary collaboration and enhance research
Aligning existing information-seeking processes with Conversational Information Seeking (25 July, 2022)
Speaker: Johanne Trippas
Abstract:
Conversational information seeking (CIS) research is moving rapidly in various directions, including user interaction, system design, and evaluation. This talk focuses on the theoretical foundations and information-seeking processes for CIS. I will cover theoretical concepts in CIS, provide background in existing CIS systems, including spoken dialogue systems, voice user interfaces, chatbots, and live chat support, and align existing information-seeking processes with CIS. I will illustrate CIS's possible interactions and functional goals through the lens of information search behaviours.
Bio:
Johanne Trippas is a Vice-Chancellor’s Research Fellow at the School of Computing Technologies, RMIT University. Recently, her work has focused on developing next-generation capabilities for intelligent systems, including spoken conversational search, digital assistants in cockpits, and artificial intelligence to identify cardiac arrests. She completed her PhD in Computer Science investigating Conversational Systems at RMIT University in 2019 under the supervision of Professor Lawrence Cavedon, Professor Mark Sanderson, and Doctor Damiano Spina. She was awarded the RMIT University Deputy Vice-Chancellor’s Higher Degree by Research Prize for her doctoral work and thesis. Previously, she was a Doreen Thomas Research Fellow at the University of Melbourne. She has been an ACM SIGIR Student Liaison, and has co-organized tutorials and workshops at ACM SIGIR and ACM CHIIR. Johanne is appointed ACM SIGIR Artifact Evaluation Committee vice-chair and ACM CHIIR Steering Committee member. She currently serves as ACM SIGIR 2022 Workshops Chair and ACM CUI 2022 Full Papers Chair.
The event will be held in a hybrid format.
Please register on eventbrite to participate in person:
https://www.eventbrite.co.uk/e/aligning-existing-information-seeking-processes-with-cis-tickets-388056406157
If you are not able to attend in person and planning to participate online, please register in zoom:
https://uofglasgow.zoom.us/meeting/register/tJ0oceGupjkjH9ylCY_eItc3Ua7AfUaxk2Di
Complex question and clarifications (06 July, 2022)
Speaker: Mark Sanderson
Abstract:
I will present the latest research from the RMIT IR group focusing on complex question answering and clarifications in conversational systems. The talk will be a tour of research that has been conducted in the last year at RMIT. I will also present brief overviews of some of the other research that we have conducted in the last few years to build our understanding of complex question answering and how people currently, or in future, will interact with such systems. I will also detail what we think is a key challenge that we think information retrieval and question answering systems will need to tackle in the future, understanding where queries/questions come from and how to manage the incredible variation in search strategies that people display when searching for information.
Bio:
Mark Sanderson is the Dean for Research for the schools of Engineering and Computing Technologies at RMIT University. Mark is also the head of the information retrieval group at RMIT. Mark studied for his PhD at the University of Glasgow completing in 1997. He was one of the founding members of Glasgow’s IR group. Mark has published over 250 papers and supervised 30 PhD students.
The event will be held in a hybrid format.
If you are planning to participate in person, please register on eventbrite:
https://www.eventbrite.co.uk/e/complex-question-and-clarifications-tickets-377294517057
If you are planning to participate online, please register in zoom:
https://uofglasgow.zoom.us/meeting/register/tJ0oceGupjkjH9ylCY_eItc3Ua7AfUaxk2Di
Efficient Neural Ranking using Forward Indexes (04 July, 2022)
Speaker: Jurek Leonhardt
Abstract:
Neural document ranking approaches, specifically transformer models, have achieved impressive gains in ranking performance. However, query processing using such over-parameterized models is both resource and time intensive. In this paper, we propose the Fast-Forward index -- a simple vector forward index that facilitates ranking documents using interpolation of lexical and semantic scores -- as a replacement for contextual re-rankers and dense indexes based on nearest neighbor search. Fast-Forward indexes rely on efficient sparse models for retrieval and merely look up pre-computed dense transformer-based vector representations of documents and passages in constant time for fast CPU-based semantic similarity computation during query processing. We propose index pruning and theoretically grounded early stopping techniques to improve the query processing throughput. We conduct extensive large-scale experiments on TREC-DL datasets and show improvements over hybrid indexes in performance and query processing efficiency using only CPUs. Fast-Forward indexes can provide superior ranking performance using interpolation due to the complementary benefits of lexical and semantic similarities.
Bio:
Jurek Leonhardt is a PhD student at L3S Research Center, Leibniz University Hannover, Germany. His advisor is Prof. Avishek Anand. Jurek mainly works on information retrieval. Specifically, his current focus lies on efficiency of neural ranking models. Less prominently, Jurek works on effectiveness and interpretability (by design) for ranking and retrieval. Jurek research interests include Efficient and Effective Neural Ranking, and Interpretable Models for Information Retrieval.
The event will be held in a hybrid format.
If you are planning to participate in person, please register on eventbrite:
https://www.eventbrite.co.uk/e/efficient-neural-ranking-using-forward-indexes-tickets-373193621167
If you are planning to participate online, please register in zoom:
https://uofglasgow.zoom.us/meeting/register/tJ0oceGupjkjH9ylCY_eItc3Ua7AfUaxk2Di
Item- and Sequence-level Contrastive Learning in Sequential Recommendation (20 June, 2022)
Speaker: Ruihong Qiu
Abstract:
Sequential recommendations aim to recommend items, such as products, songs and places, to users based on the sequential patterns of their historical records. Most existing sequential recommender models consider the next item prediction task as the training signal. According to our observation, there is a core issue in existing methods that the learned embedding of items or sequences are not sufficiently representative. In this talk, two novel methods in solving the problem for item and sequence embeddings will be presented. (1) MMInfoRec (ICDM 2021), a novel sequential recommendation framework that overcomes the challenges in item embeddings based on a memory augmented multi-instance contrastive predictive coding scheme (2) DuoRec (WSDM 2022), a novel sequential recommendation framework that solves a representation degeneration problem in item embeddings by regularising the sequence embeddings with contrastive learning.
Bio:
Ruihong Qiu is a Postdoctoral Research Fellow at the University of Queensland. He received his PhD in Computer Science at the University of Queensland in 2022 and BSc in Electrical Engineering at Beihang University in 2018. His research interests focus on theory and application of recommender system. Ruihong’s research outcomes are published at SIGIR, ACM MM, WSDM, ICDM, CIKM, TOIS, etc. He has also served as a PC member or a reviewer of CIKM, WSDM, SIGIR, VLDB, TKDE and TOIS.
The event will be held in a hybrid format.
If you are planning to participate online, please register in zoom ("register for event" link above)
If you are planning to join in person, please register in eventbrite:
Recommender systems: From shallow learning to deep learning (13 June, 2022)
Speaker: Chang-Dong Wang
Abstract:
With the population of the Internet and rich internet services, recommender systems have become a widely used fundamental technique. The conventional recommender systems mainly rely on the shallow learning, such as collaborative filtering and content-based recommendation. Due to the capability of learning complex user-item nonlinear relation, deep learning has attracted an increasing amount of attention in recent years. In this talk, we will introduce recommender systems from shallow learning to deep learning, emphasizing their intrinsic differences, in particular, the perspective of performance and explainability.
Bio: Chang-Dong Wang received the Ph.D. degree in computer science in 2013 from Sun Yat-sen University, Guangzhou, China. He was a visiting student at University of Illinois at Chicago from Jan. 2012 to Nov. 2012. He joined Sun Yat-sen University in 2013, where he is currently an associate professor with School of Computer Science and Engineering. His current research interests include machine learning and data mining. He has published over 70 scientific papers in international journals and conferences such as IEEE TPAMI, IEEE TKDE, IEEE TCYB, IEEE TNNLS, KDD, AAAI, IJCAI. His ICDM 2010 paper won the Honorable Mention for Best Research Paper Awards. He won 2012 Microsoft Research Fellowship Nomination Award. He was awarded 2015 Chinese Association for Artificial Intelligence (CAAI) Outstanding Dissertation. He is an Associate Editor in Journal of Artificial Intelligence Research (JAIR).
The event will be held in a hybrid format.
If you are planning to participate online, please register in zoom:
https://uofglasgow.zoom.us/meeting/register/tJ0oceGupjkjH9ylCY_eItc3Ua7AfUaxk2Di
If you are planning to participate in person, please register on eventbrite:
https://www.eventbrite.co.uk/e/recommender-systems-from-shallow-learning-to-deep-learning-tickets-358607704297
Responsible Information Access Beyond Fairness (06 June, 2022)
Speaker: Asia Biega
Abstract
To be fully responsible, information access systems should account for a variety of social, legal, and ethical principles. These principles apply not only to the underlying algorithms, but also other system facets, including data and user interfaces. In this talk, I will discuss a few responsibilty concepts which are currently driving our research, including data minimization, nudging, and digital well-being.
Bio
Asia J. Biega is a tenure-track faculty member at the leading the Responsible Computing group. Her research centers around developing, examining and computationally operationalizing principles of responsible computing, data governance & ethics, and digital well-being.
Before joining MPI-SP, Asia worked at , winning the DBIS Dissertation Award of the German Informatics Society.
In her work, Asia engages in interdisciplinary collaborations while drawing from her traditional CS education and her industry experience, including consulting and engineering stints at Microsoft, Google and in e-commerce.
The event will be held in a hybrid format.
For in-person participation, please register on Eventbrite:
https://www.eventbrite.co.uk/e/responsible-information-access-beyond-fairness-tickets-334664218687
For online participation, please register in zoom
Search Among Sensitive Content (30 May, 2022)
Speaker: Mahmoud Sayed
Abstract
Current search engines are designed to find what we want. But many collections can not be made available for search engines because they contain sensitive content that needs to be protected. Before release, such content needs to be examined through a sensitivity review process, which can be difficult and time-consuming. To address this challenge, search technology should be capable of providing access to relevant content while protecting sensitive content. First, in this talk, I will present an approach that leverages evaluation-driven information retrieval (IR) techniques. These techniques optimize an objective function that balances the value of finding relevant content with the imperative to protect sensitive content. This requires evaluation measures that balance between relevance and sensitivity. Baselines are introduced for addressing the problem, and a proposed approach that is based on building a listwise learning to rank model is described. The model is trained with a modified loss function to optimize for the evaluation measure. Second, I will describe a new test collection for the sensitivity-aware retrieval task, which is based on the Avocado Research Email Collection. Finally, I will describe a new public test collection with annotations for one class of exempt material subject to the deliberative process privilege, which is used for studying the ability of text classification techniques to identify those materials that are exempt from release under that privilege.
Bio
Mahmoud Sayed is a Data Scientist at Microsoft. He received his Ph.D. from the University of Maryland, College Park, under the supervision of Prof. Doug Oard. His research interests are Information Retrieval and Machine Learning. In particular, he is interested in multi-criteria learning to rank
The event will be held in a hybrid format.
For in-person participation, please register on Eventbrite:
https://www.eventbrite.co.uk/e/search-among-sensitive-content-tickets-349072283597
For online participation, please register in zoom
Neural Query Performance Prediction (23 May, 2022)
Speaker: Suchana Datta
Abstract
The first part of the talk will present a recently proposed neural cross-encoder based query performance prediction (QPP) approach. Supervised approaches for QPP are often trained on pairs of queries to capture their relative retrieval performance. However, pointwise approaches are generally preferable for efficiency reasons. With this motivation, the speaker will present a proposed novel end-to-end neural cross-encoder based approach that was trained pointwise on individual queries, but listwise over the top ranked documents (split into chunks).
The second part of the talk will present the feasibility and robustness of pointwise query performance prediction evaluation. The speaker will explain a pointwise QPP evaluation framework to evaluate the quality of a QPP system for individual queries by measuring the similarities in each predicted vs. true value, and eventually aggregate these over a set of queries.
Bio
Suchana Datta is a second-year PhD student at the School of Computer Science, University College Dublin, Ireland. She is also a member of the Machine learning group of Insight Centre for Data Analytics. Suchan is working under the supervision of Dr. Derek Greene from University College Dublin and Dr. Debasis Ganguly from the University of Glasgow. Her research activities span topics on Information Retrieval (IR) and Natural Language Processing (NLP). More specifically, Suchana is pursuing novel search interfaces and algorithms towards addressing causality-driven search, i.e. where a search user wants to find out documents answering the ‘Why’ questions. Her research interests also include deep neural network applications in various IR problems, more specifically QPP.
The event will be held in a hybrid format.
For in-person participation, please register on Eventbrite:
https://www.eventbrite.co.uk/e/neural-query-performance-prediction-tickets-345606547487
For online participation, please register in zoom
Applied research for product development (16 May, 2022)
Speaker: Dyaa Albakour
Abstract
Conducting research in a product development environment comes with unique challenges. Firstly, product development is a fast moving environment, which requires a lean approach to ensure incremental improvements. Secondly, it requires effective collaboration with teams and individuals of diverse skill sets. Also, among the challenges, is the gap between theoretical quality metrics, such as precision and recall, and the impact on business metrics or the user satisfaction. Finally, deploying a new solution, for example a new ranking algorithm, into a production system comes with operational complexities such as cost and latency.
In this talk, the speaker will highlight the learnings from his experience at Signal AI in applying Information Retrieval (IR) and Natural Language Processing (NLP) to build a large-scale decision augmentation platform. Processing millions of documents a day, the platform is used by thousands of professionals globally for reputation management and business intelligence. The speaker will give practical examples on how we tackle the above challenges with a pragmatic and experimental-driven approach.
Bio
Dyaa Albakour is an applied researcher in IR and NLP. He holds a PhD degree in Information Retrieval from the University of Essex (2012). Since September 2015, he has worked at Signal AI, where he currently leads the development of AI-powered products for decision augmentation. Prior to that, Dyaa was a post-doctoral researcher in the Terrier IR research group at the University of Glasgow.
The event will be held in a hybrid format.
For in-person participation, please register on Eventbrite:
https://www.eventbrite.co.uk/e/applied-research-for-product-development-tickets-338846768807
For online participation, please register in zoom
Different sides of the same coin? (25 April, 2022)
Speaker: Fiana Raiber
Abstract
Various tasks and challenges in the information retrieval field have been independently addressed in the literature, including federated search, fusion-based retrieval, cluster ranking, and query-performance prediction. Using a general probabilistic formalism, we draw novel connections between these tasks and the methods used to address them. Then, we focus on two of the tasks: query-performance prediction and cluster ranking tasks. The first is predicting the effectiveness of retrieval performed in response to a query with no relevance judgments. The second is predicting the effectiveness of clusters created from the documents most highly ranked by some search performed in response to the query with no relevance judgments. We present a novel approach for cluster ranking that utilizes Markov Random Fields and study the merits of applying a similar approach based on the same principles to predict query performance.
Bio
Fiana Raiber is a senior manager of the text research team at Yahoo. She earned her Ph.D. in information management engineering from the Technion - Israel Institute of Technology, where she also completed postdoctoral studies. Currently, she holds the position of a visiting scientist, collaborating with faculty members and graduate students. Fiana is a co-author of over 30 conference and journal papers in the field of information retrieval. Her research interests include cluster ranking, query-performance prediction, and adversarial search. She currently serves as the SIGIR short papers program co-chair. She is a (senior) program committee member of numerous conferences, including SIGIR, ICTIR, WSDM, and CIKM.
The event will be held in a hybrid format.
For in-person participation, please register on Eventbrite:
https://www.eventbrite.co.uk/e/different-sides-of-the-same-coin-tickets-324017253327
For online participation, please register in zoom
Reproducing Personalised Session Search over the AOL Query Log, or How to Reconstruct a Historic Web Corpus (04 April, 2022)
Speaker: Sean MacAvaney
Abstract
Despite its troubled origins, the AOL Query Log is an important resource for the IR community, representing the only large-scale web dataset that includes users and search sessions. The dataset does not contain textual information about the documents referenced, so researchers instead pull modern versions of the documents from the web. In the 16 years since the log was taken, most pages referenced have changed substantially or no longer exist at all. In this work, we show that a more genuine and complete version of the corpus can be generated using the Web Archive's Wayback Machine. As a bonus, the dataset is more available to the community, since anybody can download the content themselves. Using this technique, we show far more pages can be recovered (from 55% of pages to 93% of pages), with 84% of the pages coming from a 6-month window preceding and encapsulating the time of the log. Improved completeness of the log allow us to recover more and longer sessions from the log for training and evaluating personalised search algorithms. We achieve mixed results when we try to reproduce several personalised search algorithms using both our version of the corpus and a commonly-used version scraped in 2017. Finally, we establish new performance baselines using modern ad-hoc re-ranking approaches, and find that appending the URL to the content can improve performance across the board -- given that many queries in the log are navigational in nature.
Bio
Sean MacAvaney is a Lecturer in Machine Learning at the University of Glasgow. His main research focuses on deep learning techniques for building effective, efficient, and interpretable search ranking algorithms. He did his PhD on this topic at Georgetown University's IRLab, under the supervision of Ophir Frieder and Nazli Goharian. He has received the Allen Institute for AI Intern of the Year Award, the Georgetown IR Lab Service Award, and the ARCS Endowment Fellowship.
The event will be held in a hybrid format.
For in-person participation, please register on Eventbrite:
https://www.eventbrite.co.uk/e/reproducing-personalised-session-search-over-the-aol-query-log-tickets-310539129897
For online participation, please register in zoom
Different sides of the same coin? (28 March, 2022)
Speaker: Fiana Raiber
Abstract
Various tasks and challenges in the information retrieval field have been independently addressed in the literature, including federated search, fusion-based retrieval, cluster ranking, and query-performance prediction. Using a general probabilistic formalism, we draw novel connections between these tasks and the methods used to address them. Then, we focus on two of the tasks: query-performance prediction and cluster ranking tasks. The first is predicting the effectiveness of retrieval performed in response to a query with no relevance judgments. The second is predicting the effectiveness of clusters created from the documents most highly ranked by some search performed in response to the query with no relevance judgments. We present a novel approach for cluster ranking that utilizes Markov Random Fields and study the merits of applying a similar approach based on the same principles to predict query performance.
Bio
Fiana Raiber is a senior manager of the text research team at Yahoo. She earned her Ph.D. in information management engineering from the Technion - Israel Institute of Technology, where she also completed postdoctoral studies. Currently, she holds the position of a visiting scientist, collaborating with faculty members and graduate students. Fiana is a co-author of over 30 conference and journal papers in the field of information retrieval. Her research interests include cluster ranking, query-performance prediction, and adversarial search. She currently serves as the SIGIR short papers program co-chair. She is a (senior) program committee member of numerous conferences, including SIGIR, ICTIR, WSDM, and CIKM.
The event will be held in a hybrid format.
For in-person participation, please register on Eventbrite:
https://www.eventbrite.co.uk/e/different-sides-of-the-same-coin-tickets-305509425917
For online participation, please register in zoom
Neural Symbolic Processing - Effectively Handling Knowledge and Reasoning (21 March, 2022)
Speaker: Hang Li
Abstract
In recent years, deep learning has made remarkable achievements in artificial intelligence. Deep learning technologies cannot effectively deal well with knowledge and reasoning, however, the main components of human intelligence. Neural symbol processing, manages to combine neural processing and traditional symbol processing to solve this problem, which is also a hot topic of recent research. In this talk, the speaker will introduce two pieces of our recent work on neural symbolic processing. One is to use the seq2seq technology to extract information from a text and turn it into structured information, which we call text-to-table. The other is to perform neural symbol processing for language understanding, not only leveraging neural networks to conduct analogical reasoning, but also leveraging neural networks to generate programs and execute programs to conduct logical reasoning.
Bio
Hang Li is currently a Director of the AI Lab at ByteDance Technology. He is also a Fellow of ACL, Fellow of IEEE, and Distinguished Scientist of ACM. He graduated from Kyoto University and earned his Ph.D. from the University of Tokyo. He worked at NEC Research as researcher and at Microsoft Asia Research as senior researcher and research manager. He was a director and chief scientist of Noah's Ark Lab of Huawei Technologies prior he joined ByteDance.
The event will be held in a hybrid format.
For in-person participation, please register on Eventbrite:
https://www.eventbrite.co.uk/e/neural-symbolic-processing-effectively-handling-knowledge-and-reasoning-tickets-294083711297
For online participation, please register in zoom
https://uofglasgow.zoom.us/meeting/register/tJ0oceGupjkjH9ylCY_eItc3Ua7AfUaxk2Di
Principled Multi-Aspect Evaluation Measures of Rankings (14 March, 2022)
Speaker: Maria Maistro
Abstract
Information Retrieval evaluation has traditionally focused on defining principled ways of assessing the relevance of a ranked list of documents with respect to a query. Several methods extend this type of evaluation beyond relevance, making it possible to evaluate different aspects of a document ranking (e.g., relevance, usefulness, or credibility) using a single measure (multi-aspect evaluation). However, these methods either are (i) tailor-made for specific aspects and do not extend to other types or numbers of aspects, or (ii) have theoretical anomalies, e.g., assign maximum score to a ranking where all documents are labelled with the lowest grade with respect to all aspects (e.g., not relevant, not credible, etc.).
We present a theoretically principled multi-aspect evaluation method that can be used for any number, and any type, of aspects. A thorough empirical evaluation using up to 5 aspects and a total of 425 runs officially submitted to 10 TREC tracks shows that our method is more discriminative than the state-of-the-art and overcomes theoretical limitations of the state-of-the-art.
Bio
Maria Maistro studied initially Mathematics (BSc, University of Padua, 2011; MSc, University of Padua, 2014) and then Computer Science (PhD, University of Padua, 2018). She is a Marie Curie fellow and a tenure track assistant professor at the Department of Computer Science, University of Copenhagen (DIKU). Prior to this, she was a postdoctoral researcher at the Department of Computer Science, University of Copenhagen (DIKU) and at the University of Padua in Italy. She conducts research in information retrieval, and particularly on evaluation, reproducibility and replicability, click log analysis, expert search, learning to rank and applied machine learning. She has already co-organized several international scientific events (e.g., reproducibility track chair ECIR 2021, tutorial track chair SIGIR 2022), among which tracks in evaluation campaigns (i.e., at TREC, CLEF and NTCIR) and international educational events (e.g., Malawi Data Science Bootcamp). She has served as member of programme committees and reviewer for highly ranked conferences and journals in information retrieval. Her teaching interests include web science, information retrieval, database management systems, and web applications.
The event will be held in a hybrid format.
For in-person participation, please register on Eventbrite:
https://www.eventbrite.co.uk/e/principled-multi-aspect-evaluation-measures-of-rankings-tickets-294078144647
For online participation, please register in zoom
https://uofglasgow.zoom.us/meeting/register/tJ0oceGupjkjH9ylCY_eItc3Ua7AfUaxk2Di
Sequential and Session-Based Recommender Systems (07 March, 2022)
Speaker: Gabriel de Souza Pereira Moreira
Abstract
Recommender systems help users to find relevant content, products, media and much more in online services. They also help such services to connect their long-tailed (unpopular) items to the right people, to keep their users engaged and increase conversion.
Traditional recommendation algorithms, e.g. collaborative filtering, usually ignore the temporal dynamics and the sequence of interactions when trying to model user behaviour. But users’ preferences do change over time. Sequential recommendation algorithms can capture sequential patterns in users browsing might help to anticipate the next user interests for better recommendation. For example, users getting started into a new hobby like cooking or cycling might explore products for beginners, and move to more advanced products as they progress over time. They might also completely move to another topic of interest, so that recommending items related to their long past preferences would become irrelevant.
A special case of sequential-recommendation is the session-based recommendation task, where you have only access to the short sequence of interactions within the current session. This is very common in online services like e-commerce, news and media portals, where the user might be brand new or prefer to browse anonymously (and due to GDPR compliance no cookies are collected). This task is also relevant for scenarios where users’ interests change a lot over time depending on the user context or intent, so leveraging the current session interactions is more promising than old interactions to provide relevant recommendations.
In this talk, it will be presented an overview of the sequential and session-based recommendation tasks, the recent Deep Learning architectures inspired in NLP (RNNs and Transformers) that have been used to tackle those problems and how you can train and build end-to-end recsys pipelines using the tools we have been building for NVIDIA Merlin - an open source platform for large-scale recommender systems - which includes the Transformers4Rec library.
Bio
Gabriel Moreira is a Senior Applied Research Scientist at NVIDIA, leading Merlin team research efforts on recommender systems and also working in the development of Merlin libraries like Transformers4Rec and Merlin Models. He has his PhD degree from Instituto Tecnológico de Aeronáutica (ITA), Brazil with a focus on Deep Learning for RecSys and Session-based recommendation. Before joining NVIDIA, he was Lead Data Scientist at CI&T -- a digital transformation consulting company -- for 5 years, after working as software engineer for more than a decade. In 2019, he was recognized as a Google Developer Expert (GDE) for Machine Learning.
The event will be held in a hybrid format.
For in-person participation, please register on Eventbrite:
https://www.eventbrite.co.uk/e/sequential-and-session-based-recommender-systems-tickets-289462308557
For online participation, please register in zoom
https://uofglasgow.zoom.us/meeting/register/tJ0oceGupjkjH9ylCY_eItc3Ua7AfUaxk2Di
Recommender Systems & Diversity of Consumption (28 February, 2022)
Speaker: Lucas Maystre
Abstract
We study the user experience on Spotify through the lens of diversity—the coherence of the set of songs a user listens to. We quantify how musically diverse every user is by taking advantage of a high-fidelity song embedding. We find that high consumption diversity is strongly associated with important long-term user metrics, such as conversion and retention. However, we also find that algorithmically-driven listening through recommendations is associated with reduced consumption diversity. Furthermore, we observe that when users become more diverse in their listening over time, they do so by shifting away from algorithmic consumption and increasing their organic consumption. Our work illuminates a central tension in online
platforms: how do we recommend content that users are likely to enjoy in the short term while simultaneously ensuring they can remain diverse in their consumption in the long term?
Bio
Lucas Maystre is a research scientist at Spotify, working on improving users' long-term engagement and satisfaction. His research interests revolve around probabilistic machine learning and range from designing effective models to developing computationally-efficient inference algorithms. He received a PhD from EPFL, supported by a Google fellowship in Machine Learning.
The event will be held in a hybrid format.
For online participation, please register in zoom:
https://uofglasgow.zoom.us/meeting/register/tJ0oceGupjkjH9ylCY_eItc3Ua7AfUaxk2Di
For in-person participation, please register on Eventbrite:
https://www.eventbrite.com/e/recommender-systems-diversity-of-consumption-tickets-274629894407
Recommending people in social networks: algorithmic models and network diversity (21 February, 2022)
Speaker: Javier Sanz-Cruzado Puig
Abstract:
Contact recommendation appears as one of the most relevant problems in the confluence between recommender systems and online social networks. The goal of this problem is to identify those people in a social network with whom a user might be interested to connect. In this seminar, we explore two different aspects of contact recommendation.
First, we explore the design of novel and effective algorithms, looking to increase the density of the network. For this, we adapt classical information retrieval models to recommend people in social networks and use them in three different tasks: as direct recommenders, as similarity measures in nearest neighbours schemes and as samplers and features in learning to rank.
Next, we consider the potential of contact recommendation algorithms to drive the evolution of networks towards desirable properties of the network. We investigate the definition of novel metrics that quantify the effects of recommendations over the network and analyse how these changes might affect the users of the network.
Bio:
Javier Sanz-Cruzado is a post-doctoral research associate at the Terrier Team at the University of Glasgow, working on the EU Infinitech project with Prof. Iadh Ounis, Dr. Craig Macdonald and Dr. Richard McCreadie. Currently, he investigates the application of recommender systems in the financial domain.
Previously, he obtained a PhD in Computer Science from Universidad Autónoma de Madrid, under the supervision of Prof. Pablo Castells. During his doctoral studies, he explored the task of recommending people in online social networks.
This event will be in a hybrid format. For in-person participation please register by link:
https://www.eventbrite.co.uk/e/recommending-people-in-social-networks-models-and-network-diversity-tickets-269544433657
Modelling for Millions of Users: Tales from Question Answering at Scale (14 February, 2022)
Speaker: Luca Soldaini
Abstract
Every day, millions of users interact with virtual voice assistants (VVA) to seek information on a wide range of topics. To be able to effectively answer such a broad variety of questions, VVAs leverage Web Question Answering (WQA) systems. WQA systems combine a large-scale search engine with an efficient answer selection pipeline to quickly find relevant answers for their users. In this talk, Luca is going to discuss the overall design of WQA systems and related challenges; then, they will present three approaches to increase their efficiency and effectiveness. The first two are transformer models designed to increase QA throughput and reduce latency while guaranteeing high-quality answers; for the third one, Luca will discuss how natural language generation models can be used to improve the fluidness of answers returned to users, both in English as well as in other languages. Taken together, these three research projects can significantly improve the speed and accuracy of WQA systems.
Bio
Luca Soldaini is an Applied Research Scientist at the Allen Institute for AI working on Semantic Scholar. Their current research interests are question answering and information retrieval systems operating at scale. Before joining AI2, they were an Applied Scientist at Amazon Alexa. Luca obtained their Ph.D. in Computer Science from Georgetown University; during their doctoral studies, they investigated approaches to help health professionals and laypeople find trustworthy and relevant medical information online. Luca's research has been published in top-tier NLP and IR conferences, such as ACL, NAACL, WWW, and SIGIR. They have served as Senior Area Chair for NAACL 2022, Area Chair for ACL, NAACL, ARR, and AAAI, and were part of the D&I committee at NAACL 2021. Luca is also a Core Organizer at Queer In AI, a nonprofit dedicated to raising awareness of queer issues in AI and fostering an inclusive community for queer researchers.
This event will be in a hybrid format. For in-person participation please register by link:
https://www.eventbrite.co.uk/e/modelling-for-millions-of-users-tales-from-question-answering-at-scale-tickets-265823183297
What’s the matter with IR evaluation measures? Scales? Significance Tests? Meaningfulness? (07 February, 2022)
Speaker: Nicola Ferro
Abstract
The main goal of Information Retrieval experimentation is to determine the effectiveness of IR systems and to compare them in order to determine the best approaches. Evaluation measures are the way to quantify the effectiveness of IR systems and their scores are then used in follow-up statistical analyses, aimed at drawing inferences about the analysed systems and how they would perform once in production.
However, evaluation measures are based on measurement scales which, in turn, determine the allowable operations with scores from those scales. For example, mean and variance should be computed only when relying on interval scales as well as parametric significance tests. Departing from scale properties causes a bias in the evaluation outcomes and, especially, affects the meaningfulness of the conclusions drawn, i.e. their invariance with respect to allowable transformations of a measurement scale.
Currently, there is a lot of debate in the IR community about whether we should strictly adhere to scale properties or not and what would be the implications of a choice or another. Independently from the stance you take, it is a matter of fact that these issues have been completely overlooked so far while they should be carefully addressed.
In this talk, we will introduce the fundamental notions about scales of measurement and meaningfulness, and we will show how they apply to IR evaluation measures. Unfortunately, most IR evaluation measures are not interval scales and those depending on the recall base will never be. However, we will propose an approach to transform measures not depending on the recall base into proper interval scale. Finally, we will discuss the outcomes of a thorough experimentation on TREC collection, deeply analysing the impact of departing from scale assumption and showing that, on average, 25% of the decisions about which systems are significantly different will change because of the scale properties of IR evaluation measures.
Main References
- Ferrante, M., Ferro, N., and Pontarollo, S. (2019). A General Theory of IR Evaluation Measures. IEEE Transactions on Knowledge and Data Engineering (TKDE), 31(3):409–422.
- Ferrante, M., Ferro, N., and Losiouk, E. (2020). How do interval scales help us with better understanding IR evaluation measures?. Information Retrieval Journal, 23(3):289-317.
- Ferrante, M., Ferro, N., and Fuhr, N. (2021). Towards Meaningful Statements in IR Evaluation. Mapping Evaluation Measures to Interval Scales. IEEE Access, 9: 136182-136216.
Bio
Nicola Ferro is full professor of computer science at the University of Padua, Italy. His research interests include information retrieval, its experimental evaluation, multilingual information access and digital libraries and he published more than 350 papers on these topics. He is the chair of the CLEF evaluation initiative, which involves more than 200 research groups world-wide in large-scale IR evaluation activities. He was the coordinator of the EU 7FP Network of Excellence PROMISE on information retrieval evaluation. He is associate editor of ACM TOIS and was general chair of ECIR 2016, short papers program co-chair of ECIR 2020, resource papers program co-chair of CIKM 2021.
Probing and infusing biomedical knowledge for pre-trained language models (31 January, 2022)
Speaker: Zaiqiao Meng
Abstract
Pre-trained language models (PLMs) have orchestrated incredible progress on myriads of few- or zero-shot language understanding tasks, by pre-training model parameters in a task-agnostic way and transferring knowledge to specific downstream tasks via finetuning. Leveraging factual knowledge from knowledge graphs (KGs) to augment PLMs is of paramount importance for knowledge-intensive tasks, such as question answering and fact checking. Especially in the biomedical domain where public training corpora are limited and noisy, trusted biomedical KGs are crucial for deriving accurate inferences. Zaiqiao will introduce one our proposed knowledge infusion approach, named Mixture-of-Partitions (MoP), which is to infuse factual knowledge based on partitioned KGs into PLMs, and automatically route useful knowledge from these adapters to downstream tasks. Knowledge probing is another crucial task for understanding the knowledge transfer mechanism behind the PLMs. Despite the growing progress of probing knowledge for PLMs in the general domain, specialised areas such as biomedical domain are vastly under-explored. Zaiqiao will also introduce a new biomedical knowledge probing benchmark, namely MedLAMA, and a novel probing approach, namely Contrastive Probe, for probing biomedical knowledge of PLMs.
Bio
Zaiqiao is currently a Lecturer at the IDA section of the University of Glasgow. He was previously working as a Postdoctoral Researcher at the Language Technology Laboratory of the University of Cambridge, and at the Terrier team of the University of Glasgow, respectively. Zaiqiao obtained his Ph.D. in computer science from Sun Yat-sen University in December 2018. His research interests include information retrieval, recommender systems, graph neural networks, knowledge graphs and NLP.
Do I really have to read that paper? (17 January, 2022)
Speaker: Jake Lever
Abstract
Biomedical researchers face an overwhelming number of papers to read as their research becomes increasingly interdisciplinary. We must build automated methods to help them digest this vast knowledge and guide them to new research directions. Information extraction methods offer the opportunity to intelligently summarize the combined biomedical knowledge locked in decades of research articles. This talk will explore some of these unique challenges in biomedical applications of natural language processing.
Bio
Jake is a new lecturer in the School of Computing Science with a focus on biomedical text mining. He did his postdoctoral research at Stanford University and completed his Ph.D. at the University of British Columbia in Vancouver, Canada.
Effective and Efficient Dense Retrieval via Joint Optimization with Compact Index (10 January, 2022)
Speaker: Jingtao Zhan
Abstract
Information Retrieval community has witnessed fast-paced advances in Dense Retrieval (DR), which performs first-stage retrieval with embedding-based search. In many practical embedding-based retrieval applications, approximate nearest neighbor search (ANNS) is needed to build compact and fast embedding indexes. However, existing ANNS trades effectiveness for efficiency and trivially applying ANNS to DR causes severe effectiveness loss. This talk will present two recent related publications (JPQ, RepCONC) and show that joint optimization of dual-encoders and ANNS methods is a promising solution to this issue. The speaker will discuss why joint optimization could achieve substantial performance gains and how to tackle the non-differentiability problem in this joint optimization framework.
Bio
Jingtao Zhan is a PhD student at Tsinghua University supervised by Prof. Yiqun Liu. He explores deep learning methods in web search with a focus on efficiency and explainability. He has published several papers in multiple top-tier IR conferences and served as PC member for WSDM 2022.
Neural Machine Translation Inside Out (06 December, 2021)
Speaker: Lena Voita
Elena (Lena) Voita is a PhD student at the University of Edinburgh and the University of Amsterdam supervised by Ivan Titov and Rico Sennrich and supported by the Facebook PhD Fellowship. She is mostly interested in understanding what and how neural models learn; she also worked quite a lot on (mostly document-level) neural machine translation. Previously, Lena spent 4 years at different parts of Yandex; 2.5 of them as a research scientist at Yandex Research side by side with the Yandex Translate team. She also teaches NLP at the Yandex School of Data Analysis; the extended public version of (a part of) this course is available at "NLP Course For You".
Footprint of Societal Biases in Information and Language Processing (29 November, 2021)
Speaker: Navid Rekab-saz
Recent advances in unbiased learning to rank from position-biased click feedback (22 November, 2021)
Speaker: Harrie Oosterhuis
Search and recommendation systems are vital for the accessibility of content on the internet. Search engines allow users to search through large online collections with little effort. Recommendation systems help users discover content that they may not know they find interesting. The basis for these systems are ranking models that turn collections of items into rankings: small ordered lists of items to be displayed to users. Modern ranking models are mostly optimized based on user interactions. Generally, learning from user behavior leads to systems that receive more user engagement than those optimized based on expert judgements. However, user interactions are biased indicators of user preference: often whether something is interacted has less to do with preference and more with where and how it was presented.
In response to this bias problem, recent years have seen the introduction and development of the counterfactual Learning to Rank (LTR) field. This field covers methods that learn from historical user interactions, i.e. click logs, and aim to optimize ranking models w.r.t. the actual user preferences. In order to achieve this goal, counterfactual LTR methods have to correct for the biases that affect clicks.
Bio:
Data-efficient and Explainable Ranking with BERT models (15 November, 2021)
Speaker: Leonid Boytsov
In the second part of the talk, I present our exploration of classical (non-neural) and neural lexical translation models. A lexical translation model (IBM Model 1) explicitly encodes pairwise interactions between query and document tokens: IBM Model 1 can be used both independently or as a special layer applied to contextualized embeddings. I show that applying the neural lexical translation model, which provides partial interpretability, leads to no degradation of accuracy and can slightly boost accuracy compared to using a BERT FirstP model.
Leonid holds a PhD from the Carnegie Mellon University in language technologies (2018) and an MSc/BSc from the Moscow State University (1997) in applied mathematics and computer science.
Overall, Leonid Boytsov has been a professional computer scientist since 1996 working on information retrieval, computer vision, speech recognition, and financial management systems. He remembers dependency parsing and the USSR.
An important by-product of his research is an efficient and flexible library for k-NN search codenamed NMSLIB, which was created in collaboration with several other researchers. NMSLIB has 1M+ downloads. It was adopted by Amazon and incorporated into TensorFlow similarity.
From research to production - bringing the neural search paradigm shift to production (08 November, 2021)
Speaker: Jo Kristian Bergum
Abstract:
Search is going through a paradigm shift, sometimes referred to as the “BERT revolution.” The introduction of pre-trained language transformer models like BERT has brought significant advancements in search and document ranking state-of-the-art.
Bringing these promising methods to production in an end-to-end search serving system is not trivial. It requires substantial middleware glue and deployment effort to connect open-source tools like Apache Lucene, vector search libraries (e.g. FAISS), and model inference servers. However, the open-source serving engine Vespa, which Yahoo has developed since 2003, offers features that enable implementing state-of-the-art retrieval and ranking methods using a single serving engine which reduces the deployment complexity and failure modes significantly.
This talk gives an overview of the Vespa search serving architecture and features enabling expressing state-of-the-art retrieval and ranking methods. We dive into Vespa’s implementations of sub-linear retrieval algorithms for both sparse and dense representations to produce candidate documents for (re-)ranking efficiently. Vespa also allows expressing the end-to-end multi-stage retrieval and ranking pipeline, including inference using transformer models. We also touch on real world application constraints, search result diversification, and how serving search over static research document collections differs from real world search applications.
Bio:
Jo Kristian Bergum works as a distinguished engineer at Yahoo, working primarily on the open source Vespa.ai big data serving engine.
Reinforcement Learning from Reformulations in Conversational Question Answering over Knowledge Graphs (25 October, 2021)
Speaker: Magdalena Kaiser
Abstract:
The rise of personal assistants has made conversational question answering (ConvQA) a very popular mechanism for user-system interaction. State-of-the-art methods for ConvQA over knowledge graphs (KGs) can only learn from crisp question-answer pairs found in popular benchmarks. In reality, however, such training data is hard to come by: users would rarely mark answers explicitly as correct or wrong. In this work, we take a step towards a more natural learning paradigm - from noisy and implicit feedback via question reformulations. A reformulation is likely to be triggered by an incorrect system response, whereas a new follow-up question could be a positive signal on the previous turn's answer. We present a reinforcement learning model, termed CONQUER, that can learn from a conversational stream of questions and reformulations. CONQUER models the answering process as multiple agents walking in parallel on the KG, where the walks are determined by actions sampled using a policy network. This policy network takes the question along with the conversational context as inputs and is trained via noisy rewards obtained from the reformulation likelihood. To evaluate CONQUER, we create and release ConvRef, a benchmark with about 11k natural conversations containing around 205k reformulations. Experiments show that CONQUER successfully learns to answer conversational questions from noisy reward signals, significantly improving over a state-of-the-art baseline.
Bio:
Magdalena Kaiser is a PhD Student in the Databases and Information Systems Group at the Max Planck Institute for Informatics (MPII), Saarbrücken, Germany, under the supervision of Prof. Gerhard Weikum and Dr. Rishiraj Saha Roy. Her research focuses on conversational question answering. In particular, she is interested in leveraging feedback to improve conversational systems. In her work, she applies techniques from Information Retrieval, Natural Language Processing and Machine Learning, particularly Reinforcement Learning. Further information can be found on her website: http://people.mpi-inf.mpg.de/~mkaiser/.
A modular framework for task automation guided by VR Teleoperation (20 October, 2021)
Speaker: Vanja Popovic
Vanja will be presenting his research updates on "A modular framework for task automation guided by VR Teleoperation". This is a Behavioural Based Reinforcement Learning approach where behaviours are trained through Behavioural Cloning from demonstrations and combined into more complex behaviours with Reinforcement Learning. The main aim of the presentation is to share his latest results with the CVAS group, explain the successes and pitfalls and gather feedback from the group.
Query Performance Prediction for Neural Models and With Neural Models (18 October, 2021)
Speaker: Debasis Ganguly
Abstract:
Query performance prediction (QPP) methods, which aim to predict the performance of a query, often rely on evidence in the form of different characteristic patterns in the distribution of retrieval status values (RSVs). However, when we consider neural IR models rather than their statistical counterparts, we find that these RSVs are often less reliable, since they are bounded within a short interval.
To address this limitation, in the first part of the talk, i.e. for the “for” part of my talk, we propose a model-agnostic QPP framework that gathers additional evidences by leveraging information from the characteristic patterns of RSV distributions computed over a set of automatically-generated query variants, relative to that of the current query.
In the second part of the talk we focus on the “with” part, i.e., motivated by the recent success of end-to-end deep neural models for ranking tasks, we present here a supervised end-to-end neural approach for QPP. In contrast to unsupervised approaches that rely on various statistics of document score distributions, our approach is entirely data-driven leveraging information from the semantic interactions between the terms of the query and those of the top-retrieved documents. The architecture of the model comprises multiple layers of 2D convolution filters followed by a feed-forward layer of parameters.
Next, if time permits, I’ll also briefly point out to some of the analysis in variations in the results of QPP approaches in terms of reproducibility.
Bio:
Debasis Ganguly is a lecturer in Data Science at the University of Glasgow. Generally speaking, his research activities span across a wide range of topics on Information Retrieval (IR) and Natural Language Processing (NLP). His research focus is on applications of unsupervised methods leveraging word embeddings for ad-hoc IR, query performance prediction, multi-objective neural networks for fair predictions and privacy-preserved learning, explainability and trustworthiness of ranking models, and defence against adversarial attacks on neural models.
The in-person seminar is enabled with this event and people can register via the following link to join:
https://www.eventbrite.co.uk/e/query-performance-prediction-for-neural-models-and-with-neural-models-tickets-190376861317
A demonstration of a 3D printed robotic arm: Stages from printing to moving arm (13 October, 2021)
Speaker: Ali AlQallaf
Building an entire robotic arm is a multidisciplinary task with different challenges to reach the expected outcomes of a functional robot arm. Moreover, developing and engineering a robot arm has different stages start from a 3D robot arm design and end up with equipment the proper electronics to the arm. Furthermore, I will discuss some potential issues associated with any robotic arm development and their solutions.
Not All Relevance Scores are Equal: Efficient Uncertainty and Calibration Modeling for Deep Retrieval Models (11 October, 2021)
Speaker: Daniel Cohen
Assessing top-𝑘 preferences (04 October, 2021)
Speaker: Charles Clarke
Abstract
NDCG and similar measures remain standard for the offline evaluation of search, recommendation, question answering and similar systems. These measures require definitions for two or more relevance levels, which human assessors then apply to judge individual documents. Due to this dependence on a definition of relevance, it can be difficult to extend these measures to account for factors beyond relevance. Rather than propose extensions to these measures, we instead propose a radical simplification to replace them. For each query, we define a set of ideal rankings and compute the maximum rank similarity between members of this set and an actual ranking generated by a system. This maximum similarity to an ideal ranking becomes our effectiveness measure, replacing NDCG and similar measures. As an example, we extend offline evaluation with preference judgements. Assessors make preference judgments faster and more consistently than graded judgments. Preference judgments can also recognize distinctions between items that appear equivalent under graded judgments. Unfortunately, preference judgments can require more than linear effort to fully order a pool of items, and evaluation measures for preference judgments are not as well established as those for graded judgments, such as NDCG. We explore the assessment process for partial preference judgments, with the aim of identifying and ordering the top items in the pool, rather than fully ordering the entire pool. We demonstrate the practical feasibility of this approach by crowdsourcing partial preferences for the TREC 2019 Conversational Assistance Track. This new approach has its most striking impact when comparing modern neural rankers, where it is able to recognize significant improvements in quality that would otherwise be missed by NDCG.
Biography
Charles Clarke is a Professor in the School of Computer Science at the University of Waterloo, Canada. His research focuses on data intensive tasks and efficiency, including search, ranking, question answering, and other problems involving human language data. He has supervised over 30 graduate students to completion and published over 200 refereed contributions on a wide range of topics, including search, metrics, user interfaces, filesystem search, natural language processing, machine learning, and databases. He has worked on search engine technology for both Microsoft Bing and Facebook Search. Clarke is an ACM Distinguished Scientist and leading member of the search and information retrieval community, serving as the Chair of the Executive Committee for the ACM Special Interest Group on Information Retrieval from 2013 to 2016.
Cost Modeling for Technology-assisted review (27 September, 2021)
Speaker: Eugene Yang
Abstract
Technology-assisted review (TAR) is the most widely-used framework for high recall retrieval problems, such as electronic discovery for legal cases, systematic review for precision medicine, sunshine law requests, etc. It leverages a supervised learning model to iteratively prioritize documents for human experts to review for reducing the reviewing cost by minimizing the amount of non-relevant documents presented to the expert. However, evaluation in the past focused on the effectiveness of the underlying model instead of the overall cost. This talk will discuss a novel cost modeling framework for TAR that provides more insights into TAR operational decisions and creates opportunities for future deployment.
Bio
Eugene Yang is currently a research associate at the Human Language Technology Center of Excellence at Johns Hopkins University. He received his Ph.D. from Georgetown University under the advice of Ophir Frieder and David D. Lewis. His dissertation focuses on advancing the state-of-the-art technology-assisted review from multiple aspects, including cost modeling and stopping rule. Before joining Georgetown, he studied quantitative finance and was a front-end engineering in Taiwan.
Constructing personal knowledge bases for search (20 September, 2021)
Speaker: Andrew Yates
Personal knowledge bases (PKBs) are reusable resources containing user traits that can be used to personalize downstream applications like search and recommender systems. In contrast with latent user representations generated and stored by a remote system, personal knowledge bases can give users control over the data used for personalization. In this talk, I will describe our work constructing personal knowledge bases from challenging data sources and exploring how they can be used to improve search results. The former part of the talk will focus on the task of identifying long-tail attribute values like uncommon professions or hobbies in a zero-shot setting, while the latter will explore what kinds of user profiles are useful and how they can be leveraged. I will conclude with a discussion of open challenges and future work.
"What can I cook with these ingredients?" - Conversational Search in the cooking domain (28 June, 2021)
Speaker: Alexander Frummet
Abstract:
As conversational search becomes more pervasive, it becomes increasingly important to understand the user's underlying information needs when they converse with such systems in diverse domains. We conduct an in-situ study to understand information needs arising in a home cooking context as well as how they are verbally communicated to an assistant. A human experimenter plays this role in our study. Based on the transcriptions of utterances, we derive a detailed hierarchical taxonomy of diverse information needs occurring in this context, which require different levels of assistance to be solved. Current research on how assistance can be provided will be described in the talk.
Bio:
Alexander Frummet is a lecturer and PhD student at the Chair of Information Science in Regensburg, Germany. From 2013 to 2018 he studied General and Comparative Linguistics, Information Science (both Bachelor’s Degree) and Media Informatics (Master’s Degree) at the University of Regensburg.
Towards more practical complex question answering (21 June, 2021)
Speaker: Chen Zhao
Question answering is one of the most important and challenging tasks for understanding human language. With the help of large scale benchmarks, state-of-the-art neural methods have made significant progress to even answer complex questions that require multiple evidence pieces. Nevertheless, training existing SOTA models requires several assumptions (e.g., intermediate evidence annotation, corpus semi-structure) that limit the applicability to only academic testbeds. In this talk, I discuss several solutions to make current QA systems more practical.
I first describe a state-of-the-art system for complex QA with an extra hop attention in its layers to aggregate different pieces of evidence following the structure. Then I introduce a dense retrieval approach that iteratively forms an evidence chain through beam search in dense representations, without using semi-structured information. Finally I describe a dense retrieval work that focuses on a weakly-supervised setting, by learning to find evidence from a large corpus, and relying only on distant supervision for model training.
Bio:
Chen Zhao is a fifth-year phd candidate at university of Maryland, College Park, advised by Jordan Boyd-Graber and Hal Daume III. His research interests lie in question answering, including knowledge representation from large text corpora for complex QA, and semantic paring over tables.
Natural Language Processing with Less Data and More Structures (14 June, 2021)
Speaker: Diyi Yang
Abstract:
Recently, natural language processing (NLP) has had increasing success and produced extensive industrial applications. Despite being sufficient to enable these applications, current NLP systems often ignore the structures of language and heavily rely on massive labeled data. In this talk, we take a closer look at the interplay between language structures and computational methods via two lines of work. The first one studies how to incorporate linguistically-informed relations between different training data to help both text classification and sequence labeling tasks when annotated data is limited. The second part demonstrates how various structures in conversations can be utilized to generate better dialog summaries for everyday interaction.
Bio:
Diyi Yang is an assistant professor in the School of Interactive Computing at Georgia Tech, also affiliated with the Machine Learning Center (ML@GT) at Georgia Tech. She is broadly interested in Computational Social Science, and Natural Language Processing. Diyi received her PhD from the Language Technologies Institute at Carnegie Mellon University. Her work has been published at leading NLP/HCI conferences, and also resulted in multiple awards or nominations from EMNLP 2015, ICWSM 2016, SIGCHI 2019, CSCW 2020, and SIGCHI 2021. She is named as a Forbes 30 under 30 in Science, a recipient of IEEE AI 10 to Watch, and has received faculty research awards from Amazon, Facebook, JPMorgan Chase, and Salesforce.
Progress in the Breadth: Broadening the Scope of Language Understanding (24 May, 2021)
Speaker: Daniel Khashabi
Abstract:
Despite remarkable progress in building models for challenge benchmarks, the scope of progress remains limited to niche datasets (rather than a broad spectrum of language understanding tasks). How can we expand the scope of the abilities of our models?
In this talk, I discuss two instances of modeling approaches to enabling systems that address a broader range of problems. In the first part, I introduce UnifiedQA, a single model that generalizes to multiple different QA formats (multiple-choice QA, extractive QA, abstractive QA, yes-no QA). In the second part, I discuss a paradigm that enables models to generalize across a variety of tasks (not just QA) by leveraging natural language "instructions" of each task. For both works, I present empirical evidence on systems' better generalization across datasets and domains.
Based on the following works:
- UnifiedQA: Crossing Format Boundaries With a Single QA System
- Natural Instructions: Benchmarking Generalization to New Tasks from Natural Language Instructions
Bio:
Daniel Khashabi is a “Young Investigator” at Allen Institute for AI, Seattle. His interests lie at the intersection of artificial intelligence and natural language processing. He earned his PhD from the University of Pennsylvania and his undergraduate degree from Amirkabir University of Technology (Tehran Polytechnic).
Contextualized Neural Retrieval Models: From Effective to Efficiency (17 May, 2021)
Speaker: Ben He
Evaluating and Improving Neural Models for Ranking Responses in Information-Seeking Conversations (10 May, 2021)
Speaker: Gustavo Penha
Abstract:
In this talk I will present an overview of techniques to improve neural rankers in the field of conversational search. Specifically, I will dive into the task of ranking responses in information-seeking dialogues. I will introduce our efforts in building a dataset (MANTiS) for the repeatable and offline evaluation of neural rankers. After that I will present a baseline model for the task followed by techniques to train better models by modifying different parts of the baseline: ordering of the training examples, negative sampling procedures, representation learning, labels and loss functions and handling uncertainty in the predictions. I will finish by discussing possible future research directions in the field of conversational search and recommendation.
Bio:
Gustavo Penha is a PhD candidate at TU Delft, supervised by Claudia Hauff working in the fields of IR, NLP and ML. His recent research is focused on neural ranking models for conversational search and recommendation.
Do People and Neural Nets Pay Attention to the Same Words? Studying Eye-tracking Data for Non-factoid QA Evaluation (26 April, 2021)
Speaker: Baranova Valeriia
Abstract:
We investigated how users evaluate passage-length answers for non-factoid questions. We conduct a study where answers were presented to users, sometimes shown with automatic word highlighting. Users were tasked with evaluating answer quality, correctness, completeness, and conciseness. Words in the answer were also annotated, both explicitly through user mark up and implicitly through user gaze data obtained from eye-tracking. Our results show that the correctness of an answer strongly depends on its completeness, conciseness is less important. Analysis of the annotated words showed correct and incorrect answers were assessed differently. Automatic highlighting helped users to evaluate answers quicker while maintaining accuracy, particularly when highlighting was similar to annotation. We fine-tuned a BERT model on a non-factoid QA task to examine if the model attends to words similar to those annotated. Similarity was found, consequently, we propose a method to exploit the BERT attention map to generate suggestions that simulate eye gaze during user evaluation.
Bio:
Baranova Valeriia, PhD candidate at RMIT University (supervised by Mark Sanderson, Falk Scholer and Bruce Croft). The former head of research and development NLP department at Tinkoff Bank.
Understanding Dynamic User Intention in Personalized Recommendation (19 April, 2021)
Speaker: Prof. Min Zhang
Abstract:
User intention is an essential factor to be considered for recommender systems. Beyond inherent user preference addressed in traditional recommendation algorithms, dynamic user intention is paid more attention to in recent years. However, user intention modeling is non-trivial due to the complex context. This talk will summarize our recent progress on capturing dynamic user intention in sequential recommendation with knowledge-aware temporal dynamic models. A benchmarking platform for sequential recommendation named ReChorus will also be introduced. Comparative experiments demonstrate the proposed methods achieve remarkable improvements in real-world recommendation datasets. Related researches have been published on WWW’19, SIGIR’20, and TOIS in 2021.
Bio:
Dr. Min Zhang is a tenured associate professor in the Department of Computer Science & Technology (DCST), Tsinghua University, and is the vice director of the AI lab, DCST. She specializes in Web search, personalized recommendation, and user modeling. She also serves as Editor-in-Chief of ACM Transaction on Information Systems (TOIS), and ACM SIGIR Executive Committee member, and PC chair or Area Chairs of top conferences. Her work receives more than 4000 citations in recent five years. She was awarded IBM Global Faculty Award 2020, etc. She also owns 12 patents and cooperates with a lot of international and domestic enterprises.
Document re-ranking and entity set expansion (12 April, 2021)
Speaker: Prof. Ben He
What Does Conversational Information Access Exactly Mean and How to Evaluate It? (22 March, 2021)
Speaker: Prof. Krisztian Balog
Towards Mixed-Initiative Conversational Information Seeking (15 March, 2021)
Speaker: Prof. Hamed Zamani
Abstract:
While conversational information seeking has roots in early information retrieval research, recent advances in automatic speech recognition and conversational agents, as well as popularity of devices with limited bandwidth interfaces, have led to increasing interest in this area. An ideal conversational search system requires to go beyond the typical "query-response" paradigm by supporting mixed-initiative interactions. In this talk, I will review the recent efforts on developing mixed-initiative conversational search systems and draw connections with early work on interactive information retrieval. I will describe methods for generating and evaluating clarifying questions in response to search queries. I will further highlight the connections between conversational search and recommendation, and finish with a discussion on the next steps that require significant progress in the context of mixed-initiative conversational search.
Bio:
Hamed Zamani is an Assistant Professor in the College of Information and Computer Sciences at the University of Massachusetts Amherst (UMass), where he also serves as the Associate Director of the Center for Intelligent Information Retrieval (CIIR). His research focuses on developing and evaluating statistical and machine learning models with application to (interactive) information access systems including search engines, recommender systems, and question answering. His current projects are related to neural information retrieval, weakly supervised deep learning, and conversational information seeking. Prior to UMass, Hamed was a Researcher at Microsoft. In 2019, he received his Ph.D. from UMass under supervision of W. Bruce Croft. He obtained his M.Sc. and B.Sc. degrees from University of Tehran.
Entity Linking in Documents and Conversation (08 March, 2021)
Speaker: Prof. Faegheh Hasibi
FA*IR: A fair top-k ranking algorithm for multiple protected groups (01 March, 2021)
Speaker: Meike Zehlike
information retrieval and deep reinforcement learning (22 February, 2021)
Speaker: Prof. Grace Hui Yang
Generating music in the raw audio domain (18 February, 2021)
Speaker: Sander Dieleman
Realistic music generation is a challenging task. When machine learning is used to build generative models of music, typically high-level representations such as scores, piano rolls or MIDI sequences are used that abstract away the idiosyncrasies of a particular performance. But these nuances are very important for our perception of musicality and realism, so we embark on modelling music in the raw audio domain. I will discuss some of the advantages and disadvantages of this approach, and the challenges it entails.
Biomedical Knowledge-Enhanced Language Modeling (15 February, 2021)
Speaker: Dr. Zaiqiao Meng
Abstract:
Pretraining large neural language models, such as BERT, has led to impressive gains on many natural language processing (NLP) tasks. However, most pretraining efforts focus on general domain corpora, such as newswire and Web, while learning text representations to accurately capture complex and fine-grained semantic relationships in the biomedical domain remains as a challenge. Addressing this is of paramount importance for tasks such as entity linking where complex relational knowledge is pivotal. In this talk, I will provide an overview of how to incorporate diverse knowledge into a pre-training model such as BERT. In particular, I will detail SAPBERT, a pre-training scheme based on BERT, which self-aligns the representation space of biomedical entities with a metric learning objective function leveraging UMLS, a collection of biomedical ontologies with >4M concepts.
Bio:
Zaiqiao is currently a Research Associate at the Language Technology Laboratory (LTL) of the University of Cambridge. Before that, he was a Research Assistant at the Terrier team of the University of Glasgow. Zaiqiao's research interests include Graph Neural Networks, Knowledge Graphs, Recommender Systems, and Natural Language Processing.
Smart Factory INDU-ZERO (03 February, 2021)
Speaker: Karl Preiss, Scott Brady
Summary:
NMIS is working on the initial feasibility development of a new 5-hectare smart factory concept for off-site automated home thermal renovation façade systems for local government authorities and housing associations in the UK and around the EU. North Sea Region as part of a multi-nation cross-functional consortium. The renovation kit designs consist of several Sandwich panels for walls and roofs made from standard EPS blocks, CNC milled, then skinned, sealed, decorated and window and door apertures lined, then fully assembled ready for building onto a complete house unit on-site. In this project, the focus of NMIS is to design the blueprint of the factory in 2D and in 3D and simulate key process stages for discrete event, visual material flow, and robotic analysis. This also involves assessing product-process feasibility and design for manufacture/assembly. Flexible, smart automation is a key high-level deliverable, and we are considering various options to help achieve this. At this stage, it’s only a research project, but we are hoping to use our developed 3D factory model for communication purposes and to attract additional funding from industrial investors.
The volume of the proposed factory is intended to clad 15,000 homes per year, so potentially 90,000 individual panels. To meet the Paris Agreement requirements for the EU region we are covering, over 40 similar factories could be required, built to the same blueprint. So there is much potential for growth in off-site manufacture of these 2D + 2.5D panels.
Links to the project:
https://www.strath.ac.uk/research/advancedformingresearchcentre/news/indu-zero/
https://northsearegion.eu/indu-zero/about-the-project/
Zoom link: https://uofglasgow.zoom.us/j/93570332919?pwd=VktWb1RLTHkyWURDSndVdksyNDdLQT09 (Passcode: 516455)
Speaker: Karl Preiss, Scott Brady
When: 1:00 pm – 2:00 pm, Wednesday, 3rd February
Where: https://uofglasgow.zoom.us/j/93570332919?pwd=VktWb1RLTHkyWURDSndVdksyNDdLQT09 (Passcode: 516455)
Summary:
NMIS is working on the initial feasibility development of a new 5-hectare smart factory concept for off-site automated home thermal renovation façade systems for local government authorities and housing associations in the UK and around the EU. North Sea Region as part of a multi-nation cross-functional consortium. The renovation kit designs consist of several Sandwich panels for walls and roofs made from standard EPS blocks, CNC milled, then skinned, sealed, decorated and window and door apertures lined, then fully assembled ready for building onto a complete house unit on-site. In this project, the focus of NMIS is to design the blueprint of the factory in 2D and in 3D and simulate key process stages for discrete event, visual material flow, and robotic analysis. This also involves assessing product-process feasibility and design for manufacture/assembly. Flexible, smart automation is a key high-level deliverable, and we are considering various options to help achieve this. At this stage, it’s only a research project, but we are hoping to use our developed 3D factory model for communication purposes and to attract additional funding from industrial investors.
The volume of the proposed factory is intended to clad 15,000 homes per year, so potentially 90,000 individual panels. To meet the Paris Agreement requirements for the EU region we are covering, over 40 similar factories could be required, built to the same blueprint. So there is much potential for growth in off-site manufacture of these 2D + 2.5D panels.
Links to the project:
https://www.strath.ac.uk/research/advancedformingresearchcentre/news/indu-zero/
https://northsearegion.eu/indu-zero/about-the-project/
Building start-ups, with Stewart Whiting (01 February, 2021)
Speaker: Dr. Stewart Whiting
Abstract:
Startups are exciting. If you enjoy sleepless nights, endless stress and a torturous learning curve with little chance of success - but with a potential for huge impact and financial upside – then startups are for you! There will never be a better time to start than now.
A lot of startup talks are founders telling their own story, or abstract ‘how to do a tech startup’ bullet points. The truth is, there is no recipe and every startup is completely different. Instead, I’ll talk through some pragmatic tips I think could be helpful for uni students/academics thinking about starting their journey, and I’ll add a few stories from my experiences along the way.
Bio:
I started my undergrad in business and comp sci at Glasgow in 2006 and continued on to a PhD in IR which I completed in 2015. I knew I was a useless academic, so my future was in industry. I interned twice at MSR in Silicon Valley, working on applied research. While writing up, I was quietly working on a few different startup ideas because I wanted to stay in Scotland but didn’t see any companies where I could work on tech that interested me.
I ended up co-founding SNAP40, now known as Current Health. Our mission is simple: we’re helping healthcare transition from hospital to home. Our full-service remote monitoring product allows healthcare professionals and clinical trial teams to spot high-risk patients who are getting sick at home and intervene rapidly. The last year has been mad. We’re now at ~80 full-time employees, operating in 4 continents and have raised $25M+ in investment.
Understanding Product Reviews: Question-Answering and Brand-Sentiment Detection (25 January, 2021)
Speaker: Prof. Yulan He
Abstract:
In this talk, I will present our recent work on analysing product reviews. I will start with a cross-passage hierarchical memory network for generative question-answering on product reviews. It extends XLNet by introducing an auxiliary memory module consisting of two components: the context memory collecting cross-passage evidence, and the answer memory working as a buffer continually refining the generated answers. The proposed architecture outperforms the state-of-the-art baselines with better syntactically well-formed answers and increased precision in addressing questions based on Amazon reviews. I will next present the Brand-Topic Model (BTM) which aims to detect brand-associated polarity-bearing topics from product reviews. BTM is able to automatically infer real-valued brand-associated sentiment scores and generate fine-grained sentiment-topics in which we can observe continuous changes of words under a certain topic while its associated sentiment gradually varies from negative to positive. Experimental results show that BTM outperforms a number of competitive baselines in brand ranking, achieving a better balance of topic coherence and uniqueness, and extracting better-separated polarity-bearing topics.
Short bio:
Yulan He is a Professor at the Department of Computer Science in the University of Warwick, UK. Her research interests lie in the integration of machine learning and natural language processing for text analytics. She has published over 170 papers on topics including sentiment analysis, topic/event extraction, clinical text mining, recommender systems, and spoken dialogue systems. She has been the recipient of a CIKM 2020 Test-of-Time Award, AI 2020 Most Influential Scholar Honourable Mention by AMiner, and a Turing AI Acceleration Fellowship. She was a Program Co-Chair in EMNLP 2020. Yulan obtained her PhD degree in spoken language understanding from the University of Cambridge, and her MEng and BASc degrees in Computer Engineering from Nanyang Technological University, Singapore.
Closing the Dequantization Gap: PixelCNN as a Single-Layer Flow (21 January, 2021)
Speaker: Ole Winther
https://uofglasgow.zoom.us/j/91703102253?pwd=QnlYYWJyVWVoanFJTk5nNFJ4Tms4UT09
Flow models have recently made great progress at modeling ordinal discrete data such as images and audio. Due to the continuous nature of flow models, dequantization is typically applied when using them for such discrete data, resulting in lower bound estimates of the likelihood. In this paper, we introduce subset flows, a class of flows that can tractably transform finite volumes and thus allow exact computation of likelihoods for discrete data. Based on subset flows, we identify ordinal discrete autoregressive models, including WaveNets, PixelCNNs and Transformers, as single-layer flows. We use the flow formulation to compare models trained and evaluated with either the exact likelihood or its dequantization lower bound. Finally, we study multilayer flows composed of PixelCNNs and non-autoregressive coupling layers and demonstrate state-of-the-art results on CIFAR-10 for flow models trained with dequantization.
Using geometry to form identifiable latent variable models and Isometric Gaussian Process Latent Variable Model (03 December, 2020)
Speaker: Søren Hauberg & Martin Jørgensen
Please note that the timeslot has changed to 12:00-13:30.
There will be two talks in this session:
-----------------------------------------------------------
12:00-13:00 Using geometry to form identifiable latent variable models - Prof Søren Hauberg
Generative models learn a compressed representation of data that is often used for downstream tasks such as interpretation, visualization and prediction via transfer learning. Unfortunately, the learned representations are generally not statistically identifiable, leading to a high risk of arbitrariness in the downstream tasks. We propose to use differential geometry to construct representations that are invariant to reparametrizations, thereby solving the bulk of the identifiability problem. We demonstrate that the approach is deeply tied to the uncertainty of the representation and that practical applications require high-quality uncertainty quantification. With the identifiability problem solved, we show how to construct better priors for generative models, and that the identifiable representations reveal signals in the data that were otherwise hidden.
----------------------------------------------------------
13:00-13:30: Isometric Gaussian Process Latent Variable Model - Martin Jørgensen, Postdoc
I present a generative unsupervised model where the latent variable respects both the distances and the topology of the modeled data. The model leverages the Riemannian geometry of the generated manifold to endow the latent space with a well-defined stochastic distance measure, which is modeled as Nakagami distributions. These stochastic distances are sought to be as similar as possible to observed distances along a neighborhood graph through a censoring process. The model is inferred by variational inference. I demonstrate how the model can encode invariances in the learned manifolds.
-----------------------------------------------------------
Zoom link: https://uofglasgow.zoom.us/j/95874104571?pwd=Rjc0VERQR25ReHRSSzRweUtEUlYvUT09
Soft Squishy Electronic Skin (23 November, 2020)
Speaker: Ravinder Dahiya
https://uofglasgow.zoom.us/j/92128593434?pwd=enhTc2xvKyt5Njd5MDU3K1p0ZkFDdz09
The miniaturization led advances in microelectronics over 50 years have revolutionized our lives through fast computing and communication. Recent advances in the field are propelled by applications such as electronic skin in robotics, wearable systems, and healthcare technologies etc. Often these applications require electronics to be soft and Squishy so as to conform to 3D surfaces. These requirements call for new methods to realize sensors, actuators electronic devices and circuits on unconventional substrates such as plastics, papers and elastomers. This lecture will present various approaches (over different time and dimension scales) for obtaining distributed electronic, sensing and actuation devices on soft and flexible substrates, especially in context with the tactile or electronic skin (eSkin). These approaches range from distributed off-the-shelf electronics integrated on flexible printed circuit boards, to novel alternatives such as eSkin constituents obtained by printed nanowires, graphene and ultra-thin chips, etc. The technology behind such sensitive flexible and squishy electronic systems is also the key enabler for numerous emerging fields such as internet of things, smart cities and mobile health etc. This lecture will also discuss how the flexible electronics research may unfold in the future.
Bayesian model-based clustering in high dimensions (19 November, 2020)
Speaker: Paul Kirk
https://uofglasgow.zoom.us/j/92184721880?pwd=dExzeDhxU3h6RnplYlg1UkoxY3RjZz09
Although the challenges presented by high dimensional data in the context of regression are well-known and the subject of much current research, comparatively little work has been done on this in the context of clustering. In this setting, the key challenge is that often only a small subset of the features provides a relevant stratification of the population. Identifying relevant strata can be particularly challenging when dealing with high-dimensional datasets, in which there may be many features that provide no information whatsoever about population structure, or -- perhaps worse -- in which there may be (potentially large) feature subsets that define irrelevant stratifications. For example, when dealing with genetic data, there may be some genetic variants that allow us to group patients in terms of disease risk, but others that would provide completely irrelevant stratifications (e.g. which would group patients together on the basis of eye or hair colour). Bayesian profile regression is an outcome-guided model-based clustering approach that makes use of a response in order to guide the clustering toward relevant stratifications. Here we consider how this approach can be extended to the “multiview” setting, in which different groups of features (“views”) define different stratifications. We present some results in the context of breast cancer subtyping to illustrate how the approach can be used to perform integrative clustering of multiple ‘omics datasets.
Adaptive Pointwise-Pairwise Learning-to-Rank for Content-based Recommendation and Period-aware Content-based Attention for Time Series Forecasting (02 November, 2020)
Speaker: Yagmur Gizem Cinar
Abstract:
In this talk, she will present (i) adaptive pointwise-pairwise learning-to-rank for content-based recommendation and (ii) period-aware content-based attention for time series forecasting.
(i) Ranking is widely used in information retrieval, recommendation and natural language processing applications. When designing search or recommender systems and, especially, “learning to rank” strategies, one typically faces the issue of choosing which one of the “pointwise”, “pairwise” and “listwise” approaches should be adopted. Each approach has its own advantages and drawbacks. They focus on the pointwise and pairwise approaches, and extend the standard pointwise and pairwise paradigms for learning-to-rank in the context of personalized recommendation, by considering these two approaches as two extremes of a continuum of possible strategies. It consists of a surrogate loss, adaptive pointwise-pairwise learning-to-rank loss, that models how to select and combine these two approaches adaptively, depending on the context (query or user, pair of items, etc.)
(ii) Recurrent neural networks (RNNs) recently received considerable attention for sequence modelling and time series analysis. Many time series contain periods, e.g. seasonal changes in weather time series or electricity usage at day and night time. Here, they first analyze the behaviour of RNNs with an attention mechanism with respect to periods in time series. Period-aware attention mechanism for sequence-to-sequence RNNs is designed to capture periods in time series with or without missing values.
Short Bio: Yagmur Gizem Cinar is a postdoctoral researcher at Laboratoire d’Informatique de Grenoble, Univ. Grenoble Alpes, Grenoble, France. She was a postdoctoral research scientist at Naver Labs Europe, France (2018-2020). She received her PhD on Sequence Prediction with Recurrent Neural Networks in the Context of Time Series and Information Retrieval Search Sessions at Univ. Grenoble Alpes in 2019, and MSc degrees in Artificial Intelligence and Electrical Engineering at KU Leuven in 2015 and 2014, respectively. Her research interests are machine learning, deep sequential learning and multi-modal learning.
zoom passcode: 290551
Dark Data: Why What You Don’t Know Matters (02 November, 2020)
Speaker: David J. Hand
Dark data are data you don’t have. It might be that you want today’s data, but all you have is yesterday’s. It might be that certain types of cases are missing from your sample. It might be that the recorded values are inaccurate – no measuring instrument is perfect. It might be that the process of collecting the data changes those very data themselves. It might be that you have only summary values, like averages, which tell you nothing about extremes. Or it might be data that has been collected and stored but not analysed – perhaps they were collected for regulatory compliance reasons. I outline a taxonomy of fifteen types of dark data, showing just how serious the consequences can be. But then I go further, showing strategies for coping with dark data, and even how to take advantage of it in a strategic application of ignorance.
(The book is available in electronic form in the University library, and is worth a read : http://tinyurl.com/yxwtsotq )
A Brief History of Deep Learning applied to Information Retrieval: A Personal Perspective (19 October, 2020)
Speaker: Rodrigo Nogueira
Abstract: In the past two years, we have seen remarkable progress in the development of information retrieval systems. Behind this ongoing revolution are pre-trained deep learning models, whose initial success in natural language processing promptly sparked interest in the information retrieval community. In this talk, I will discuss my journey of applying deep learning to information retrieval, from a naive start of developing navigational methods, passing by spectacular failures in using reinforcement learning in query reformulation, to finally succeed with pre-trained language models applied to multi-stage ranking and document expansion.
Short Bio: Rodrigo Nogueira is a post-doctoral researcher at the University of Waterloo (Canada), an adjunct professor at UNICAMP (Brazil), and a senior research scientist at NeuralMind (Brazil). He holds a Ph.D. from New York University (NYU), where he worked on at the intersection of Deep Learning, Natural Language Processing, and Information Retrieval under the supervision of prof. Kyunghyun Cho. He has an Ms.C. degree from UNICAMP, where he developed with prof. Roberto Alencar Lotufo an award-winning algorithm for detecting fake fingerprints.
zoom passcode: 420597
Towards Human-Robot collaboration (30 September, 2020)
Speaker: Dr Sebastian Stein
Abstract:
Intelligent agents are capable of learning to make decisions and act in increasingly complex environments. This trend continues in multi-agent scenarios, where populations of agents learn to coordinate their actions towards a common goal, and in safety-critical systems, where hard constraints limit exploration. While intelligent systems have been found to be particularly useful when they support instead of replace humans, human-robot collaboration is currently under-explored.
In this talk, I approach human-robot collaboration from two extremes of the collaborative continuum: intelligent user interfaces and autonomous reinforcement learning agents. In exploring 'the space in between' one encounters questions such as How do we design intuitive interfaces to control agents' level of autonomy? How do agents learn to become increasingly autonomous over time? What internal model adequately represents collaborative tasks? How do we communicate goals and how do agents process implicit feedback? I will sketch a research plan to address some of these questions and I am keen to discuss this with the audience.
--------------------------------------------------------------------------------
Here is the link to the group meeting of Wednesday:
https://uofglasgow.zoom.us/j/91550064658
--------------------------------------------------------------------------------
We are looking forward to seeing you all on Wednesday and we hope that you will have a nice day!
Using an Inverted Index Synopsis for Query Latency and Performance Prediction (28 September, 2020)
Speaker: Nicola Tonellotto
In this talk I will discuss how index synopses – which are stochastic samples of the full index – can be used for attaining accurate timings. Experiments using the TREC ClueWeb09 collection, and a large set of user queries, show that using a small random sample it is possible to very accurately estimate properties of the larger index, including sizes of posting list unions and intersections. I will also show that index synopses facilitate two use cases: (i) predicting the query latencies on the full index and classifying long-running queries can be accurately achieved using index synopses; (ii) the effectiveness of queries can be estimated more accurately using a synopsis index post-retrieval predictor than a pre-retrieval predictor. This work is partially supported by the Italian Ministry of Education and Research (MIUR) in the framework of the CrossLab project (Departments of Excellence).
Short Bio: Dr. Nicola Tonellotto (male) is assistant professor at the Information Engineering Department of the University of Pisa since 2019. From 2002 to 2019 he was researcher at the Information Science and Technologies Institute “A. Faedo” of the National Research Council of Italy. His main research interests include Cloud Computing, Web Search, Information Retrieval and Deep Learning. He co-authored more than 60 papers on these topics in peer reviewed international journal and conferences. He participated and coordinated activities in several European projects such as CoreGRID, NextGRID, GRIDComp, S-Cube, MIDAS, BigDataGrapes. He is co-recipient of the ACM SIGIR 2015 Best Paper Award. He taught or teaches BSc, MSc and PhD courses on Cloud computing, distributed enabling platforms and information retrieval.
Reinforcement Learning @Huawei R&D London – Towards real-world autonomous decision making (10 August, 2020)
Speaker: Haitham Ammar
Abstract: Reinforcement learning (RL) is a technique that enables sequential decision making via autonomous agents. Applications of such a field are ubiquitous in Huawei ranging from self-driving cars to 5G wireless networks and data-centre cooling systems. Though successful in solving game-like environments, current methods for reinforcement learning are hard to apply in the real world due to their inefficient learning process, unsafe exploration strategies, and non-robust deployable models. In this talk, we will survey SOTA solutions to RL and demonstrate our contributions in tackling the above problems to enable the next generation of useful learners. We demonstrate that through principled frameworks that combine probabilistic modelling, numerical optimisation and game-theory, one can reduce sample complexity by orders of magnitude, enable safe learning processes, and improve robustness when compared to other methods.
I will also detail various collaboration potentials with the team.
https://uofglasgow.zoom.us/j/92128593434?pwd=enhTc2xvKyt5Njd5MDU3K1p0ZkFDdz09
Multiresolution Multitask Gaussian Processes: Air quality in London (27 February, 2020)
Speaker: Theo Damoulas
Date to be confirmed
We consider evidence integration from potentially dependent observation processes under varying spatio-temporal sampling resolutions and noise levels. We offer a multi-resolution multi-task framework, termed MRGPs, while allowing for both inter-task and intra-task multi-resolution and multi-fidelity. We develop shallow Gaussian Process (GP) mixtures that approximate the difficult to estimate joint likelihood with a composite one and deep GP constructions that naturally handle scaling issues and biases. By doing so, we generalize and outperform state of the art GP compositions and offer information-theoretic corrections and efficient variational approximations for inference. We demonstrate the competitiveness of MRGPs on synthetic settings and on the challenging problem of hyper-local estimation of air pollution levels across London from multiple sensing modalities operating at disparate spatio-temporal resolutions.
IR Seminar: Applied Research in Cross-functional Product Development (24 February, 2020)
Speaker: James Brill
Signal AI is an AI-powered platform for media monitoring and intelligence. In Signal AI, we as data scientists, actively apply research in a variety of different fields, such as entity classification, text clustering, and news ranking to develop our platform.
To do that, we work as a part of a cross-functional product teams, each focusing on specific user problems. Applying research in this environment comes with unique challenges. Firstly, product development is a fast moving environment which reduces our ability to exhaustively explore the problem space. Secondly, it is hard to understand the value to the user using theoretical quality metrics such as precision & recall. Finally, deploying a new solution, e.g. a new clustering algorithm, into a production environment comes with operational complexities such as cost, latency, and efficiency. In this talk, I will give examples of these challenges from my work at Signal AI, and explain how they can be mitigated by pragmatic, experimental-driven and people-orientated decision making principles.
Speaker Bio
IR Seminar: Quantum-inspiration for User Behaviour Modelling in Information Interaction and Beyond (17 February, 2020)
Speaker: Sagar Uprety
Abstract:
The fields of cognitive and decision sciences are concerned with building mathematical models to explain and predict human decisions. Most of these models are based on set-theoretic logic and probability axioms. However, there are a large number of studies in these fields which show that human behaviour violates these axioms. Such puzzling findings (e.g. preference reversal, conjunction/disjunction fallacy, disjunction effect, prisoner’s dilemma, order effects, etc.) have been deemed as cases of “irrational behaviour”. The emerging field of Quantum Cognition posits that the underlying mathematical structures of Quantum Theory (QT) provides a better account of human decisions than the traditional models. Quantum probability theory is more general than the classical probability theory and has been successful in explaining and modelling some of the aforementioned violations of rational decision theory.
In this presentation I will begin with the motivation behind using QT to explain and model human decisions. I will talk about the fundamental principles of QT which are analogous to certain cognitive mechanisms and give some examples of Quantum-inspired models. Also discussed will be recent work done in applying QT to user behaviour analysis in IR. I will conclude with the potential applications of Quantum Cognition in modelling user decisions in the online world.
Bio:
Sagar Uprety is a Marie Curie Researcher in the project QUARTZ and a final year PhD student at the Open University. His research focusses on investigating quantum-like phenomena in user behaviour in IR and building quantum-inspired user models. He has degrees in Computer Science and Physics and has worked as a software developer for a vertical search engine and as a machine learning engineer for a local search engine in India, building voice search products and chatbots. His broad research interests are in User Modelling , Quantum Cognition, Information Interaction and Behavioural Data Science.
Advanced Machine Learning Reading Group (12 February, 2020)
Speaker: Salman Mohammadi
We will have our next session tomorrow, Wednesday at 14:00 in SAWB 203. Salman will continue with variational inference, picking up where Valentin left off.
IR Seminar: Modelling user interaction utilising Information Foraging Theory (27 January, 2020)
Speaker: Ingo Frommholz
Abstract
System-oriented information retrieval has traditionally been dealing with scoring functions to compute a probability of relevance of a document with respect to a query. On the other hand, user-oriented IR has been dealing with aspects of information behaviour and user interaction. While there have been recent efforts to combine both aspects of IR, still models that integrate and formally describe user aspects are missing. In this talk, I will present some research that utilises Information Foraging theory (IFT) to create a model for advanced user interaction with the system. We will discuss how IFT can inform such a model by applying it in an image search scenario. My talk will further look at some ideas of how to integrate continued user interaction in a formal mathematical framework inspired by quantum theory.
Speaker Bio
Ingo Frommholz is a senior lecturer in computer science at the School of Computer Science and Technology of the University of Bedfordshire in Luton. He received his PhD in 2008 at the University of Duisburg-Essen (Germany) on the topic of probabilistic logic-based information retrieval models. His current research focuses on information retrieval and digital libraries aspects, in particular, formal IR models based on probabilistic logics and quantum probabilities for interactive IR. He is also Bedfordshire's PI of the Horizon 2020 Marie Skłodowska-Curie European Training Network QUARTZ (Quantum Information Access and Retrieval Theory) to adopt a novel approach to IR based on the quantum mechanical framework.
Artificial Intelligence for Data Analytics (23 January, 2020)
Speaker: Chris Williams
The practical work of deploying a machine learning system is
dominated by issues outside of training a model: data preparation,
data cleaning, understanding the data set, debugging models, and so
on. The goal of the Artificial Intelligence for Data Analytics project
at the Alan Turing Institute is to help to automate the whole data
analytics process by drawing on advances in AI and machine learning.
We will describe tools to address such tasks, including identifying
syntactic and semantic data types, data integration, and identifying
and repairing missing and anomalous data.
Joint work with the AIDA team: Taha Ceritli, James Geddes, Ernesto
Jimenez-Ruiz, Ian Horrocks, Alfredo Nazabal, Tomas Petricek, Charles
Sutton, Gerrit Van Den Burg.
IR Seminar: Crowdsourcing and evaluating text quality (20 January, 2020)
Speaker: David Howcroft
bstract
Over the last decade, crowdsourcing has become a standard method for collecting training data for NLP tasks and evaluating NLP systems for things like text quality. Many evaluations, however, are still ill-defined.
In the practical portion of this talk I present an overview of current tasks addressed with crowdsourcing in computational linguistics, along with tools for implementing them. This overview is meant to be interactive: I am sharing some of the best or most interesting tasks I am aware of, but I would like us to have a conversation about how *you* are using crowdsourcing as well.
After this discussion of tasks, tools, and best practices, I introduce a new research program from the Heriot-Watt NLP Lab looking at human and automatic evaluations for natural language generation. This includes foundational work to make our evaluations more well-defined, experimental work developing new reading time measures to assess readability, and modeling work as we seek new methods of quality estimation that improve upon metrics like BLEU and BERTscore.
Speaker Bio
Dave Howcroft is a computational linguist interested in linguistic complexity and natural language generation. Currently focused on evaluation methods for natural language generation, he joined the Interaction Lab at Heriot-Watt University's School of Mathematics and Computer Sciences as a research associate in June 2019, coming from Saarland University in Germany.
Disentangled representation learning in healthcare applications (20 January, 2020)
Speaker: Sotirios A Tsaftaris
Prof. Sotirios A Tsaftaris
Canon Medical/Royal Academy of Engineering Research Chair in Healthcare AI Chair in Machine Learning and Computer Vision at the University of Edinburgh (UK)
Turing Fellow Alan Turing Institute
Abstract: The detection of disease, segmentation of anatomy and other classical image analysis tasks, have seen incredible improvements due to deep learning. Yet these advances need lots of data: for every new task, new imaging scan, new hospital, more training data are needed. In this talk, I will show how deep neural networks can learn latent and disentangled embeddings suitable for several analysis tasks. Within a multi-task learning setting I will show that the same framework can learn embeddings drawing supervision from self-supervised tasks that use reconstruction and also temporal dynamics, and weakly supervised tasks obtaining supervision from health records [1,2]. I will then present an extension of this framework on multi-modal (multi-view) learning and inference [3]. I will then discuss how different architectural choices affect disentanglement [3] and highlight issues that raise the need for (new) metrics for assessing disentanglement in content/style disentanglement settings. Time permitting, I will present a challenging auto-regressive task: learning to age the human brain [4]. I will conclude by highlighting challenges for deep learning in healthcare in general.
Papers that will be discussed (in approximate order):
- A. Chartsias, T. Joyce, G. Papanastasiou, S. Semple, M. Williams, D. Newby, R. Dharmakumar, S.A. Tsaftaris, 'Disentangled Representation Learning in Cardiac Image Analysis,' Medical Image Analysis, Vol 58, Dec 2019 https://arxiv.org/abs/1903.09467
- G. Valvano, A. Chartsias, A. Leo, S.A. Tsaftaris, 'Temporal Consistency Objectives Regularize the Learning of Disentangled Representations,' First MICCAI Workshop, DART 2019, in Conjunction with MICCAI 2019, Shenzhen, China, October 13 and 17, 2019. https://arxiv.org/abs/1908.11330
- A. Chartsias, G. Papanastasiou, C. Wang, S. Semple, D. Newby, R. Dharmakumar, S.A. Tsaftaris, Disentangle, align and fuse for multimodal and zero-shot image segmentation,' https://arxiv.org/abs/1911.04417
- T. Xia, A. Chartsias, S.A. Tsaftaris, 'Consistent Brain Ageing Synthesis,' MICCAI 2019. http://tsaftaris.com/preprints/Tian_MICCAI_2019.pdf
Bio [Long]: Prof. Sotirios A. Tsaftaris, obtained his PhD and MSc degrees in Electrical Engineering and Computer Science (EECS) from Northwestern University, USA in 2006 and 2003 respectively. He obtained his Diploma in Electrical and Computer Engineering from the Aristotle University of Thessaloniki, Greece.
Currently, he is Canon Medical/Royal Academy of Engineering Research Chair in Healthcare AI, and Chair in Machine Learning and Computer Vision at the University of Edinburgh (UK). He is also a Turing Fellow with the Alan Turing Institute. Previously he was an Assistant Professor with IMT Institute for Advanced Studies, Lucca, Italy and Director of the Pattern Recognition and Image Analysis Unit at IMT (2011-2015). Prior to that, he held a joint Research Assistant Professor appointment at Northwestern University with the Departments of Electrical Engineering and Computer Science (EECS) and Radiology Feinberg School of Medicine (2006-2011).
Infinitech Cakes Event (14 January, 2020)
Speaker: Information Retrieval Group
The Information Retrieval group will be hosting a cakes morning on Tuesday the 14th of January to celebrate the start of the new Horizon 2020 Infinitech project we are part of. Infinitech is one of the European Commission's Flagship projects (valued at over 15 million euros) and lays the groundwork for future financial service projects in Horizon Europe.
We encourage anyone who is interested in research into financial services and big finance data to come along and join us!
Machine learning for healthcare applications: Becoming the expert (05 December, 2019)
Speaker: Alison O'Neil
Machine learning has shown great promise for healthcare applications, matching human performance for some classes of problem. Meantime, the use of electronic medical records is becoming more common and healthcare technologies and infrastructure are advancing, whilst radiology and many other medical specialties are under-resourced. As a result, there are huge opportunities to use automation and AI to improve workflow and to assist the doctor to make complex decisions faster and more accurately. However, data is often sensitive and difficult to access (especially for rare pathologies), expert annotators are a scarce resource, and high stakes means stringent accuracy requirements. This talk will discuss the challenges - and ways to solve them! - of training real-world expert AI systems for healthcare applications, illustrated through Canon Medical’s AI Research projects in image analysis, natural language processing, and risk stratification from clinical data.
A reinforcement learning based traffic signal control in a connected vehicle environment (29 November, 2019)
Speaker: Sebastian Stein and Saeed Maadi
Understanding where cells move using microscopes, computers and equations. (29 November, 2019)
Speaker: Robert Insall
Throughout our life cycles, the cells in our bodies need to move around. If they need to get anywhere they need to be steered; Random migration is ineffective over longer distances.
Recent research has taught us a great deal about how cells respond to steering cues, but surprisingly little about where those cues come from. Recently, a combination of mathematical modelling, studies in amoebas, and analysis of cancer cells shows that cells frequently make their own gradients, often from sources with no positional information at all, at the same time as they respond to them.
Because this is based around positive feedback loops powered by signalling and diffusion, the results are often unpredictable and frequently fascinating and beautiful. Cells may move in waves, streams, or repel one another into carefully-delineated territories. Furthermore, the process of doing so can make them remarkably better at interpreting their environments than we have ever expected was possible.
I will show examples of cells moving collectively at a distance from one another, solving mazes of different shapes, and the mechanisms that enable cancer cells to spread from tumours into the bloodstream. I will also describe uses of transfer learning to identify mutations in the relevant pathways and propose a difficult inverse problem that Computing Science experts may be able to solve.
IR Seminar: Revisiting Offline Evaluation for Implicit-Feedback Recommender Systems (25 November, 2019)
Speaker: University of Antwerp
Abstract:
Recommender systems often need to be evaluated in an offline setting, through experiments on some historical dataset.
Several recent papers have shown that the robustness and reproducibility of results obtained through such procedures leaves much to be desired.
Furthermore, more often than not, these results do not align with success in an online setting.
Offline experiments are still much more efficient than online deployment, so a clear need arises for effective offline procedures than can accurately predict online performance.
In this talk, I will present some of our work in this regard, on how temporal constraints and presentation bias in datasets can corrupt offline results.
Finally, we will move towards off-policy and counterfactual evaluation, and show how methods from the reinforcement learning world can be applied in recommender system settings.
Bio:
Olivier Jeunen is a 3rd year PhD student at the University of Antwerp, Belgium.
His main line of research is centred around offline evaluation of recommender systems, with a recent focus on causal inference for machine learning, and bandit algorithms for recommendation.
Statistical emulation of cardiac mechanics (08 November, 2019)
Speaker: Dirk Husmeier
In recent years, we have witnessed impressive developments in the mathematical modelling of complex physiological systems. This provides unprecedented novel opportunities for improved disease diagnosis based on an enhanced quantitative physiological understanding. In a recent proof of concept study, we have shown that the biomechanical parameters of a state-of-the-art cardiac mechanics model have encouraging diagnostic power for early diagnosis of the risk to myocardial infarction (heart attack) and decision making related to alternative treatment options. However, estimating the biomechanical parameters non-invasively from magnetic resonance imaging (MRI) is computationally expensive and can take several weeks of high-performance computing time. This constitutes a severe obstacle for translational research, preventing uptake in the clinic and thwarting any pathway to genuine impact in healthcare. The problem is that state-of-the-art mathematical models of complex physiological systems are typically based on systems of nonlinear coupled partial differential equations (PDEs), which have no closed-form solution and have to be integrated numerically, e.g. using finite element simulations. This is not an issue for the so-called forward problem, where the objective is to understand a system’s behaviour for given physiological parameters. However, many physiological parameters cannot be measured noninvasively, and hence have to be estimated indirectly based on a quantitative measure of the discrepancy between model predictions and non-invasive measurements. This calls for thousands of numerical integrations as part of an iterative optimization or sampling routine, incurring computational run times in the order of days or weeks.
A potential way to deal with the high computational complexity and make progress towards a clinical decision support system that can make disease prognostications and risk assessments in real time, is statistical emulation. The idea is to approximate the computationally expensive mathematical model (the simulator) with a computationally cheap statistical surrogate model (the emulator) by a combination of massive parallelization and nonlinear regression. Starting from a space-filling design in parameter space, the underlying partial differential equations are solved numerically on a parallel computer cluster, and methods from nonparametric Bayesian statistics based on Gaussian Processes (GPs) are applied to multivariate smooth interpolation. When new data become available (e.g. myocardial strains from MRI scans) the resulting proxy objective function can be maximized (for maximum likelihood estimation) or sampled from (using Markov chain Monte Carlo) at low computational costs, without further computationally expensive simulations of the original mathematical model.
In my talk, I will compare different emulation strategies and loss functions, and assess the reduction in computational complexity. For large data sets, it is not computationally feasible to train a GP, as the computational complexity is of the order of the third power of the data set size, and I will compare various alternative paradigms for dealing with this issue. I will describe a proof-of-concept study, with encouraging results: While conventional parameter estimation based on numerical simulations from the cardiac mechanics model leads to computational costs in the order of weeks, our emulation method reduces the computational complexity to the order of the quarter of an hour, while effectively maintaining the same level of accuracy. However, there are still substantial hurdles to overcome in our endeavour to move this work forward towards personalised medicine and to develop a decision support system that can be used by clinical practitioners, which I will discuss.
If time permits, I will discuss an extension of this framework to uncertainty quantification in the fluid dynamics of the pulmonary blood circulation system, with applications to the diagnosis of pulmonary hypertension (high blood pressure in the lungs).
IR Seminar: Modelling Stopping Criteria for Search Results using a Poisson Processes (04 November, 2019)
Speaker: Mark Stevenson
Title: Modelling Stopping Criteria for Search Results using a Poisson Processes
Abstract:
Text retrieval systems often return large sets of documents, particularly when applied to large collections. These scenarios as common in important applications, such as background literature searches for systematic reviews which are important in evidence-based medicine. Stopping criteria can reduce the number of documents that need to be manually evaluated for relevance by predicting when a suitable level of recall has been achieved. In this work, a novel method for determining a stopping criterion is proposed that models the rate at which relevant documents occur using a Poisson process. This method allows a user to specify both a minimum desired level of recall to achieve and a desired probability of having achieved it. We evaluate our method on a public dataset of systematic review search results and compare it with previous techniques for determining stopping criteria.
Bio:
I am a Senior Lecturer in Sheffield University's Department of Computer Science where I am a member of the Natural Language Processing group.The aim of my research is the development of systems to extract knowledge from text and assist users in accessing this information.
IR Seminar: Hierarchical and Context-aware Query Expansion (28 October, 2019)
Speaker: Shahrzad Naseri
Abstract
Current neural information retrieval models focus on reranking an initial candidate pool of retrieved documents. However, for many queries the core matching algorithms fails to identify many (or even all) relevant results in the candidate pool. To make progress we need more effective core matching algorithms that improve recall. In this talk, I will describe different local and global expansion methods to formulate the query in order to improve the initial candidate pool for difficult hierarchical queries. Next, I will explain the shortcomings of the traditional global expansion methods, i.e. static embedding methods, and how we can incorporate the newly developed contextualized word representations for expansion and core document matching.
Bio
Shahrzad Naseri is a PhD candidate in the Center for Intelligent Information Retrieval (CIIR) in the College of Information and Computer Sciences at the University of Massachusetts Amherst (UMass Amherst) advised by Prof. James Allan. She holds a Master of Science in Computer Science from UMass Amherst in computer science and a Bachelor of Science in Information Technology from Amirkabir University of Technology (Tehran Polytechnic), Iran. She is currently a visiting researcher n School of Computing Science at the University of Glasgow, under the supervision of Prof. Jeff Dalton. Shahrzad’s research lies in the intersection of Information Retrieval and Natural Language Processing (NLP). She is interested in investigating different input representations for information retrieval systems.
Glasgow workshop on Contextual Recommendations (24 October, 2019)
Speaker: Multiple speakers
This is a celebratory workshop, with a keynote talk from Prof Fabio Crestani, presentations of local work at the University of Glasgow, as well as from local company ThinkAnalytics. This workshop is co-located with the PhD defence of Jarana Manotumruksa. The talks will be preceded by lunch (pizzas) and followed by drinks.
Schedule:
1300 - 1400 Lunch
1400 - 1440 [keynote] Prof Fabio Crestani
1440 - 1520 Jarana Manotumruksa - Effective Neural Architectures for Context-Aware Venue Recommendation
1520 - 1540 coffee break
1540 - 1620 Shahad Ahmed/Flavia Veres - Recommendations at ThinkAnalytics
1620 - 1640 Amir Jadinedad - Unifying Explicit and Implicit Feedback for Rating Prediction and Ranking Recommendation Tasks [EPSRC Closed Loop Data Science programme]
1640 - 1700 Ziaqiao Meng - Variational Bayesian Context-aware Representation for Grocery Recommendation [H2020 BigDataStack project]
1700 - 1800 Drinks
Seeing into the past – emerging applications of computer vision and machine learning in archaeology (23 October, 2019)
Speaker: Dave Cowley and Rachel Opitz
Title: Seeing into the past – emerging applications of computer vision and machine learning in archaeology
Abstract:
Awareness of the implications of computer vision, machine learning and AI is growing within the archaeological research community. This seminar looks at how the uptake of these methods is impacting several of our own archaeological research projects, centred around the key question: how do we recognize and classify archaeological features and objects? Within our current projects, a major focus of research is how to leverage these approaches to facilitate the analysis of large landscape datasets like Airborne Laser Scanning, while exploring the basis on which archaeological identifications and classification are made. The development of this application is raising questions, including the efficacy of working with derived visualisations rather than raw surface models or 3D shape data, the role of training sets when classes and categories are poorly defined, and how to integrate these approaches into archaeological workflows and research practice. Parallel questions arise in projects on perceptual saliency-based approaches to classification, and on the detection and classification of wear on objects. This seminar presents work in progress and aims to highlight the particular challenges of working with archaeological data and practices.
Bios:
Dave Cowley is an archaeologist at Historic Environment Scotland, where he manages a two-year research and development project scoping approaches to rapid large area archaeological mapping. He has developed an interest in computer vision and AI for archaeology, recognising it as a valuable mechanism to expedite archaeological object detection, especially with complex and extensive data, and to explore how we define archaeological sites and landscapes.
Rachel Opitz is a lecturer in Archaeology at Glasgow. Her research focuses on applications of remote sensing and digital 3D data in archaeology. She is particularly interested in visual perception of digital 3D objects and features, and in the impact of new technologies on archaeological practice. Current projects focus on working with archaeological landscape data in VR, detecting use-wear on digital 3D models of archaeological objects, and perceptual saliency based approaches to object classification.
Vision Guided Autonomous Inspection for Manufacturing and Re-manufacturing Industry (09 October, 2019)
Speaker: Dr Aamir Khan
Ultrasonic based Non-destructive Testing (NDT) has seen wide applications in recent years. Achieving flexible automation for such testing method is a growing research area. In this regard, enabling automated vision-guided robotic ultrasonic tool-path based NDT inspections are desirable in the manufacturing and re-manufacturing industry. The complexity of this task is augmented by the varying nature of the parts. This talk will be about structure from motion (SfM) based vision-guided robotic NDT inspection. An automated and model free vision system is proposed and integrated into a robotic work-cell that can produce 3D models of challenging objects with sub-millimeter accuracy. These 3D models are used to generate the tool-path for ultrasonic probe to approach the surface of an object in the robotic work-cell and perform NDT inspection. Different SOA approached are also impended to perform image acquisition in order to capture the appearance of an object and provide the effects of these approaches on the 3D models obtained from SfM. We demonstrate that the developed automated vision system can achieve sub-millimeter accuracy for the 3D models with low texture, self-similar and glossy objects, without having to perform training of input data. Furthermore, these 3D models are used to generate the Ultrasonic tool-path for NDT inspection of parts.
Bio: I am a Research Associate at the University of Strathclyde working in the area of Computer/Robot Vision since April 2018. I work on several research and industrial projects where I apply my computer vision/robot vision skills to address a wide range of challenges in different application areas. Currently, my work focus is on building 3D models of parts that are required to go through NDT inspection in a Manufacturing and Remanufacturing industry. Prior to joining CUE Robotics Team at University of Strathclyde, I graduated with a Doctorate degree from University of Glasgow in 2018. I was also working as Part time Research Assistant at University of Glasgow, for an Innovate UK project.
IR Seminar: The Semantic Space of Human Activity Phrases (07 October, 2019)
Speaker: Steve Wilson
In this talk, I will describe work on training and tuning models that can produce vector representations of human activity phrases that correlate with several dimensions of humans’ notions of semantics. I will show how we can use these representations to automatically cluster human activity phrases based on their semantic relations with one another, and I will describe how we can train deep learning models to make predictions about the clusters of activities that Twitter uses are likely to tweet about doing.
Speaker Bio:
IR Seminar: Dialogue-Based Information Retrieval (30 September, 2019)
Speaker: Abhishek Kaushik
Speaker Bio:
Machine Learning Models for Inference from Outliers (26 September, 2019)
Speaker: Mahesan Niranjan
Abstract:
While much of recent literature on machine learning address regression and classification problems, several problems of interest relate to detecting a relatively small number of outliers from large collections of data. Such problems have been addressed in the context of target tracking, condition monitoring of complex engines and patient health monitoring in an intensive care setting, for example. The popular approach, in these settings, of estimating a probability density over normal data and comparing the likelihood of a test observation against a threshold set from this suffers the well known problem of the curse of dimensionality. Circumventing this involves modelling – data driven or otherwise – to capture known relationships in the data and looking for novelty in the residuals. This talk will describe several problems taken from the Computational Biology, Chemistry and Fraud Detection domains to illustrate this. We will discuss structured matrix approximation and tensor methods for multi-view data and suitable algorithms for their estimation.
Speaker:
Mahesan Niranjan is Professor of Electronics and Computer Science at the University of Southampton. Prior to this appointment in 2008, he has held academic positions at the University of Cambridge as Lecturer in Information Engineering and at the University of Sheffield as Professor of Computer Science. At Sheffield, he also served as Head of Computer Science and Dean of Engineering. His research is in the area of Machine Learning, and he has worked on both the algorithmic and applied aspects of the subject. Some of his work has been fairly influential in the field – e.g. the SARSA algorithm widely used in the Reinforcement Learning literature. More recently, his focus of research is in data-driven inference problems in computational biology. More from:
Deep Residual Learning for Everyday Computer Vision Tasks (06 September, 2019)
Speaker: Chaitanya Kaul
Residual learning is a concept in neural networks that exploits feature reuse from intermediate layers of a neural network to create more robust feature embeddings. In this talk, I will present three deep learning architectures that deal with the processing of 2D images and 3D point clouds, exploiting residual learning. I will present the evaluation of these models on benchmark medical image segmentation datasets as well as benchmark 3D point cloud classification and segmentation datasets. The results show high performance gains compared to the benchmarks, as well as highly competitive performance with respect to the state of the art techniques.
IR Seminar: Implicit User Feedback in Open-Ended Dialogs, Alex Chuklin (Google) (02 September, 2019)
Speaker: Alex Chuklin
Present-day dialog systems are either trained on a vast amount of chit-chat data—and therefore cannot support a meaningful conversation,—or built to assist the user in a particular well-defined domain and therefore are limited by nature. We argue that to develop a truly open-domain dialog system grounded in knowledge we need a new learning setup.
In this talk I will present our work on predicting user satisfaction from variety of behavioral signals and discuss how such implicit satisfaction signal can be used to power the online user-based learning of a dialog system. This talk is based on the joint work with Ming Zeng, Qi Guo, Dmitry Lagun, and Junfeng He.
IR Seminar: The Potential of Making Sense of Quantities for IR (16 July, 2019)
Speaker: Yusra Ibrahim
Abstract:
Numbers are an integral part of the language, though they are often
overlooked by Natural Language Understanding (NLU) and Information
Extraction research. Numerical quantities appear in scientific research
results, financial reports, and medical records, among others. They are
arranged in tabular formats or infused in natural text. In this talk, I
will present how we harness this abundance of data towards building the
next-generation Information Retrieval systems, that are capable of
answering complex queries about quantities. I will present our recent
work in aligning quantity mentions in tables and text, and in answering
quantity-constrained search queries. I will also touch upon future
challenges and open problems in this domain.
Bio
Yusra Ibrahim is a Ph.D. Student at Max-Planck Institute for Informatics
(MPI-INF). Her primary research interest is Information Extraction. The
topic of her thesis is understanding quantities in web tables and their
surrounding text.
Yusra obtained her Master's degree from the University of Pierre and
Marie Curie (Paris 6). During her master's, she collaborated with the
National Library of France (BNF) and the Max Planck Institute in Named
Entity Recognition and Disambiguation. She spent a few years developing
software products for various industries. She has publications in
top-tier conferences, such as CIKM, ICDE, WWW, and ISWC.
Machine learning in optics: from solving inverse problems in imaging to high-speed hardware implementations (07 June, 2019)
Speaker: Alejandro Turpin
Advanced computational algorithms such as machine learning and Bayesian inference have left their traditional space within computing science and are impacting multiple areas, such as biomedical imaging, artificial vision, and neuroscience. In this talk I will discuss two different works where machine learning, in particular artificial neural networks, have been used in inverse problems in imaging to overcome the limitations from hardware: imaging through complex media and 3D imaging with single point detectors.
Trained to Fuzz! (13 May, 2019)
Speaker: Martin Sablotny
Software testing is used to ensure the correct functionality of a program and to discover flaws in the software which can introduce security issues. A prominent software testing technique is so-called fuzz testing. Here, a test case generator creates input data for a program under test and the execution of it is monitored to discover unintended behaviour. However, developing test case generators for fuzz testing is a labour intensive task mainly because it is necessary to study the format specifications and reimplement them before even starting to generate any test cases. In this talk, I’ll outline a novel machine learning based approach which can significantly speed up the development of fuzz testers. First, I’ll show that it is possible to improve an existing fuzzer by utilising generative deep learning methods and provide guidance on how to select good performing model without actually executing any test cases. Secondly, readily available real-world data is used to train a test generator from ground up. Finally, I will outline how deep reinforcement learning can be applied to fuzz testing and teach the fuzzer how to generate test cases which maximises code coverage in a closed-loop manner.
SICSA DVF Masterclass - Predicting multi-view and structured data with kernel methods (10 May, 2019)
Speaker: Prof. Juho Rousu (SICSA DVF)
During the last two decades, kernel methods - including, but not limited to the celebrated support vector machine - have been extremely successful in many walks of life. They continue to be a good alternative to deep neural networks in many real-world applications where data is complex and high-dimensional, and the amount of training data is medium-scale - from hundreds to a few tens of thousands of training examples.
In this masterclass I will focus on how kernel methods can be used for applications where the prediction setup involves heterogeneous or structured data, in particular learning with multiple data sources and predicting structured output.
Bibliography
Bhadra, S., Kaski, S. and Rousu, J., 2017. Multi-view kernel completion. Machine Learning, 106(5), pp.713-739.
Cichonska, A., Pahikkala, T., Szedmak, S., Julkunen, H., Airola, A., Heinonen, M., Aittokallio, T. and Rousu, J., 2018. Learning with multiple pairwise kernels for drug bioactivity prediction. Bioinformatics, 34(13), pp.i509-i518.
Hue, M. and Vert, J.P., 2010, June. On learning with kernels for unordered pairs. In ICML (pp. 463-470).
Marchand, M., Su, H., Morvant, E., Rousu, J. and Shawe-Taylor, J.S., 2014. Multilabel structured output learning with random spanning trees of max-margin markov networks. In Advances in Neural Information Processing Systems (pp. 873-881).
Scholkopf, B. and Smola, A.J., 2001. Learning with kernels: support vector machines, regularization, optimization, and beyond. MIT press.
Shawe-Taylor, J. and Cristianini, N., 2004. Kernel methods for pattern analysis. Cambridge university press.
Su, H., Gionis, A. and Rousu, J., 2014, January. Structured prediction of network response. In International Conference on Machine Learning (pp. 442-450).
Su, H. and Rousu, J., 2015. Multilabel classification through random graph ensembles. Machine Learning, 99(2).
Taskar, B., Guestrin, C. and Koller, D., 2004. Max-margin Markov networks. In Advances in neural information processing systems (pp. 25-32).
Tsochantaridis, I., Joachims, T., Hofmann, T. and Altun, Y., 2005. Large margin methods for structured and interdependent output variables. Journal of machine learning research, 6(Sep), pp.1453-1484.
Machine Learning for Energy Disaggregation (30 April, 2019)
Speaker: Mingjun Zhong
The speaker is a candidate for a Lectureship in the School
Energy disaggregation, i.e., non-intrusive load monitoring, is a technique to separate home appliances from only the mains electricity meter readings. Energy disaggregation is a single channel Blind Source Separation problem and is thus unidentifiable. In this talk, I will present how machine learning methods could be devised to tackle this unidentifiable problem. Firstly, energy disaggregation was represented as a factorial hidden Markov model (FHMM). Bayesian methods were then developed to infer the appliance sources from the mains readings. I will present how domain knowledge can be integrated into FHMM to alleviate the unidentifiable problem. Secondly, energy disaggregation was represented as a supervised learning problem. We thus proposed a sequence-to-point (seq2point) learning with neural networks for energy disaggregation. Interestingly, we showed that interpretable fingerprints for electricity appliances could be extracted from the mains, which were used for energy disaggregation essentially.
Multimodal Deep Learning with High Generalisation across Mobile Recognition Tasks (23 April, 2019)
Speaker: Valentin Radu
Lectureship candidate.
A growing number of devices around us embed a variety of sensors and sufficient computation power to enable them intelligent (e.g., smartphones, smart-watches, smart-toothbrushes). Despite the many sensors available, applications often use just one sensor for a task, e.g., accelerometer to count the number of steps, barometer to detect changes in elevation. By this they miss out on the opportunity to capture complementary sensing perspectives from multiple sensors to increase robustness and to enable more advanced context recognition information. Combining many sensing modalities is not easy. In this presentation I will show that using deep learning can gracefully integrate diverse sensing modalities efficiently across many recognition tasks. In our proposed solution we dedicate neural network structures to extract features specific to each sensing modality followed by additional bridging layers to perform the classification across distilled features. We show this approach generalises well across a number of recognition tasks specific to mobile and wearable devices, while operating within suitable energy budgets.
Small Molecule Identification through Machine Learning: CSI:FingerID and beyond (17 April, 2019)
Speaker: Prof. Juho Rousu (SICSA DVF)
Abstract
Identification of small molecules from biological samples remains a major bottleneck in understanding the inner workings of biological cells and their environment. Machine learning on data from large public databases of tandem mass spectrometric data has transformed this field in recent years, with tools like CSI:FingerID, and CSI:IOKR demonstrating a step-change improvement in identification rates compared to previous approaches. In this presentation, I will give an overview of the technology inside these tools and review some recent developments in making use of additional information sources for improving the identification rates, in particular learning to predict the order of molecules eluting from liquid-chromatographic system.
Dührkop, K., Fleischauer, M., Ludwig, M., Aksenov, A.A., Melnik, A.V., Meusel, M., Dorrestein, P.C., Rousu, J. and Böcker, S., 2019. SIRIUS 4: a rapid tool for turning tandem mass spectra into metabolite structure information. Nature Methods 16, pp- 299-302
Short Bio:
Juho Rousu is a Professor of Computer Science at Aalto University, Finland. Rousu obtained his PhD in 2001 form University of Helsinki, while working at VTT Technical Centre of Finland. In 2003-2005 he was a Marie Curie Fellow at Royal Holloway University of London. In 2005-2011 he held Lecturer and Professor positions at University of Helsinki, before moving to Aalto University in 2012 where he leads a research group on Kernel Methods, Pattern Analysis and Computational Metabolomics (KEPACO). Rousu’s main research interest is in learning with multiple and structured targets, multiple views and ensembles, with methodological emphasis in regularised learning, kernels and sparsity, as well as efficient convex/non-convex optimisation methods. His applications of interest include metabolomics, biomedicine, pharmacology and synthetic biology.
IR Seminar: Recommendations in a Marketplace: Personalizing Explainable Recommendations with Multi-objective Contextual Bandits (08 April, 2019)
Speaker: Rishabh Mehrotra
In recent years, two sided marketplaces have emerged as viable business models in many real world applications (e.g. Amazon, AirBnb, Spotify, YouTube), wherein the platforms have customers not only on the demand side (e.g. users), but also on the supply side (e.g. retailer, artists). Such multi-sided marketplace involves interaction between multiple stakeholders among which there are different individuals with assorted needs. While traditional recommender systems focused specifically towards increasing consumer satisfaction by providing relevant content to the consumers, two-sided marketplaces face an interesting problem of optimizing their models for supplier preferences, and visibility.
In this talk, we begin by describing a contextual bandit model developed for serving explainable music recommendations to users and showcase the need for explicitly considering supplier-centric objectives during optimization. To jointly optimize the objectives of the different marketplace constituents, we present a multi-objective contextual bandit model aimed at maximizing long-term vectorial rewards across different competing objectives. Finally, we discuss theoretical performance guarantees as well as experimental results with historical log data and tests with live production traffic in a large-scale music recommendation service.
IR seminar: Unbiased Learning to Rank from User Interactions (01 April, 2019)
Speaker: Harrie Oosterhuis
On the Road to a Transfer Learning Paradigm based on Interpretable Factors of Variation (29 March, 2019)
Speaker: Tameem Adel
For the last two years, I have been working on addressing other challenges and limitations of deep models, most notably challenges that relate to the integration of such models within real-world applications, e.g. interpretability and fairness.
I will show an example of an algorithm, referred to as prediction difference analysis, providing (local) explanations of classification decisions taken by deep models. On the other hand, developing global explanations by learning interpretable data representations is also becoming ever more important as machine learning models grow in size and complexity. In our ICML-2018 paper, we proposed two rather contrasting interpretability frameworks. The first aims at controlling the accuracy vs. interpretability tradeoff by providing an interpretable lens for an existing model (which has already been optimized for accuracy). We developed an interpretable latent variable model whose data are the representation in an existing (generative or discriminative) model, weakly supervised by limited side information. We extended the approach using an active learning strategy to choose the most useful side information to obtain, allowing a human to guide what "interpretable" means. The second framework relies on joint optimization for a representation which is both maximally informative about the interpretable information and maximally compressive about the non-interpretable data factors. This leads to a novel perspective on the relationship between compression and regularization. An intriguing, related perspective is that of developing a quantified interpretability paradigm where learning can be transferred among tasks, based on (partially) interpretable factors of variation.
I will also briefly speak about other topics I have been working on prior to that, e.g. learning and approximate inference on probabilistic graphical models (PGMs) and transfer learning.
Post-CHIIR IR Seminar (15 March, 2019)
Speaker: Jaime Arguello and Adam Roegiest
IR Seminar: Topic-centric sentiment analysis of UK parliamentary debate transcripts (25 February, 2019)
Speaker: Gavin Abercrombie
Debate transcripts from the UK House of Commons provide access to a wealth of information concerning the opinions and attitudes of politicians and their parties towards arguably the most important topics facing societies and their citizens, as well as potential insights into the democratic processes that take place within Parliament.
By applying natural language processing and machine learning methods to debate speeches, it is possible to automatically determine the attitudes and positions expressed by speakers towards the topics they discuss.
This talk will focus on research on speech-level sentiment analysis and opinion-topic/policy detection, as well as discussing the challenges of working in this domain.
Bio
Gavin Abercrombie holds a Masters degree in IT & Cognition from the University of Copenhagen, and is currently a second-year PhD student at the School of Computer Science, University of Manchester. His research interests include natural language understanding and computational social science.
Challenges and Opportunities at the Intersection of the Computing and Social Sciences (21 February, 2019)
Speaker: Multiple speakers
Joint Variational Uncertain Input Gaussian Processes (20 February, 2019)
Speaker: Carl Edward Rasmussen & Adrià Garriga-Alonso
Standard mean-field variational inference in Gaussian Processes with uncertain inputs systematically underestimates posterior uncertainty. In particular, the factorisation assumption employed in the approximating distribution severely limits the framework’s accuracy. We lift this assumption, and show that the resulting scheme gives much more realistic predictive uncertainties, and can be implemented in a sparse and practical way. The algorithm has implications for latent variable models generally, including stacked (Deep) GPs and time series models.
IDI Journal Club: Graph Attention Networks (31 January, 2019)
Speaker: Joshua Mitton
In this journal club meeting, Josh will lead the discussion of the paper "Graph Attention Networks".
Abstract:
We present graph attention networks (GATs), novel neural network architectures that operate on graph-structured data, leveraging masked self-attentional layers to address the shortcomings of prior methods based on graph convolutions or their approximations. By stacking layers in which nodes are able to attend over their neighbourhoods’ features, we enable (implicitly) specifying different weights to different nodes in a neighbourhood, without requiring any kind of computationally intensive matrix operation (such as inversion) or depending on knowing the graph structure upfront. In this way, we address several key challenges of spectral-based graph neural networks simultaneously, and make our model readily applicable to inductive as well as transductive problems. Our GAT models have achieved or matched state-of-the-art results across four established transductive and inductive graph benchmarks: the Cora, Citeseer and Pubmed citation network datasets, as well as a protein-protein interaction dataset (wherein test graphs remain unseen during training).
Paper:
https://mila.quebec/wp-content/uploads/2018/07/d1ac95b60310f43bb5a0b8024522fbe08fb2a482.pdf
Big Hypotheses: a generic tool for fast Bayesian Machine Learning (18 January, 2019)
Speaker: Prof. Simon Maskell
- There are many machine learning tasks that would ideally involve global optimisation across some parameter space. Researchers often pose such problems in terms of sampling from the distribution and favour Markov Chain Monte Carlo (MCMC) or its derivatives (e.g., Gibbs sampling, Hamiltonian Monte Carlo (HMC) and Simulated annealing). While these techniques can offer good results, they are slow. We describe an alternative numerical Bayesian algorithm, the Sequential Monte Carlo (SMC) sampler. SMC samplers are closely related to particle filters and are reminiscent of genetic algorithms. More specifically, an SMC sampler replaces the single Markov chain considered by MCMC with a population of samples. The inherent parallelism present makes the SMC sampler a promising starting point for developing a scalable Bayesian global optimiser, e.g., that runs 86,400 times faster than MCMC and might be able to be 86,400 times more computationally efficient. The University of Liverpool and STFC’s Hartree centre have recently started working on a £2.5M EPSRC-funded project (with significant support from IBM, NVidia, Intel and Atos) to develop SMC samplers into a general purpose scalable numerical Bayesian optimisation and embody them as a back-end in the software package Stan. This talk will summarise recent developments, initial results (in a subset of problems posed by Astrazeneca, AWE, Dstl, Unilever, physicists, chemists, biologists and psychologists) and planned work over the next 5 years towards developing a high-performance parallel Bayesian inference implementation that can be used for a wide range of problems relevant to researchers working in a range of application domains.
Quantum inspired image compression. (11 December, 2018)
Speaker: Bruno Sanguinetti
Pushing image sensors and algorithms to the quantum limit (11 December, 2018)
Speaker: Bruno Sanguinetti
IR Seminar: Measuring User Satisfaction and Engagement (10 December, 2018)
Speaker: Adam Zhou
Abstract:
In the online world, it is important to design user-centric applications that can engage the users and make them satisfied. In order to improve user satisfaction and engagement, one prerequisite is to find effective ways to measure them.
In this talk, I will present several efforts to measure satisfaction and engagement in the context of search and mobile app usage. Firstly, I will present my work that aims to find the best metrics (either offline or online) for various search scenarios: organic, aggregated and image search. Secondly, I will talk about various ways how mobile users engage with the apps and how to exploit them to predict their next engagement. Finally, I will very briefly cover some of our current work on conversational search.
Bio:
Ke (Adam) Zhou holds dual academic and industrial appointments as an assistant professor in the school of computer science at University of Nottingham, and a Senior Research Scientist at Nokia Bell Labs. His research interests and expertise lie in web search and analytics, evaluation metrics, text mining and human computer interaction. He has published over 50 publications in reputable conferences and journals. His past researches have won the best paper award in ECIR'15 and CHIIR'16, and best paper honorable mention in SIGIR'15.
IR Seminar: Alana: Entertaining and Informative Open-domain Social Dialogue using Ontologies and Entity Linking (03 December, 2018)
Speaker: Ioannis Konstas
IR Seminar: The Quantified Self as Testbed for Multimodal Information Retrieval (19 November, 2018)
Speaker: Frank Hopfgartner
Investigating How Conversational Search Agents Affect User’s Behaviour, Performance and Search Experience (05 November, 2018)
Speaker: Mateusz Dubiel
Voice based search systems currently do not support natural conversational interaction. Consequently, people tend to limit their use of voice search to simple navigational tasks, as more complex search tasks require more sophisticated dialogue modelling. Previous research has demonstrated that a voice based search system’s inability to preserve contextual information leads to user’s dissatisfaction and discourages further usage. In my talk I will explore how people’s search behaviour, performance and perception of usability change when interacting with a conversational search system which supports natural language interaction, as opposed to a voice based search system which does not.
Short bio:
Mateusz Dubiel is a PhD candidate in the department of Computer and information Sciences at Strathclyde University in Glasgow . His research is focused on development and evaluation of conversational search agents. Mateusz holds an MSc in Speech and Language Processing from The University of Edinburgh.
IR Seminar: Measuring the Utility of Search Engine Result Pages (08 October, 2018)
Speaker: Dr. Leif Azzopardi
Web Search Engine Result Pages (SERPs) are complex responses to queries, containing many heterogeneous result elements (web results, advertisements, and specialised ``answers'') positioned in a variety of layouts. This poses numerous challenges when trying to measure the quality of a SERP because standard measures were designed for homogeneous ranked lists.
In this talk, I will explain how we developed a means to measure the utility and cost of SERPs.
To ground this work we adopted the C/W/L framework by Moffat et al which enables a direct comparison between different measures in the same units of measurement, i.e. expected (total) utility and cost. I argue that the extended C/W/L framework provides a clearer and more interpretable framework for measurement i.e. utility, cost (in time), and also predicted stopping rank - the latter two are both directly observable - and so the quality of the metric can be assessed by how well it predicts these observables.
Within this framework, we proposed a new measure based on Information Foraging Theory, which can account for the heterogeneity of elements, through different costs, and which naturally motivates the development of a user stopping model that adapts behaviour depending on the rate of gain. This directly connects models of how people search with how we measure search, providing a number of new dimensions in which to investigate and evaluate user behaviour and performance.We perform an analysis over 1000 popular queries issued to a major search engine, and report the aggregate utility experienced by users over time. Then in an comparison against common measures, we show that the proposed foraging based measure provides a more accurate reflection of the utility and of observed behaviours (stopping rank and time spent).
Talk: Performance-oriented management in the large-scale cluster (08 October, 2018)
Speaker: Dr Chao Chen
To support a number of complex data analysis frameworks in different areas, a maintainable large-scale cluster with the required QoS is necessary. The cluster management is the core element can not only orchestrate various data analysis frameworks and services to harmoniously coexist, but also maximise the performance and utilisation to cluster physical machines as much as possible. This presentation will focus on resources provision, allocation and job scheduling for the cluster management.
Talk: Resource management in Grid and Cloud Infrastructures (08 October, 2018)
Speaker: Dr Hamid Arabnejad
Increasing availability of different type of resources in Grid and Cloud platforms associated with today's fast-changing, and unpredictability of submitted workload, has propelled an interest towards self-adaptive manager system that dynamically detects and reallocates system resources to user’s applications in order to optimize the given Quality of service (e.g. performance, energy, reliability, resource utilization) for the target platform. However, finding an effective resource management solution to support diverse application performance objectives in heterogeneous computing environments becomes a difficult challenge.
Resource Management (RM) is the collective term that describes the best practices, processes, procedures, and technology tools to manage available resources in the target platform. RM has a focus across multiple aspects, such as applications, servers, networking, and storage, to address efficient usage of available resources to meet user application requirement objectives while addressing performance, availability, capacity, and energy requirements in a cost-effective manner.
The focus of this talk will discuss issues and challenges of resource allocation and scheduling in Grid and Cloud systems. We will first provide a characterization of workload and resource management. Then, we will then describe our recent work to address this challenge.
Towards data-driven hearing aid solutions (04 October, 2018)
Speaker: Widex staff
Widex will give an informal overview of the company and current challenges in the hearing aid domain. We will discuss challenges related to data collection, machine learning and real-time optimisation with humans in the loop.
Variational Sparse Coding (13 June, 2018)
Speaker: Francesco Tonolini
We propose a new method for sparse coding based on the variational auto-encoder architecture, which allows sparse representations with generally intractable probabilistic models. We assume data to be generated from a sparse distribution prior in the latent space of a generative model and aim to maximise the observed data likelihood with a variational auto-encoding approach. We consider both the Laplace and the spike and slab priors and in each case derive an analytic approximation to the regularisation term in the variational lower bound, making posterior inference as efficient as in the standard variational auto-encoder case. By inducing sparsity in the prior, training results in a recognition function that generates sparse representations of observed data. Such representations can then be used as information-rich inputs to further learning tasks.
Deep, complex networks for inversion of transmission effects in multimode optical fibres (30 May, 2018)
Speaker: Oisin Moran
We use complex-weighted, deep convolutional networks to invert the effects of multimode optical fibre distortion of a coherent input image. We generated experimental data based on collections of optical fibre responses to greyscale, input images generated with coherent light, and measuring only image amplitude (not amplitude and phase as is typical) at the output of the \SI{10}{\metre} long \SI{105}{\micro\metre} diameter multimode fibre. This data is made available as the {\it Optical fibre inverse problem} Benchmark collection. The experimental data is used to train complex-weighted models with a range of regularisation approaches and subsequent denoising autoencoders. A new {\it unitary regularisation} approach for complex-weighted networks is proposed which performs best in robustly inverting the fibre transmission matrix, which fits well with the physical theory.
Modelling the creative process through black-box optimisation (23 May, 2018)
Speaker: Anders Kirk Uhrenholt
IR Seminar: Controversy Analysis and Detection (21 May, 2018)
Speaker: Shiri Dori-Hacohen
IR Seminar: Understanding and Leveraging the Impact of Response 1 Latency on User Behaviour in Web Search (18 May, 2018)
Speaker: Ioannis Arapakis
Understanding Capsule Networks (16 May, 2018)
Speaker: Piotr Ozimek
Abstract:
In recent years convolutional neural networks (CNNs) have revolutionized the fields of computer vision and machine learning. On multiple occasions, they have achieved state of the art performance on a variety of vision tasks, such as object detection, classification and segmentation. In spite of this CNNs suffer from a variety of problems: they require large and diverse datasets that may be expensive to obtain, they do not have an explicit and easy to interpret internal object representation, and they are easy to fool by manipulating spatial relationships between visual features in the input image. To address these issues Hinton et. al. have devised a new neural network architecture called the Capsule Network (CapsNet), which consists of explicit and encapsulated neural structures whose output represents the detected object or feature in a richer and more interpretable format. CapsNets are a new concept that is still being researched and developed, but they have already achieved state of the art performance on the MNIST dataset without any data augmentation. In this talk, I will give a brief overview of the current state of CapsNets, explain the motivation behind them as well as their architecture.
Bio:
Surviving the Flood of Big Data Streams (30 April, 2018)
Speaker: Richard McCreadie
Research talk abstract: The way big data is being processed is evolving from predominantly batch-based analysis of static datasets, towards to microservice-driven architectures designed to analyse big data streams. This change raises new challenges for both data systems enginers examining how to build efficient and scalable architectures/platforms; as well as for researchers and developers looking to extract value from emerging real-time streams. In this talk, I will discuss how real-time streaming data is altering the research landscape from the perspective of real-time event detection and modelling. In particular, I will cover my past and present research in this area, focusing on challenges in data systems development, event detection from real-time streams, as well as how to model information from event streams over time. I will conclude the talk with a discussion on some promising new research directions to examine in this area in the future.
Lectureship abstract: We are asking all IDA Lectureship candidates to give a 15 minute lecture, as if they were teaching Level 4 undergraduates. The topic is “Explaining the matrix factorisation (MF) approach for collaborative filtering”.
Scaling Entity Linking with Crowdsourcing (23 April, 2018)
Speaker: Dyaa Albakour
In this presentation, we first review the current state-of-the-art for the EL task and make the case for using supervised learning approaches to tackle EL. These approaches require large amounts of labelled data, which represent a bottleneck for scaling them out to cover large numbers of entities. To mitigate this, we have developed a production-ready solution to efficiently collect high-quality labelled data at scale using Active Learning and Crowdsourcing. In particular, we will discuss the different steps and the challenges in tuning the design parameters of the crowdsourcing task. The design parameters include the qualification of the workers and UI features that help them complete the task. The tuning aims to limit the noise, reduce the cost and maximise the throughput of labelling whilst maintaining the quality of the resulting models for EL.
Signal Media is a research-led company that uses text analytics and machine learning to turn streams of unstructured text, e.g. news articles, into useful information for professional users. One of the core components of Signal’s text analytics pipeline is entity linking (EL).
Shard Effects on Effectiveness (18 April, 2018)
Speaker: Mark Sanderson
Title
Shard Effects on Effectiveness
Abstract
Studying the experimental factors that impact IR measures is often overlooked when comparing IR systems. In particular, the effects of splitting the document collection into shards has not been examined in detail. I will talk about our use of the general linear mixed model framework and present a model that encompasses the experimental factors of system, topic, shard, and their interaction effects. The model allows us to more accurately estimate significant differences between the effect of various factors. We study shards created by various methods used in prior work and better explain observations noted in prior work in a principled setting and offer new insights. Notably, I describe how we discovered that the topic*shard interaction effect is large, almost globally across all datasets, an observation that has not been recognised or measured before to our knowledge.
Prototyping Deep Learning Applications Through Knowledge Transfer (16 April, 2018)
Speaker: Nina S Dethlefs
Deep learning plays an ever increasing role in artificial intelligence and a growing number of libraries facilitate the fast development of new applications. For each new learning task, some trial and error is normally required to tune hyperparameters or find an adequate learning representation etc before a suitable prediction model can be learnt. In this talk, I explore the possibility of transferring hyperparameters (and learning representations) from one task to another based on the tasks’ similarity. The idea is to reuse previously acquired knowledge and in this way reduce time and development costs and speed up prototyping of new deep learning applications. I present a number of case studies from natural language processing and other AI tasks that show how knowledge transfer can - in some cases - lead to state-of-the-art performance on unseen tasks while substantially reducing computation time. Embedding important operations into a generalised abstract framework, e.g. a domain specific programming language, facilitates prototyping even further.
Bio
I am a Lecturer in Computer Science at the University of Hull, UK. I lead the Big Data Analytics groups and I am a member of the Computational Science group. Previously, I was a Research Fellow at the Interaction Lab at Heriot-Watt University, Edinburgh. I have a PhD in Computational Linguistics from the University of Bremen, Germany. My research interests are in computational intelligence and machine learning - particularly deep learning and optimisation - as well as natural language processing. I investigate how machine learning algorithms themselves can be equipped with intelligence so as to enable transfer learning across domains and learning tasks. Most of my work has been in natural language processing but I have also worked in other areas, including health informatics and human-robot interaction.
Simulating Interaction for Evaluation (09 April, 2018)
Speaker: Leif Azzopardi
Search is an inherently interactive, non-deterministic and user-dependent process. This means that there are many different possible sequences of interactions which could be taken (some ending in success and others ending in failure). Simulation provides a powerful tool for low-cost, repeatable and reproducible evaluations which explore a large range of different possibilities - and enables the analysis of IR systems, interfaces, user behaviour and user strategies. To run a simulation, a model of the user is formalised, and then used, for example, as the basis of a metric, to create a test collection, or generate interaction data. In this talk, I will give an overview of various methods that we have developed in order to: (1) create simulated test collections which enable more extensive evaluations, as well as enable the evaluation on new collections without the expense of costly user judgements, and (2) create user interaction data, which enables a range of different user strategies/behaviours to be compared and contrasted in a systematic manner.
Bio: Dr. Leif Azzopardi is a Chancellor's Fellow in Data Science and Associate Professor at the University of Strathclyde, Glasgow within Department of Computer and Information Science. He leads the Interactive Information Retrieval group within Strathclyde's iSchool. His research focuses on examining the influence and impact of search technology on people and society and is heavily underpinned by theory. He has made numerous contributions in: (i) the development of statistical language models for document, sentence, expert retrieval, (ii) the simulation and evaluation of users and their interactions, (iii) the analysis of systems and retrieval bias using retrievability theory and the (iv) the formalisation of search and search behaviour using economic theory. He has given numerous keynotes, invited talks and tutorials through out the world on retrievability, search economics, and simulation. He is co-author of the Tango with Django (www.tangowithdjango.com) which has seen over 1.5 million visitors. And more recently he has been co-developing resources for IR research with Lucene (www.github.com/lucene4ir/), while co-creating evaluation resources for Technology Assisted Reviews as part of the CLEF eHealth Track 2017. He is an honorary lecturer at the University of Glasgow (where he was previously a Senior Lecturer) and an honorary Adjunct Associate Professor at Queensland University of Technology. He received his Ph.D. in Computing Science from the University of Paisley in 2006, under the supervision of Prof. Mark Girolami and Prof. Keith van Rijsbergen. Prior to that he received a First Class Honours Degree in Information Science from the University of Newcastle, Australia, 2001.
Analyzing and Using Large-scale Web Graphs (29 March, 2018)
Speaker: Ansgar Scherp
The talk first provides an overview about my research in Data Science, namely text and data mining. Subsequently, I focus on graph data mining on the Web. I have developed a schema-level index called SchemEX in order to be able to search in large-scale web graphs. The SchemEX index can be efficiently computed in a stream-based fashion with reasonable accuracy over graphs of billions of edges. The data search engine LODatio+ (see: http://lodatio.informatik.uni-kiel.de/) uses the SchemEX index to find relevant data sources. In order to quickly develop, tailor, and compare schema-level indices, I provide a novel formal, parameterized model for schema-level indices. A grant challenge is to deal with the evolution of the web graphs, specifically their schema in terms of types and properties used to describe entities. I have investigated the dynamics of entities in order to find, e. g., periodicities in the schema changes, and to use this information to predict future changes. This is important for various future data-driven applications that aim at using graph data on the web.
IR Seminar: Using Synthetic Text for Developing Content Coordination Metrics and Semantic Verification (12 March, 2018)
Speaker: Dmitri Roussinov
Recurrent neural language models allowed generating realistically looking synthetic texts, but the use of those texts for scientific purposes has been largely unexplored. I will present my work in progress and some forthcoming results on using simulated text for developing the metrics for catching coordinated content in microblogs (e.g. Twitter trolling attacks) and verifying semantic classes of words (e.g. France is a country, Gladiator is a movie, but not a country) for question answering applications. My simulation results support the conjecture that only when the metric takes the context and the properties of the repeated sequence into consideration, it is capable of separating organic and coordinated content. I will also demonstrate how those context-specific adjustments can be obtained using existing resources.
Bio:
Dr. Roussinov is a Senior Lecturer in Computer and Information Sciences University of Strathclyde. He has contributed to the fields of information systems, information retrieval, natural language processing, search engines, security informatics, medical informatics, human computer interaction, databases and others. He received his doctoral degree in Information Systems from the University of Arizona (advisor H. Chen), Master’s in Economics from Indiana University, and his undergraduate in Physics and computer science from Moscow Institute of Physics and Technology.
Learning from samples of variable quality (26 February, 2018)
Speaker: Mostafa Deghani
The success of deep neural networks to date depends strongly on the availability of labeled data which is costly and not always easy to obtain. Usually, it is much easier to obtain small quantities of high-quality labeled data and large quantities of unlabeled, weak or noisy data. The problem of how to best integrate these two different sources of information during training and hot to get best of samples of variable quality is an active pursuit in the field of semi-supervised learning. In this talk, we are going to discuss some methods for training neural networks with labels with different quality.
Bio:
Mostafa Dehghani is a PhD student at the University of Amsterdam working with Jaap Kamps and Maarten de Rijke. His doctorate research lies at the intersection machine learning and information retrieval, in particular employing weak supervision signals for training neural models for IR problems. He has contributed to top-tier ML and IR conferences like NIPS, ICLR, SIGIR, CIKM, WSDM, and ICTIR by publishing papers and giving tutorials and received awards at SIGIR, ICTIR, ECIR, and CLEF for some of his works. He has done internships at Google Research on search conversationalization and currently interning at Google Brain.
SOCIAL & CROSS-DOMAIN RECOMMENDATIONS (19 February, 2018)
Speaker: Dimitrios Rafailidis
How the selections of social friends can influence user preferences in recommender systems? How can we exploit distrust relationships when generating product, movie or song recommendations? In the first part of my talk I will present my recent research in social recommender systems, and how these questions are answered to produce accurate recommendations by considering both trust and distrust relationships.
While Amazon users can rate products from different domains, such as books, toys and clothes, they do not necessarily have the same behavior when different types of products are recommended, making the widely used collaborative filtering strategy underperform. So, the main challenge is to carefully transfer the knowledge of user preferences from one domain to another by handling their different behaviors accordingly. In the second part of my talk, I will demonstrate my recently proposed algorithm for generating cross-domain recommendations and how the different user behaviors are weighted across multiple domains."
BIO: "Dimitrios Rafailidis is a postdoctoral research fellow at the Department of Computer Science at UMons in Belgium. His research interests are recommender systems and social media mining. His primary research goal is to generate personalized recommendations of massive, multimodal and streaming user data from different social media platforms, or any source that can capture user preferences. His main focus is on capturing user preference dynamics, and producing social and cross-domain recommendations. The results from this research have been published in leading peer reviewed journals, like TBD, TiiS, TOMCCAP, TMM, TCBB, TSMC, TASLP and SNAM, and highly selective conference proceedings such as RecSys, ECML/PKDD, CIKM, SIGIR, WWW and ASONAM."
QUANTITATIVE EVALUATION OF CANINE PELVIC LIMB ATAXIA USING A WIRELESS ACCELEROMETER SYSTEM (15 February, 2018)
Speaker: Rodrigo Gutierrez-Quintana
R. Gutierrez-Quintana, K.L. Holmes, Z. Hatfield, P. Amengual Batle, J. Brocal, K. Lazzerini, R. José-López. Small Animal Hospital, School of Veterinary Medicine, University of Glasgow, UK.
An inexpensive and easily available method for objectively identifying and grading pelvic limb ataxia in dogs in the clinical setting is urgently needed. An alternative approach to conventional gait analysis techniques is the use of accelerometers attached to the body. They have the advantages of being low cost and allowing non-restrictive evaluation in a normal environment.
The purpose of this prospective study was to perform gait analysis using a lumbar accelerometer in dogs with pelvic limb ataxia and healthy controls; and assess whether the data obtained could be used to differentiate these 2 groups.
Fifty-three dogs (21 healthy controls and 32 dogs with pelvic limb ataxia) of different size breeds were included. All dogs were walked in a straight line, on a non-slippery surface, at a slow walking pace for 50 meters using a short lead. Acceleration signals were measured using a wireless tri-axial accelerometer that was secured with an elastic band at the level of the fifth lumbar vertebra. The average and coefficient of variation of the peak-to-peak amplitude was calculated for each acceleration component (x: Cranio-caudal, y: Latero-lateral and z; Dorso-ventral). Mann-Whitney test was used to compare groups (p<0.05).
A significant difference between affected and control dogs was identified in the coefficient of variation of the x axis (p<0.0001).
The results of the present study suggest that the coefficient of variation of the cranio-caudal axis could represent an objective measure of pelvic limb ataxia in dogs. Further longitudinal studies in a larger number of cases are indicated.
IR Seminar: A Survey of Information Retrieval Approaches with Embedded Word Vectors (05 February, 2018)
Speaker: Debasis Ganguly
IR Seminar: Natural Language Understanding in Virtual Agents for Airline Pilots. (22 January, 2018)
Speaker: Sylvain Daronnat
Abstract:
This presentation summarizes a 6 months master's internship that took place at Airbus (Toulouse, France) around a virtual agent research thematic for airline pilots. Our initial hypothesis was that a intent categorization system could benefit from using synthetic “natural-like” data. In order to test this hypothesis we decided, first, to create a methodology that would help us collect natural questions from end-users. Then we used the “natural” data we previously collected along with a synthetic question generator we designed in order to output synthetic questions that are as close as possible from the original ones. Lastly, we experimented on the synthetic datasets using various tools in order to put to the test our initial hypothesis. The results we obtained allowed us to open new perspectives on the natural language understanding part of the virtual agent system for airline pilots.
Short bio:
My name is Sylvain Daronnat, I'm a PhD student in computer and information sciences at Strathclyde University working on implementing new human-agent collaboration systems aboard submarines. For this project I'm also funded by Thales, a company designing electrical systems for various industries. Before my PhD, I was studying Natural Language Processing at the Grenoble Alpes University in France.
Approaches to analysis of genomic data (17 January, 2018)
Speaker: Thomas Otto
A huge amount of data in biological sciences are generated in the hope to answer biological questions. This is possible due to the decreased price of high throughput methods. Although many analysis tools exist, there is a need to improve many of them. Further, there are many opportunities to develop new methods by combining existing dataset sets.
In this talk, I will present some of the datasets and the methods we used/developed to analyse genomic data, including genomic and transcriptional data from malaria. I will also describe anticipated data, such as single cell RNA-Seq or detection of biomarkers.
Automated Clinical Patient Health Surveillance (15 January, 2018)
Speaker: Stewart Whiting
Part1: Calibration Brain-Computer Interfaces. Part2: The need for more flexible robotics tools (14 December, 2017)
Speaker: Jonathan Grizou
Abstract: Recent works have explored the use of brain signals to directly control virtual and robotic agents in sequential tasks. So far in such brain-computer interfaces (BCI), an explicit calibration phase was required to build a decoder that translates raw electroencephalography (EEG) signals from the brain of each user into meaningful instructions. In this talk, I will explain how we removed the need for a calibration phase. In practice, this means being able to interactively teach an agent to perform a task without it knowing beforehand how to associate the human communicative signals with their meanings. In a second part, I will talk about the open source robotic project Poppy and how it was used in art, education and research. This will bring us to the need for more flexible and modular tools to accelerate the design of robotics products.
Bio: Jonathan is currently a PostDoc within the Cronin group in charge of the Chemobot Team. The team explores how robots and algorithms can become tools for the exploration and discovery of complex physicochemical systems. Jonathan pursued his PhD at the INRIA and Ensta-ParisTech Flowers Team where he investigated how to create calibration-free interactive systems. He was advised by Manuel Lopes and Pierre-Yves Oudeyer and received the ''Prix Le Monde de la Recherche Universitaire'' 2015 for his thesis work. Jonathan is also a long-time maker and an active member of the Poppy project, an open-source project providing tools to enable the creative exploration of interactive robots for science, education, and art. Recently, and together with three robotics specialists, he co-founded Pollen Robotics a young start-up aiming to make robotic product development much simpler.
Websites:
- https://www.pollen-robotics.com/
Going beyond relevance: Incorporating effort into Information retrieval (04 December, 2017)
Speaker: Manisha Verma
Abstract:
Relevance lies at the core of evaluation of Information retrieval systems. However, with rapid development in search algorithms, a myriad number of search devices and increasing complexity of user information needs, we argue that relevance can no longer be the primary criteria for design and evaluation of IR systems. In this talk, I shall provide a brief overview of our work on characterizing, measuring and incorporating effort in IR.
The first half of the talk shall highlight our work on characterizing and measuring document specific effort. I shall provide a brief overview of how effort can be incorporated in information retrieval. I shall outline one important source of the mismatch between search log based evaluation and offline relevance judgments: the high degree of effort required to identify and consume relevant information in a document. I shall describe how to incorporate effort into existing learning to rank algorithms and their performance on publicly available datasets.
The second half of the talk shall focus on device-specific effort. Users have access to same information on several devices today. Our work attempts to analyze in-depth the differences between mobile and desktop. I shall give a brief overview of how judgments on both devices may differ significantly for different documents. I shall touch on the features that are useful in predicting effort across devices. Finally, I shall close the talk with some unresolved research questions and some failed attempts.
Bio:
Manisha Verma is a final year Ph.D. student in Media futures group at University College London. Her primary area of research is characterizing user effort and incorporating it in retrieval and evaluation. Some of her recent work has been published at conferences such as CIKM, WSDM, ECIR, and SIGIR. Over the past few years, Manisha has worked with researchers at Google, Microsoft, and Yahoo on understanding role of user effort in retrieval. She has served as an Ambassador for postgraduate women at UCL and a co-coordinator of the Tasks Track in TREC 2015-2016 and TREC CAR Track in 2017.
IR seminar: Summarizing the Situation with Social Media Streams (27 November, 2017)
Speaker: Richard McCreadie
When a crisis hits, it is important for response agencies to quickly determine the situation on the ground, such that they can deploy the limited resources at their disposal as quickly and effectively as possible. However, during an emergency, information is difficult to come-by, as response units often need to arrive on the scene before the severity of the situation can be estimated. On the other hand, during emergencies, the general public is gravitating to social media platforms to ask for assistance and to show what they see to their friends. As such, emergency services are increasingly interested in technologies that can extract relevant information from social media during an emergency, to aid situational awareness. Meanwhile, real-time summarization is an emerging field that aims to build timeline summaries of events that are happening in the world, using news and social media streams as sensors. In this talk, I will provide an overview of what emergency services want to extract from social media, and how real-time summarization systems can help achieve this. Furthermore, I will discuss current technologies and techniques for real-time summarization that are relevant to the crisis domain, along with the challenges that are yet to be solved.
Bio:
Richard McCreadie is a Research Associate at the University of Glasgow, UK. He is an information retrieval specialist, as well as developer and manager for the Terrier open source IR platform, which has been downloaded over 40,000 times since 2004. His research is focused on the interface between streaming IR and social media, tackling topics such information retrieval architectures for real-time stream processing; leveraging social media for event sensing (detecting events, extracting knowledge and summarizing those events); evaluation methodologies for streaming IR; and social media analytics, particularly when applied to security-related use-cases such as disaster management.
Richard received his Ph.D on the topic of News Vertical Search using User Generated Content in 2012 and is currently a senior researcher within the Terrier Team IR research group in Glasgow. Furthermore, he works with researchers and industry partners around the world to advance the IR field as co-chair of the streaming summarization evaluation initiatives (2014-Present) and 2018 Incident Streams emergency informatics initiative (2018) at the Text Retrieval Conference (TREC). He is active in the research community with 26 published conference papers in the areas of IR and social media, in addition to articles in longer formats, such as a book on Search in Social Media published in the highly-cited FnTIR series. Richard is also a current PC member for the top-tier conferences in the IR field (ACM CIKM, ACM SIGIR, AAAI ICWSM and ACM WSDM).
IR Seminar: A Study of Snippet Length and Informativeness: Behaviour, Performance and User Experience (20 November, 2017)
Speaker: David Maxwell
Neural Models for Information Retrieval (06 November, 2017)
Speaker: Bhaskar Mitra
Abstract: In the last few years, neural representation learning approaches have achieved very good performance on many natural language processing (NLP) tasks, such as language modelling and machine translation. This suggests that neural models may also yield significant performance improvements on information retrieval (IR) tasks, such as relevance ranking, addressing the query-document vocabulary mismatch problem by using semantic rather than lexical matching. IR tasks, however, are fundamentally different from NLP tasks leading to new challenges and opportunities for existing neural representation learning approaches for text.
IR Seminar: Jarana Manotumruksa (30 October, 2017)
Speaker: Jarana Manotumruksa
IR Seminar: Incorporating Positional Information and Other Domain Knowledge into a Neural IR Model (23 October, 2017)
Speaker: Andrew Yates
Optimal input for low reliability assistive technology (19 October, 2017)
Speaker: John Williamson
Recognition of Grasp Points for Clothes Manipulation under unconstrained Conditions (12 October, 2017)
Speaker: Luz Martinez
Abstract: I will talk about a system for recognizing grasp points in RGB-D images. This system is intended to be used in domestic robots when deploying clothes lying at random positions on a table. By taking into consideration that the grasp points are usually near key parts of clothing, such as the waist of pants or the neck of a shirt. Also, I will cover my recent work on clothing simulators that I use to obtain images to train deep learning networks.
Short-bio: Luz is a PhD student in Electrical Engineering at the University of Chile; and currently, a visiting research student in the Computer Vision and Autonomous group. Luz has worked with service robots for 4 years and has expertise in computer vision, computational intelligence, voice recognition and high-level behaviours design. She is currently working on her PhD thesis and she focuses on clothing recognition using active vision.
Leveraging from Ontologies in machine learning (05 October, 2017)
Speaker: David Stirling
This presentation considers a number of successful cases that have significantly benefited from the inclusion of an ontology framework. Firstly, a human bespoke ontology describing cyclic temporal control states has enabled successful multi-objective control (an intelligent autopilot) of a simulated aircraft. Secondly, an empirically learnt ontology was derived to identifying several industrial process modalities, which were exploited to reveal underlying causal factors for a set of undesirable modes (states) of high heat loads in a Blast Furnace. The first case reviews a novel approach for learning and building computational models of human skills that are typically utilized in complex control situations. Such skills are often internalized as sub-cognitive and automatic responses, such as those routinely used in driving a car. Previously, a degree of success in modelling these was reported via behavioural cloning. However, skills obtained by this technique, often exhibit a lack of generality and robustness when applied to different control tasks. This is now mitigated in the alternative presented approach, by segmenting and compressing a universal set of reaction plans with symbolic induction methods. This approach is termed, Compressed Heuristic Universal Reaction Planners or CHURPs. The substantially improved robustness and control performance arises from synergistic interactions and collaborations between the different CHURPs entities including, surrogate control and goal sharing. In the latter case, an abstracted ontology containing nine major heat load modalities, was initially learnt as a 38 state Gaussian Mixture Model from several years of Blast Furnace heat load data, and subsequently utilized to diagnose the casual influences determining these states. Such methodologies are now being pursued in a number of kinematic rehabilitation motion studies, as well as oncology and radiotherapy aspects of cancer care.
Bio:
Dr Stirling obtained his BEng degree from the Tasmanian College of Advanced Education (1976), an MSc (Digital Techniques) in from Heriot-Watt University, Scotland UK (1980), and his PhD from the University of Sydney (1995). He has worked for over 20 years in wide range of industries, including as a Principal Research Scientist with BHP Steel. More recently he joined the University of Wollongong as a Senior Lecturer. David has developed a wide range of expertise in data analysis and knowledge management with skills in problem solving, statistical methods, visualization, pattern recognition, data fusion and reduction. He has applied machine learning and data mining techniques in specialized classifier designs for noisy multivariate data to medical research, exploration geo-science, and financial markets, as well as to industrial primary operations.
Gesture Typing on Virtual Tabletop: Effect of Input Dimensions on Performance (28 September, 2017)
Speaker: Antoine Loriette
A Theory of How People Make Decisions Through Interaction (14 September, 2017)
Speaker: Andrew Howes
Amplifying Human Abilities: Digital Technologies to Enhance Perception and Cognition (12 September, 2017)
Speaker: Albrecht Schmidt
Historically the use and development of tools is strongly linked to human evolution and intelligence. The last 10.000 years show a stunning progress in physical tools that have transformed what people can do and how people live. Currently, we are at the beginning of an even more fundamental transformation: the use of digital tools to amplify the mind. Digital technologies provide us with entirely new opportunities to enhance the perceptual and cognitive abilities of humans. Many ideas, ranging from mobile access to search engines, to wearable devices for lifelogging and augmented realty application give as first indications of this transition. In our research we create novel digital technologies that systematically explore how to enhance human cognition and perception. Our experimental approach is to: first, understand the users in their context as well as the potential for enhancement. Second, we create innovative interventions that provide functionality that amplifies human capabilities. And third, we empirically evaluate and quantify the enhancement that is gained by these developments. It is exciting to see how ultimately these new ubiquitous computing technologies have the potential for overcoming fundamental limitations in human perception and cognition.
Data-Efficient Learning for Autonomous Robots (23 August, 2017)
Speaker: Marc Deisenroth
Fully autonomous systems and robots have been a vision for many decades, but we are still far from practical realization. One of the fundamental challenges in fully autonomous systems and robots is learning from data directly without relying on any kind of intricate human knowledge. This requires data-driven statistical methods for modeling, predicting, and decision making, while taking uncertainty into account, e.g., due to measurement noise, sparse data or stochasticity in the environment. In my talk I will focus on machine learning methods for controlling autonomous robots, which pose an additional practical challenge: Data-efficiency, i.e., we need to be able to learn controllers in a few experiments since performing millions of experiments with robots is time consuming and wears out the hardware. To address this problem, current learning approaches typically require task-specific knowledge in form of expert demonstrations, pre-shaped policies, or the underlying dynamics. In the first part of the talk, I follow a different approach and speed up learning by efficiently extracting information from sparse data. In particular, I propose to learn a probabilistic, non-parametric Gaussian process dynamics model.By explicitly incorporating model uncertainty in long-term planning and controller learning my approach reduces the effects of model errors, a key problem in model-based learning. Compared to state-of-the art reinforcement learning our model-based policy search method achieves an unprecedented speed of learning, which makes is most promising for application to real systems. I demonstrate its applicability to autonomous learning from scratch on real robot and control tasks. In the second part of my talk, I will discuss an alternative method for learning controllers for bipedal locomotion based on Bayesian Optimization, where it is hard to learn models of the underlying dynamics due to ground contacts. Using Bayesian optimization, we sidestep this modeling issue and directly optimize the controller parameters without the need of modeling the robot's dynamics.
NOTE MEETING ROOM CHANGE - NOW IN SAWB 303 DUE TO DELAYS IN BUILDING WORK COMPLETION
Context-aware and Context-Driven Applications on the Web (07 July, 2017)
Speaker: Yong Zheng
Context-awareness has been explored and applied in multiple areas, including ubiquitous computing, information retrieval and recommender systems. We may need to collect contexts in advance, so that the system can make changes by adapting to these dynamic situations. Obviously, it is much easier to collect these information from sensors in ubiquitous computing, but the process of context acquisition becomes one of the challenges in the Web applications. In this talk, we introduce the context-aware applications on the Web, especially based on the information retrieval and recommender systems. In addition, we highlight and discuss the context-driven applications that may influence the process of context collections, user interface and interactions, as well as relevant algorithms to support these novel applications.
Bio:
Dr. Yong Zheng obtained his PhD degree in Computer and Information Sciences from DePaul University, USA. Currently, he is a full-time senior lecturer at Illinois Institute of Technology, USA. His research lie in user modeling, behavior analysis, human factors (user emotions and personalities), context-awareness, multi-criteria decision making, educational learning, and recommender systems. Particularly, he is one of the experts in the context-aware recommender systems. And he served as a data science consultant at NPAW (Nice People At Work), Barcelona, Spain to help them build context-aware recommendation engines. He published more than two dozens of academic papers related to his research topics. He served as publicity chair at ACM RecSys 2018 and ACM IUI 2018. He organized multiple workshops related to recommender systems. And he was invited as PC members for a number of academic conferences, such as WWW, ACM RecSys, ACM UMAP, ACM IUI, etc
Building relevance judgments automatically for a test collection. (19 June, 2017)
Speaker: Mireille Makary
In this talk, I will talk about my ongoing research and will present two different approaches I used to build relevance judgments (qrels) for TREC test collections without any human intervention. I will talk about an approach that involves using keyphrases extraction and another based on supervised machine learning techniques using Naïve Bayes and Support vector machines classifiers.
Bio: I am a PhD student at the University of Wolverhampton, Research Group in Computational Linguistics. My research area is in information retrieval. I am also a lecturer at Computer Science Department in the International University - Lebanon.
Effectively and Efficiently Searching Among Sensitive Content (08 June, 2017)
Speaker: Professor Douglas W. Oard
In Europe today, people have a “right to be forgotten.” Exercising that right requires identifying each Web page that a person wishes to have removed from a search engine’s index. In Maryland today, people have no right to record what they hear in the course of a day without the consent of every person whom they hear. The law provides that the penalty for doing so could be as much as a year in jail for the first offence. In many jurisdictions today, citizens have a right to request information held by their government. Government officials who seek to sift through that information to determine which parts are releasable sometimes take so long to do so that the public purpose for which the request was originally made simply cannot be served. In this talk I will argue that each of these problems arises from the same cause: an almost complete lack of attention to building language technologies that can proactively protect sensitive content. I will further claim that the language technology for performing these tasks is well within the present state of the art, but that we will need to co-evolve the design of our information systems with the legislative, regulatory and normative public policy frameworks within those new capabilities would be employed. Finally, I will illustrate the considerations that arise by describing a new project in which we are seeking to integrate protection for sensitive content into a search engine that is designed to provide public access to collections in which sensitive and non-sensitive content are intermixed and unlabelled.
About the Speaker:
Douglas Oard is a Professor at the University of Maryland, College Park (USA), with joint appointments there in the College of Information Studies (Maryland’s iSchool) and the University of Maryland Institute for Advanced Computer Studies (UMIACS). Dr. Oard earned his Ph.D. in Electrical Engineering from the University of Maryland. His research interests center around the use of emerging technologies to support information seeking by end users. Additional information is available at http://terpconnect.umd.edu/~oard/.
Simple Rules from Chaos: Towards Socially Aware Robotics using Agent-Local Cellular Automata (08 May, 2017)
Speaker: Alexander Hallgren
Controlling robotic agents requires complex control methods. This study aims to take advantage of emergent behaviours to reduce the amount of complexity. Cellular automata (CA) are employed as a means to generate emergent behaviour at low computational cost. A novel architecture is developed based on subsumption architecture, which uses an agent-local CA to influences the selection of a behaviour. The architecture is tested by measuring the time it takes the robot to navigate through a maze. 2 different models are used to evaluate the system. The results indicate that the current configuration is ineffective, but a number of task are proposed as future work.
Spatial Smoothing in Mass Spectrometry Imaging (08 May, 2017)
Speaker: Arijus Pleska
In this paper, we target a data modelling approach used in computational metabolomics; to be specic, we assess whether spatial smoothing improves the topic term and noise identification. By assessing mass spectrometry imaging data, we design an enhancement for latent Dirichlet allocation-based topic models. For both data pre-processing and topic model design, we survey relevant research. Further, we present the proposed methodology in detail providing the prelimi- naries and guiding through the performed topic model en hancements. To assess the performance, we evaluate the spatial smoothing application on a number
Integrating a Biologically Inspired Software Retina with Convolutional Neural Networks (08 May, 2017)
Speaker: Piotr Ozimek
1) a visual attention mechanism that preserves high frequency information around the foveal focal point by the use of space-variant subsampling
2) a conforming and inherently scale and rotation invariant mapping for presenting images to the ConvNet
3) a highly parameterizable image compression process
The method is based on the mammalian retino-cortical transform. This is the first attempt at integrating such a process to ConvNets. To evaluate the method a dataset was built from ImageNet and a set of ConvNets with identical architectures was trained on raw, partially pre-processed and fully pre-processed images. The ConvNets achieved comparable results, suggesting an untapped potential in drawing inspirations from natural vision systems.
Investigation of users' affective and physiological traits in a multi-modal interaction context (04 May, 2017)
Speaker: Iulia Popescu
In this talk, I will present my Level 5 (MSci) project which explored how users react and what they feel when they are exposed to different types of stimuli (visual, auditory). This study aimed to understand how short-term stressors impact individuals’ behaviour when they need to complete a task in a multi-modal interaction context (e.g. searching for a flight using graphical and spoken dialogue interfaces). Additionally, I will give an overview about the data set which has been delivered as part of this project and how it can be used for further research.
Real-time Mobile Object Removal using Google Project Tango (04 May, 2017)
Speaker: Rhys Simpson
Visually removing objects from a video feed is difficult to perform in real-time, as existing solutions rely on expensive patch lookups and specific environment conditions to produce meaningful results. Results are also guessed from the image surrounding the object, usually making them physically inaccurate and visually displeasing. Recent advances in hardware and software are pushing businesses to make large investments into Augmented Reality, including furniture catalogue applications, which could greatly benefit if existing objects could be visually removed from the video feed in real-time. This paper demonstrates a novel approach where instead of painting frames in an entirely 2D context, a 3D room mesh is captured, tracked and selectively rendered to paint geometry that was behind the object over it. The object's mask, and filled textures covering the planes the object was in contact with are also sourced and tracked from this mesh. Our approach works for a broad range of objects in typical indoors scenes, where target objects are separate and against large wall and floor planes. We show that our algorithm produces much better results per frame than object removal using traditional 2D inpainting, at an interactive framerate, and demonstrate that temporal incoherence between subsequent video frames is also eliminated.
IDA Seminar: Probabilistic Deep Learning: Models for Unsupervised Representation Learning (04 May, 2017)
Speaker: Dr Sebastian Nowozin
An important problem in achieving general artificial intelligence is the data-efficient learning of representations suitable for causal reasoning, planning, and decision making. Learning such representations from unsupervised data is challenging and requires flexible models to discover the underlying manifold of high-dimensional data. Recently three new classes of unsupervised learning approaches based on deep learning have enabled major progress towards large-scale unsupervised learning: generative adversarial networks (GAN), variational autoencoders (VAE), and approaches based on integral probability metrics (IPM).
I will provide an overview of these methods, research contributions by my group, and the main open research questions around this new class of learning methods.
Big Crisis Data - an exciting frontier for applied computing. (24 April, 2017)
Speaker: Carlos Castillo
Social media is an invaluable source of time-critical information during a crisis. However, emergency response and humanitarian relief organizations that would like to use this information struggle with an avalanche of social media messages, exceeding their capacity to process them. In this talk, we will look at how interdisciplinary research has enabled the creation of new tools for emergency managers, decision makers, and affected communities. These tools typically incorporate a combination of automatic processing and crowdsourcing. The talk will also look at ethical issues of this line of research.
http://bigcrisisdata.org/
ProbUI: Generalising Touch Target Representations to Enable Declarative Gesture Definition for Probabilistic GUIs (20 April, 2017)
Speaker: Daniel Buschek
We present ProbUI, a mobile touch GUI framework that merges ease of use of declarative gesture definition with the benefits of probabilistic reasoning. It helps developers to handle uncertain input and implement feedback and GUI adaptations. ProbUI replaces today's static target models (bounding boxes) with probabilistic gestures ("bounding behaviours"). It is the first touch GUI framework to unite concepts from three areas of related work: 1) Developers declaratively define touch behaviours for GUI targets. As a key insight, the declarations imply simple probabilistic models (HMMs with 2D Gaussian emissions). 2) ProbUI derives these models automatically to evaluate users' touch sequences. 3) It then infers intended behaviour and target. Developers bind callbacks to gesture progress, completion, and other conditions. We show ProbUI's value by implementing existing and novel widgets, and report developer feedback from a survey and a lab study.
Information Foraging in Environments (31 March, 2017)
Speaker: Kevin Ong
Kevin is a PhD student from ISAR Research Group at RMIT University, Australia. Kevin had previously worked on logs from National Archives UK, Peter MacCallum Cancer Institute, Westfield Group and Listcorp.
In this talk, he will talk about his work on information foraging in physical and virtual environments. The first part of his talk will be on "Understanding information foraging in physical environment - a log analysis" and the second part of his talk will be on "information foraging in virtual environments - an observational study".
Semantic Search at Bloomberg. (27 March, 2017)
Speaker: Edgar Meij
Abstract:
Large-scale knowledge graphs (KGs) store relationships between entities that are increasingly being used to improve the user experience in search applications. At Bloomberg we are currently in the process of rolling out our own knowledge graph and in this talk I will describe some of the semantic search applications that we aim to support. In particular, I will be discussing some of our recent papers on context-specific entity recommendations and automatically generating textual descriptions for arbitrary KG relationships.
Bio:
Dr. Edgar Meij is a senior scientist at Bloomberg. Before this, he was a research scientist at Yahoo Labs and a postdoc at the University of Amsterdam, where he also obtained his PhD. His research focuses on advancing the state of the art in semantic search at Web scale, by designing entity-oriented search systems that employ knowledge graphs, entity linking, NLP, and machine learning techniques to improve the user experience, search, and recommendations. He has co-authored 50+ peer-reviewed papers and regularly teaches at the post-graduate level, including university courses, summer schools, and conference tutorials.
Assessing User Engagement in Information Retrieval Systems (20 March, 2017)
Speaker: Mengdie Zhuang
Abstract:
In this study, we investigated both using user actions from log files, and the results of the User Engagement Scale, both of which came from a study of people interacting with a retrieval interface containing an image collection, but with a non-purposeful task. Our results suggest that selected behaviour measures are associated with selected user perceptions of engagement (i.e., focused attention, felt involvement, and novelty), while typical search and browse measures have no association with aesthetics and perceived usability. This is finding can lead towards a more systematic user-centered evaluation model.
Bio:
Mengdie Zhuang is a PhD student from the University of Sheffield, UK. Her research focuses on evaluation metrics of Information Retrieval Systems.
Access, Search and Enrichment in Temporal Collections (06 March, 2017)
Speaker: Avishek Anand
There have been numerous efforts recently to digitize previously published content and preserving born-digital content leading to the widespread growth of large temporal text repositories. Temporal collections are continuously growing text collections which contain versions of documents spanning over long time periods and present many opportunities for historical, cultural and political analyses. Consequently there is a growing need for methods that can efficiently access, search and mine them. In this talk we deal with approaches in each of these aspects -- access, search and enrichment. First, I describe some of the access methods for searching temporal collections. Specifically, how do we index text to support temporal workloads? Secondly, I will describe retrieval models, which exploit historical information, essential in searching such collections. That is, how do we rank documents given temporal query intents? Finally, I will present some of the ongoing efforts to mine such collections for enriching Knowledge sources like Wikipedia.
A stochastic formulation of a dynamical singly constrained spatial interaction model (02 March, 2017)
Speaker: Mark Girolami
One of the challenges of 21st-century science is to model the evolution of complex systems. One example of practical importance is urban structure, for which the dynamics may be described by a series of non-linear first-order ordinary differential equations. Whilst this approach provides a reasonable model of spatial interaction as are relevant in areas diverse as public health and urban retail structure, it is somewhat restrictive owing to uncertainties arising in the modelling process.
We address these shortcomings by developing a dynamical singly constrained spatial interaction model, based on a system of stochastic differential equations. Our model is ergodic and the invariant distribution encodes our prior knowledge of spatio-temporal interactions. We proceed by performing inference and prediction in a Bayesian setting, and explore the resulting probability distributions with a position-specific metropolis-adjusted Langevin algorithm. Insights from studies of interactions within the city of London from retail structure are used as illustration
Collaborative Information Retrieval. (27 February, 2017)
Speaker: Nyi Nyi Htun
Presentation of 2 papers to appear at CHIIR 2017.
Paper 1:
Title: How Can We Better Support Users with Non-Uniform Information Access in Collaborative Information Retrieval?
Abstract: The majority of research in Collaborative Information Retrieval (CIR) has assumed that collaborating team members have uniform information access. However, practice and research has shown that there may not always be uniform information access among team members, e.g. in healthcare, government, etc. To the best of our knowledge, there has not been a controlled user evaluation to measure the impact of non-uniform information access on CIR outcomes. To address this shortcoming, we conducted a controlled user evaluation using 2 non-uniform access scenarios (document removal and term blacklisting) and 1 full and uniform access scenario. Following this, a design interview was undertaken to provide interface design suggestions. Evaluation results show that neither of the 2 non-uniform access scenarios had a significant negative impact on collaborative and individual search outcomes. Design interview results suggested that awareness of team’s query history and intersecting viewed/judged documents could potentially help users share their expertise without disclosing sensitive information.
Paper 2:
Title: An Interface for Supporting Asynchronous Multi-Level Collaborative Information Retrieval
Abstract: Case studies and observations from different domains including government, healthcare and legal, have suggested that Collaborative Information Retrieval (CIR) sometimes involves people with unequal access to information. This type of scenario has been referred to as Multi-Level CIR (MLCIR). In addition to supporting collaboration, MLCIR systems must ensure that there is no unintended disclosure of sensitive information, this is an under investigated area of research. We present results of an evaluation of an interface we have designed for MLCIR scenarios. Pairs of participants used the interface under 3 different information access scenarios for a variety of search tasks. These scenarios included one CIR and two MLCIR scenarios, namely: full access (FA), document removal (DR) and term blacklisting (TR). Design interviews were conducted post evaluation to obtain qualitative feedback from participants. Evaluation results showed that our interface performed well for both DR and FA scenarios but for TR, team members with less access had a negative influence on their partner’s search performance, demonstrating insights into how different MLCIR scenarios should be supported. Design interview results showed that our interface helped the participants to reformulate their queries, understand their partner’s performance, reduce duplicated work and review their team’s search history without disclosing sensitive information.
A Comparison of Document-at-a-Time and Score-at-a-Time Query Evaluation (14 February, 2017)
Speaker: Joel Mackenzie
We present an empirical comparison between document-at-a-time (DaaT) and score-at-a-time (SaaT) document ranking strategies within a common framework. Although both strategies have been extensively explored, the literature lacks a fair, direct comparison: such a study has been difficult due to vastly different query evaluation mechanics and index organizations. Our work controls for score quantization, document processing, compression, implementation language, implementation effort, and a number of details, arriving at an empirical evaluation that fairly characterizes the performance of three specific techniques:WAND (DaaT), BMW (DaaT), and JASS (SaaT). Experiments reveal a number of interesting findings. The performance gap between WAND and BMW is not as clear as the literature suggests, and both methods are susceptible to tail queries that may take orders of magnitude longer than the median query to execute. Surprisingly, approximate query evaluation in WAND and BMW does not significantly reduce the risk of these tail queries. Overall, JASS is slightly slower than either WAND or BMW, but exhibits much lower variance in query latencies and is much less susceptible to tail query effects. Furthermore, JASS query latency is not particularly sensitive to the retrieval depth, making it an appealing solution for performance-sensitive applications where bounds on query latencies are desirable.
Bio:
Joel is a PhD candidate at RMIT University, Melbourne, Australia. He works with Dr J. Shane Culpepper and Assoc Prof. Falk Scholer on efficient and effective candidate generation for multi-stage retrieval. His research interests include index efficiency, multi-stage retrieval and distributed IR.
Unsupervised Event Extraction and Storyline Generation from Text (13 February, 2017)
Speaker: Dr. Yulan He
This talk consists of two parts. In the first part, I will present our proposed Latent Event and Categorisation Model (LECM) which is an unsupervised Bayesian model for the extraction of structured representations of events from Twitter without the use of any labelled data. The extracted events are automatically clustered into coherence event type groups. The proposed framework has been evaluated on over 60 millions tweets and has achieved a precision of 70%, outperforming the state-of-the-art open event extraction system by nearly 6%. The LECM model has been extended to jointly modelling event extraction and visualisation which performs remarkably better than both the state-of-the-art event extraction method and a pipeline approach for event extraction and visualisation.
In the second part of my talk, I will present a non-parametric generative model to extract structured representations and evolution patterns of storylines simultaneously. In the model, each storyline is modelled as a joint distribution over some locations, organisations, persons, keywords and a set of topics. We further combine this model with the Chinese restaurant process so that the number of storylines can be determined automatically without human intervention. The proposed model is able to generate coherent storylines from new articles.
Bio:
Yulan He is a Reader and Director of the Systems Analytics Research Institute at Aston University. She obtained her PhD degree in Spoken Language Understanding in 2004 from the University of Cambridge, UK. Prior joining Aston, she was a Senior Lecturer at the Open University, Lecturer at the University of Exeter and Lecturer at the University of Reading. Her current research interests lie in the integration of machine learning and natural language processing for text mining and social media analysis. Yulan has published over 140 papers with most appeared in high impact journals and at top conferences such as IEEE Transactions on Knowledge and Data Engineering, IEEE Intelligent Systems, KDD, CIKM, ACL, etc. She served as an Area Chair in NAACL 2016, EMNLP 2015, CCL 2015 and NLPCC 2015
and co-organised ECIR 2010 and IAPR 2007.
Applying Machine Learning to Data Exploration. (23 January, 2017)
Speaker: Charles Sutton
One of the first and most fundamental tasks in data mining is what we might call data understanding. Given a dump of data, what's in it? If modern machine learning methods are effective at finding patterns in data, then they should be effective at summarizing data sets so as to help data analysts develop a high-level understanding of them.
I'll describe several different approaches to this problem. First I'll describe a new approach to classic data mining problems, such as frequent itemset mining and frequent sequence mining, using a new principled model from probabilistic machine learning. Essentially, this casts the problem of pattern mining as one of structure learning in a probabilistic model. I'll describe an application to summarizing the usage of software libraries on Github.
A second attack to this general problem is based on cluster analysis. A good clustering can help a data analyst to explore and understand a data set, but what constitutes a good clustering may depend on domain-specific and application-specific criteria. I'll describe a new framework for interactive clustering that allows the analyst to examine a clustering and guide it in a way that is more useful for their information need.
Finally, topic modelling has proven to be a highly useful family of methods for data exploration, but it still requires a large amount of specialized effort to develop a new topic model for a specific data analysis scenario. I'll present new results on highly scalable inference for latent Dirichlet allocation based on recently proposed deep learning methods for probabilistic models.
Slides and relevant papers will be available at http://homepages.inf.ed.ac.uk/csutton/talks/
Rethinking eye gaze for human-computer interaction (19 January, 2017)
Speaker: Hans Gellersen
Eye movements are central to most of our interactions. We use our eyes to see and guide our actions and they are a natural interface that is reflective of our goals and interests. At the same time, our eyes afford fast and accurate control for directing our attention, selecting targets for interaction, and expressing intent. Even though our eyes play such a central part to interaction, we rarely think about the movement of our eyes and have limited awareness of the diverse ways in which we use our eyes --- for instance, to examine visual scenes, follow movement, guide our hands, communicate non-verbally, and establish shared attention.
This talk will reflect on use of eye movement as input in human-computer interaction. Jacob's seminal work showed over 25 years ago that eye gaze is natural for pointing, albeit marred by problems of Midas Touch and limited accuracy. I will discuss new work on eye gaze as input that looks beyond conventional gaze pointing. This includes work on: gaze and touch, where we use gaze to naturally modulate manual input; gaze and motion, where we introduce a new form of gaze input based on the smooth pursuit movement our eyes perform when they follow a moving object; and gaze and games, where we explore social gaze in interaction with avatars and joint attention as multi-user input .
Hans Gellersen is Professor of Interactive Systems at Lancaster University. Hans' research interest is in sensors and devices for ubiquitous computing and human-computer interaction. He has worked on systems that blend physical and digital interaction, methods that infer context and human activity, and techniques that facilitate spontaneous interaction across devices. In recent work he is focussing on eye movement as a source of context information and modality for interaction.
The Role of Relevance in Sponsored Search. (16 January, 2017)
Speaker: Fabrizio Silvestri
Sponsored search aims at retrieving the advertisements that in the one hand meet users’ intent reflected in their search queries, and in the other hand attract user clicks to generate revenue. Advertisements are typically ranked based on their expected revenue that is computed as the product between their predicted probability of being clicked (i.e., namely clickability) and their advertiser provided bid. The relevance of an advertisement to a user query is implicitly captured by the predicted clickability of the advertisement, assuming that relevant advertisements are more likely to attract user clicks. However, this approach easily biases the ranking toward advertisements having rich click history. This may incorrectly lead to showing irrelevant advertisements whose clickability is not accurately predicted due to lack of click history. Another side effect consists of never giving a chance to new advertisements that may be highly relevant due to their lack of click history. To address this problem, we explicitly measure the relevance between an advertisement and a query without relying on the advertisement’s click history, and present different ways of leveraging this relevance to improve user search experience without reducing search engine revenue. Specifically, we propose a machine learning approach that solely relies on text-based features to measure the relevance between an advertisement and a query. We discuss how the introduced relevance can be used in four important use cases: pre-filtering of irrelevant advertisements, recovering advertisements with little history, improving clickability prediction, and re-ranking of the advertisements on the final search result page. Offline experiments using large-scale query logs and online A/B tests demonstrate the superiority of the proposed click-oblivious relevance model and the important roles that relevance plays in sponsored search.
Working toward computer generated music traditions (12 January, 2017)
Speaker: Bob Sturm
I will discuss research aimed at making computers intelligent and sensitive enough to working with music data, whether acoustic or symbolic. Invariably, this includes a lot of work in applying machine learning to music collections in order to divine distinguishing and identifiable characteristics of practices that defy strict definition. Many of the resulting machine music listening systems appear to be musically sensitive and intelligent, but their fraudulent ways can be revealed when they are used to create music in the styles they have been taught to identify. Such "evaluation by generation” is a powerful way to gauge the generality of what a machine has learned to do. I will present several examples, focusing in particular on our work applying deep LSTM networks to modelling folk music transcriptions, and ultimately generating new music traditions.
References:
https://github.com/IraKorshunova/folk-rnn
https://highnoongmt.wordpress.com/2015/05/22/lisls-stis-recurrent-neural-networks-for-folk-music-generation/
https://highnoongmt.wordpress.com/?s=%22Deep+learning+for+assisting+the+process%22&submit=Search
https://youtu.be/YMbWwU2JdLg
https://youtu.be/RaO4HpM07hE
https://soundcloud.com/sturmen-1
Studies of Disputed Authorship (09 January, 2017)
Speaker: Michael P. Oakes
Automatic author identification is a branch of computational stylometry, which is the computer analysis of writing style. It is based on the idea that an author’s style can be described by a unique set of textual features, typically the frequency of use of individual words, but sometimes considering the use of higher level linguistic features. Disputed authorship studies assume that some of these features are outside the author’s conscious control, and thus provide a reliable means of discriminating between individual authors. Many studies have successfully made use of high frequency function words like “the”, “of” and “and”, which tend to have grammatical functions rather than reveal the topic of the text. Their usage is unlikely to be consciously regulated by authors, but varies substantially between authors, texts, and even individual characters in Jane Austen’s novels. Using stylometric techniques, Oakes and Pichler (2013) were able to show that the writing style of the document “Diktat für Schlick” was much more similar to that of Wittgenstein than that of other philosophers of the Vienna Circle. Michael Oakes is currently researching the authorship of “The Dark Tower”, normally attributed to C. S. Lewis.
Satisfying User Needs or Beating Baselines? Not always the same. (12 December, 2016)
Speaker: Walid Magdy
Information retrieval (IR) is mainly concerned with retrieving relevant documents to satisfy the information needs of users. Many IR tasks involving different genres and search scenarios have been studied for decades. Typically, researchers aim to improve retrieval effectiveness beyond the current “state-of-the-art”. However, revisiting the modeling of the IR task itself is often essential before seeking improvement of results. This includes reconsidering the assumed search scenario, the approach used to solve the problem, or even the conducted evaluation methodology. In this talk, some well-known IR tasks are explored to demonstrate that beating the state-of-the-art baseline is not always sufficient. Novel modeling, understanding, or approach to IR tasks could lead to significant improvements in user satisfaction compared to just improving “objective” retrieval effectiveness. The talk includes example IR tasks, such as printed document search, patent search, speech search, and social media search.
Supporting Evidence-based Medicine with Natural Language Processing (28 November, 2016)
Speaker: Dr. Mark Stevenson
The modern evidence-based approach to medicine is designed to ensure that patients are given the best possible care by basing treatment decisions on robust evidence. But the huge volume of information available to medical and health policy decision makers can make it difficult for them to decide on the best approach. Much of the current medical knowledge is stored in textual format and providing tools to help access it represents a significant opportunity for Natural Language Processing and Information Retrieval. However, automatically processing documents in this domain is not straightforward and doing so successfully requires a range of challenges to be overcome, including dealing with volume, ambiguity, complexity and inconsistency. This talk will present a range of approaches from Natural Language Processing that support access to medical information. It will focus on three tasks: Word Sense Disambiguation, Relation Extraction and Contradiction Identification. The talk will outline the challenges faced when developing approaches for accessing information contained in medical documents, including the lack of available gold standard data to train systems. It will show how existing resources can help alleviate this problem by providing information that allows training data to be created automatically.
SHIP: The Single-handed Interaction Problem in Mobile and Wearable Computing (24 November, 2016)
Speaker: Hui-Shyong Yeo
Screen sizes on devices are becoming smaller (eg. smartwatch and music player) and larger (eg. phablets, tablets) at the same time. Each of these trends can make devices difficult to use with only one hand (eg. fat-finger or reachability problem). This Single-Handed Interaction Problem (SHIP) is not new but it has been evolving along with a growth of larger and smaller interaction surfaces. The problem is exacerbated when the other hand is occupied (encumbered) or not available (missing fingers/limbs). The use of voice command or wrist gestures can be less robust or perceived as awkward in the public.
This talk will discuss several projects (RadarCat UIST 2016, WatchMI MobileHCI 2016, SWIM and WatchMouse) in which we are working towards achieving/supporting effective single-handed interaction for mobile and wearable computing. The work focusses on novel interaction techniques that are not being explored thoroughly for interaction purposes, using ubiquitous sensors that are widely available such as IMU, optical sensor and radar (eg. Google Soli, soon to be available).
Biography:
Hui-Shyong Yeo is a second year PhD student in SACHI, University of St Andrews, advised by Prof. Aaron Quigley. Before that he worked as a researcher in KAIST for one year. Yeo has a wide range of interest within the field of HCI, including topics such as wearable, gestures, mixed reality and text entry. Currently he is focusing on single-handed interaction for his dissertation topic. He has published in conferences such as CHI, UIST, MobileHCI (honourable mention), SIGGRAPH and journals such as MTAP and JNCA.
Visit his homepage or twitter @hci_research
Demo of Google Soli Radar and Single Handed Smartwatch interaction (24 November, 2016)
Speaker: Hui-Shyong Yeo
This demo session will present the Google Soli Radar and Smartwatch interaction system
Biography:
Hui-Shyong Yeo is a second year PhD student in SACHI, University of St Andrews, advised by Prof. Aaron Quigley. Before that he worked as a researcher in KAIST for one year. Yeo has a wide range of interest within the field of HCI, including topics such as wearable, gestures, mixed reality and text entry. Currently he is focusing on single-handed interaction for his dissertation topic. He has published in conferences such as CHI, UIST, MobileHCI (honourable mention), SIGGRAPH and journals such as MTAP and JNCA.
Visit his homepage or twitter @hci_research
IDA coffee breaks (22 November, 2016)
Speaker: everyone
A chance to catch up informally with members of the IDA section in the Computing Science Common Room.
Human Computation for Entity-Centric Information Access (21 November, 2016)
Speaker: Dr. Gianluca Demartini
Human Computation is a novel approach used to obtain manual data processing at scale by means of crowdsourcing. In this talk we will start introducing the dynamics of crowdsourcing platforms and provide examples of their use to build hybrid human-machine information systems. We will then present ZenCrowd: a hybrid system for entity linking and data integration problems over linked data showing how the use of human intelligence at scale in combination with machine-based algorithms outperforms traditional systems. In this context, we will then discuss efficiency and effectiveness challenges of micro-task crowdsourcing platforms including spam, quality control, and job scheduling in crowdsourcing.
IDA coffee breaks (15 November, 2016)
Speaker: everyone
A chance to catch up informally with members of the IDA section in the Computing Science Common Room.
Control Theoretical Models of Pointing (11 November, 2016)
Speaker: Rod Murray-Smith
I will present an empirical comparison of four models from manual control theory on their ability to model targeting behaviour by human users using a mouse: McRuer's Crossover, Costello's Surge, second-order lag (2OL), and the Bang-bang model. Such dynamic models are generative, estimating not only movement time, but also pointer position, velocity, and acceleration on a moment-to-moment basis. We describe an experimental framework for acquiring pointing actions and automatically fitting the parameters of mathematical models to the empirical data. We present the use of time-series, phase space and Hooke plot visualisations of the experimental data, to gain insight into human pointing dynamics. We find that the identified control models can generate a range of dynamic behaviours that captures aspects of human pointing behaviour to varying degrees. Conditions with a low index of difficulty (ID) showed poorer fit because their unconstrained nature leads naturally to more dynamic variability. We report on characteristics of human surge behaviour in pointing.
We report differences in a number of controller performance measures, including Overshoot, Settling time, Peak time, and Rise time. We describe trade-offs among the models. We conclude that control theory offers a promising complement to Fitts' law based approaches in HCI, with models providing representations and predictions of human pointing dynamics which can improve our understanding of pointing and inform design.
IDA coffee breaks (08 November, 2016)
Speaker: everyone
A chance to catch up informally with members of the IDA section in the Computing Science Common Room.
Analysis of the Cost and Benefits of Search Interactions (07 November, 2016)
Speaker: Dr. Leif Azzopardi
Interactive Information Retrieval (IR) systems often provide various features and functions, such as query suggestions and relevance feedback, that a user may or may not decide to use. The decision to take such an option has associated costs and may lead to some benefit. Thus, a savvy user would take decisions that maximises their net benefit. In this talk, we will go through a number of formal models which examine the costs and benefits of various decisions that users, implicitly or explicitly, make when searching. We consider and analyse the following scenarios: (i) how long a user's query should be? (ii) should the user pose a specific or vague query? (iii) should the user take a suggestion or re-formulate? (iv) when should a user employ relevance feedback? and (v) when would the "find similar" functionality be worthwhile to the user? To this end, we analyse a series of cost-benefit models exploring a variety of parameters that affect the decisions at play. Through the analyses, we are able to draw a number of insights into different decisions, provide explanations for observed behaviours and generate numerous testable hypotheses. This work not only serves as a basis for future empirical work, but also as a template for developing other cost-benefit models involving human-computer interaction.
This talk is based on the recent ICTIR 2016 paper with Guido Zuccon: http://dl.acm.org/citation.cfm?id=2970412
IDA coffee breaks (01 November, 2016)
Speaker: everyone
A chance to catch up informally with members of the IDA section in the Computing Science Common Room.
I'm an information scientist - let me in! (31 October, 2016)
Speaker: Martin White
For the last 46 years Martin has been a professional information scientist, though often in secret. Since founding Intranet Focus Ltd he has found that the awareness of research into topics such as information behaviour, information quality and information seeking in his clients is close to zero. This is especially true in information retrieval. In his presentation Martin will consider why this is the case, what the impact might be and what (if anything) should and could be done to change this situation.
IDA coffee breaks (25 October, 2016)
Speaker: everyone
A chance to catch up informally with members of the IDA section in the Computing Science Common Room.
The problem of quantification in Information Retrieval and on Social Networks. (17 October, 2016)
Speaker: Gianni Amati
There is a growing interest to know how fast information spreads on social networks, how many unique users are participating to an event, the leading opinion polarity in a stream. Quantifying distinct elements on a flow information is thus becoming a crucial problem in many real time information retrieval or streaming applications. We discuss the state-of-art of quantification and show that many problems can be interpreted within a common framework. We introduce a new probabilistic framework for quantification and show as examples how to count opinions in a stream and how to compute the degrees of separation of a network.
Analytics over Parallel Multi-view Data (03 October, 2016)
Speaker: Dr. Deepak Padmanabhan
Conventional unsupervised data analytics techniques have largely focused on processing datasets of single-type data, e.g., one of text, ECG, Sensor Readings and Image data. With increasing digitization, it has become common to have data objects having representations that encompass different "kinds" of information. For example, the same disease condition may be identified through EEG or fMRI data. Thus, a dataset of EEG-fMRI pairs would be considered as a parallel two-view dataset. Datasets of text-image pairs (e.g., a description of a seashore, and an image of it) and text-text pairs (e.g., problem-solution text, or multi-language text from machine translation scenarios) are other common instances of multi-view data. The challenge in multi-view data analytics is about effectively leveraging such parallel multi-view data to perform analytics tasks such as clustering, retrieval and anomaly detection. This talk will cover some emerging trends in processing multi-view parallel data, and different paradigms for the same. In addition to looking at the different schools of techniques, and some specific techniques from each school, this talk will also be used to present some possibilities for future work in this area.
Dr. Deepak Padmanabhan is a lecturer with the Centre for Data Sciences and Scalable Computing at Queen's University Belfast. He obtained his B.Tech in Comp. Sc. and Engg. from Cochin University (Kerala, India), followed by his M.Tech and PhD, all in computer science, from Indian Institute of Technology Madras. Prior to joining Queen's, he was a researcher at IBM Research - India. He has over 40 publications across top venues in Data Mining, NLP, Databases and Information Retrieval. He co-authored a book on Operators for Similarity Search, published by Springer in 2015. He is the author on ~15 patent applications to the USPTO, including 4 granted patents. He is a recipient of the INAE Young Engineer Award 2015, and is a Senior Member of the ACM and the IEEE. His research interests include Machine Learning, Data Mining, NLP, Databases and Information Retrieval. Email: http://member.acm.org/~deepaksp
Improvising minds: Improvisational interaction and cognitive engagement (29 August, 2016)
Speaker: Adam Linson
In this talk, I present my research on improvisation as a general form of adaptive expertise. My interdisciplinary approach takes music as a tractable domain for empirical studies, which I have used to ground theoretical insights from HCI, AI/robotics, psychology, and embodied cognitive science. I will discuss interconnected aspects of digital musical instrument (DMI) interface design a musical robotic AI system, and a music psychology study of sensorimotor influences on perceptual ambiguity. I will also show how I integrate this work with an inference-based model of neural functioning, to underscore implications beyond music. On this basis, I indicate how studies of musical improvisation can shed light on a domain-general capacity: our flexible, context-sensitive responsiveness to rapidly-changing environmental conditions.
Recognizing manipulation actions through visual accelerometer tracking, relational histograms, and user adaptation (26 August, 2016)
Speaker: Sebastian Stein
Activity recognition research in computer vision and pervasive computing has made a remarkable trajectory from distinguishing full-body motion patterns to recognizing complex activities. Manipulation activities as occurring in food preparation are particularly challenging to recognize, as they involve many different objects, non-unique task orders and are subject to personal idiosyncrasies. Video data and data from embedded accelerometers provide complementary information, which motivates an investigation of effective methods for fusing these sensor modalities.
In this talk I present a method for multi-modal recognition of manipulation activities that combines accelerometer data and video at multiple stages of the recognition pipeline. A method for accelerometer tracking is introduced that provides for each accelerometer-equipped object a location estimate in the camera view by identifying a point trajectory that matches well the accelerometer data. It is argued that associating accelerometer data with locations in the video provides a key link for modelling interactions between accelerometer-equipped objects and other visual entities in the scene. Estimates of accelerometer locations and their visual displacements are used to extract two new types of features: (i)
Reference Tracklet Statistics characterizes statistical properties of an accelerometer’s visual trajectory, and (ii) RETLETS, a feature representation that encodes relative motion, uses an accelerometer’s visual trajectory as a reference frame for dense tracklets. In comparison to a traditional sensor fusion approach where features are extracted from each sensor-type independently and concatenated for classification, it is shown that by combining RETLETS and Reference Tracklet Statistics with those sensor-specific features performs considerably better. Specifically addressing scenarios in which a recognition
system would be primarily used by a single person (e.g., cognitive situational support), this thesis investigates three methods for adapting activity models to a target user based on user-specific training data. Via randomized control trials it is shown that these methods indeed learn user idiosyncrasies.
The whole is greater than the sum of its parts: how semantic trajectories and recommendations may help tourism. (22 August, 2016)
Speaker: Dr. Chiara Renso
During the first part of this talk I will overview my recent activity in the field of mobility data mining with particular interest in the study of semantics in trajectory data and the experience with the SEEK Marie Curie project [1] recently concluded. Then I will present two highlights of tourism recommendation works based on the idea of semantic trajectories: TripBuilder [2] and GroupFinder [3]. Tripbuilder is based on the analysis of enriched tourist trajectories extracted from Flickr photos to suggest itineraries constrained by a temporal budget and based on the travellers preferences. The Groupfinder framework recommends a group of friends with whom to enjoy a visit to a place, balancing the friendship relations of the group members with the user individual interests in the destination location.
[1] www.seek-project.eu). She was also coordinator of a bilateral CNR-CNPQ Italy-Brazil project on mobility data mining with Federal University of Cearà. She is author of more than 90 peer-reviewed publications. She is co-editor of the book "Mobility Data: Modelling, Management, and Understanding" edited by Cambridge Press in 2013; co-editor of the special issue for Journal on Knowledge and Information system (KAIS) on Context aware data mining; co-editor of International Journal of Knowledge and Systems Science (IJKSS) on Modelling Tools for Extracting Useful Knowledge and Decision Making. She has been co-chair of three editions of the Workshop on Semantic Aspects of Data Mining in conjunction with IEEE ICDM conference. She is a regular reviewer of ACM CIKM, ACM KDD, ACM SIGSPATIAL and many journals on these topics.
Skin Reading: Encoding Text in a 6-Channel Haptic Display (11 August, 2016)
Speaker: Granit Luzhnica
In this talk I will present a study we performed in to investigate the communication of natural language messages using a wearable haptic display. Our research experiments investigated both the design of the haptic display, as well as the methods for communication that use it. First, three wearable configurations are proposed basing on haptic perception fundamentals and evaluated in the first study. To encode symbols, we use an overlapping spatiotemporal stimulation (OST) method, that distributes stimuli spatially and temporally with a minima gap. Second, we propose an encoding for the entire English alphabet and a training method for letters, words and phrases. A second study investigates communication accuracy. It puts four participants through five sessions, for an overall training time of approximately 5 hours per participant.
Casual Interaction for Smartwatch Feedback and Communication (01 July, 2016)
Speaker: Henning Pohl
Casual interaction strives to enable people to scale back their engagement with interactive systems, while retaining the level of control they desire. In this talk, we will take a look on two recent developments in casual interaction systems. The first p
Casual interaction strives to enable people to scale back their engagement with interactive systems, while retaining the level of control they desire. In this talk, we will take a look on two recent developments in casual interaction systems. The first project to be presented is an indirect visual feedback system for smartwatches. Embedding LEDs into the back of a watch case enabled us to create a form of feedback that is less disruptive than vibration feedback and blends in with the body. We investigated how well such subtle feedback works in an in-the-wild study, which we will take a closer look at in this talk. Where the first project is a more casual form of feedback, the second project tries to support a more casual form of communication: emoji. Over the last years these characters have become more and more popular, yet entering them can take quite some effort. We have developed a novel emoji keyboard around zooming interaction, that makes it easier and faster to enter emoji.
Predicting Ad Quality for Native Advertisements (06 June, 2016)
Speaker: Dr Ke Zhou,
Native advertising is a specific form of online advertising where ads replicate the look-and-feel of their serving platform. In such context, providing a good user experience with the served ads is crucial to ensure long-term user engagement.
In this talk, I will explore the notion of ad quality, namely the effectiveness of advertising from a user experience perspective. I will talk from both the pre-click and post-click perspective for predicting quality for native ads. With respect to pre-click ad quality, we design a learning framework to detect offensive native ads, showing that, to quantify ad quality, ad offensive user feedback rates are more reliable than the commonly used click-through rate metrics. We translate a set of user preference criteria into a set of ad quality features that we extract from the ad text, image and advertiser, and then use them to train a model able to identify offensive ads. In terms of post-click quality, we use ad landing page dwell time as our proxy and exploit various ad landing page features to predict ad landing page with high dwell time.
Efficient Web Search Diversification via Approximate Graph Coverage (25 April, 2016)
Speaker: Carsten Eickhoff
In the case of general or ambiguous Web search queries, retrieval systems rely on result set diversification techniques in order to ensure an adequate coverage of underlying topics such that the average user will find at least one of the returned documents.
Searching for better health: challenges and implications for IR (04 April, 2016)
Speaker: Dr. Guido Zuccon
A talk about why IR researchers should care about health search
In this talk I will discuss research problems and possible solutions related to helping the general public searching for health information online. I will show that although in the first instance this appears to be a domain-specific search task, research problems associated with this task have more general implications for IR and offer opportunities to develop advances that are applicable to the whole research field. In particular, in the talk I will focus on two aspects related to evaluation: (1) the inclusion of multiple dimensions of relevance in the evaluation of IR systems and (2) the modelling of query variations within the evaluation framework.
A Comparison of Primary and Secondary Relevance Judgements for Real-Life Topics (07 March, 2016)
Speaker: Dr Martin Halvey
n this talk I present a user study that examines in detail the differences between primary and secondary assessors on a set of
The notion of relevance is fundamental to the field of Information Retrieval. Within the field a generally accepted conception of relevance as inherently subjective has emerged, with an individual's assessment of relevance influenced by numerous contextual factors. In this talk I present a user study that examines in detail the differences between primary and secondary assessors on a set of "real-world" topics which were gathered specifically for the work. By gathering topics which are representative of the staff and students at a major university, at a particular point in time, we aim to explore differences between primary and secondary relevance judgements for real-life search tasks. Findings suggest that while secondary assessors may find the assessment task challenging in various ways (they generally possess less interest and knowledge in secondary topics and take longer to assess documents), agreement between primary and secondary assessors is high.
Steps towards Profile-Based Web Site Search and Navigation (29 February, 2016)
Speaker: Prof. Udo Kruschwitz
Steps towards Profile-Based Web Site Search and Navigation
Web search in all its flavours has been the focus of research for decades with thousands of highly paid researchers competing for fame. Web site search has however attracted much less attention but is equally challenging. In fact, what makes site search (as well as intranet and enterprise search) even more interesting is that it shares some common problems with general Web search but also offers a good number of additional problems that need to be addressed in order to make search on a Web site no longer a waste of time. At previous visits to Glasgow I talked about turning the log files collected on a Web site into usable, adaptive data structures that can be used in search applications (and which we call user or cohort profiles). This time I will focus on applying these profiles to a navigation scenario and illustrate how the automatically acquired profiles provide a practical use case for combining natural language processing and information retrieval techniques (as that is what we really do at Essex).
Sentiment and Preference Guided Social Recommendation. (22 February, 2016)
Speaker: Yoke Yie Chen
In this talk, I will focus on two knowledge sources for product recommendation: product reviews and user purchase trails.
Social recommender systems harness knowledge from social media to generate recommendations. Previous works in social recommender systems use social knowledge such as social tags, social relationship (social network) and microblogs. In this talk, I will focus on two knowledge sources for product recommendation: product reviews and user purchase trails. In particular, I will present how we exploit the sentiment expressed in product reviews and user preferences which are implicitly contained in user purchase trails as the basis for recommendation.
Recent Advances in Search Result Diversification for the Web and Social Media (17 February, 2016)
Speaker: Ismail Sengor Altingovde
I will focus on the web search result diversification problem and present our novel contributions in the field.
In this talk, I will start with a short potpourri of our most recent research, emphasis being on the topics related to the web search engines and social Web. Then, I will focus on the web search result diversification problem and present our novel contributions in three directions. Firstly, I will present how the normalizaton of query relevance scores can boost the performance of the state-of-the-art explicit diversification strategies. Secondly, I will introduce a set of new explicit diversification strategies based on the score(-based) and rank(-based) aggregation methods. As a third contribution, I will present how query performance prediction (QPP) can be utilized to weight query aspects during diversification. Finally, I will discuss how these diversification methods perform in the context of Tweet search, and how we improve them using word embeddings.
Practical and theoretical problems on the frontiers of multilingual natural language processing (16 February, 2016)
Speaker: Dr Adam Lopez
Multilingual natural language processing (NLP) has been enormously successful over the last decade, highlighted by offerings like Google translate. What is left to do?
Multilingual natural language processing (NLP) has been enormously successful over the last decade, highlighted by offerings like Google translate. What is left to do? I'll focus on two quite different, very basic problems that we don't yet know how to solve. The first is motivated by the development of new, massively-parallel hardware architectures like GPUs, which are especially tantalizing for computation-bound NLP problems, and may open up new possibilities for the application and scale of NLP. The problem is that classical NLP algorithms are inherently sequential, so harnessing the power of such processors requires complete rethinking the fundamentals of the field. The second is motivated by the fact that NLP systems often fail to correctly understand, translate, extract, or generate meaning. We're poised to make serious progress in this area using the reliable method of applying machine learning to large datasets—in this case, large quantities of natural language text annotated with explicit meaning representations, which take the form of directed acyclic graphs. The problem is that probabilities on graphs are surprisingly hard to define. I'll discuss work on both of these fronts.
Information retrieval challenges in conducting systematic reviews (08 February, 2016)
Speaker: Julie Glanville
The presentation will also describe other areas where software such as text mining and machine learning have potential to contribute to the Systematic Review process
Systematic review (SR) is a research method that seeks to provide an assessment of the state of the research evidence on a specific question. Systematic reviews (SRs) aim to be objective, transparent and replicable and seek to minimise bias by means of extensive searches.
The challenges of extensive searching will be summarised. As software tools and internet interconnectivity increase, we are seeing increasing use of a range of tools within the SR process (not only for information retrieval). This presentation will present some of the tools we are currently using within the Cochrane SR community and UK SRs, and the challenges which remain for efficient information retrieval. The presentation will also describe other areas where software such as text mining and machine learning have potential to contribute to the SR process.
Learning to Hash for Large Scale Image Retrieval (14 December, 2015)
Speaker: Sean Moran
In this talk I will introduce two novel data-driven models that significantly improve the retrieval effectiveness of locality sensitive hashing (LSH), a popular randomised algorithm for nearest neighbour search that permits relevant data-points to be ret
In this talk I will introduce two novel data-driven models that significantly improve the retrieval effectiveness of locality sensitive hashing (LSH), a popular randomised algorithm for nearest neighbour search that permits relevant data-points to be retrieved in constant time, independent of the database size.
To cut down the search space LSH generates similar binary hashcodes for similar data-points and uses the hashcodes to index database data-points into the buckets of a set of hashtables. At query time only those data-points that collide in the same hashtable buckets as the query are returned as candidate nearest neighbours. LSH has been successfully used for event detection in large scale streaming data such as Twitter [1] and for detecting 100,000 object classes on a single CPU [2].
The generation of similarity preserving binary hashcodes comprises two steps: projection of the data-points onto the normal vectors of a set of hyperplanes partitioning the input feature space followed by a quantisation step that uses a single threshold to binarise the resulting projections to obtain the hashcodes. In this talk I will argue that the retrieval effectiveness of LSH can be significantly improved by learning the thresholds and hyperplanes based on the distribution of the input data.
In the first part of my talk I will provide a high level introduction of LSH. I will then argue that LSH makes a set of limiting assumptions arising from its data-independence that hamper its retrieval effectiveness. This motivates the second and third parts of my talk in which I introduce two new models that address these limiting assumptions.
Firstly, I will discuss a scalar quantisation model that can learn multiple thresholds per LSH hyperplane using a novel semi-supervised objective function [3]. Optimising this objective function results in thresholds that reduce information loss inherent in converting the real-valued projections to binary. Secondly, I will introduce a new two-step iterative model for learning the hashing hyperplanes [4]. In the first step the hashcodes of training data-points are regularised over an adjacency graph which encourages similar data-points to be assigned similar hashcodes. In the second step a set of binary classifiers are learnt so as to separate opposing bits (0,1) with maximum margin. Repeating both steps iteratively encourages the hyperplanes to evolve into positions that provide a much better bucketing of the input feature space compared to LSH.
For both algorithms I will present a set of query-by-example image retrieval results on standard image collections, demonstrating significantly improved retrieval effectiveness versus state-of-the-art hash functions, in addition to a set of interesting and previously unexpected results.
[1] Sasa Petrovic, Miles Osborne and Victor Lavrenko, Streaming First Story Detection with Application to Twitter, In NAACL'10.
[2] Thomas Dean, Mark Ruzon, Mark Segal, Jonathon Shlens, Sudheendra Vijayanarasimhan, and Jay Yagnik, Fast, Accurate Detection of 100,000 Object Classes on a Single Machine, In CVPR'13.
[3] Sean Moran, Victor Lavrenko and Miles Osborne. Neighbourhood Preserving Quantisation for LSH, In SIGIR'13.
[4] Sean Moran and Victor Lavrenko. Graph Regularised Hashing. In ECIR'15.
An electroencephalograpy (EEG)-based real-time feedback training system for cognitive brain-machine interface (cBMI) (04 November, 2015)
Speaker: Kyuwan Choi
In this presentation, I will present a new cognitive brain-machine interface (cBMI) using cortical activities in the prefrontal cortex. In the cBMI system, subjects conduct directional imagination which is more intuitive than the existing motor imagery. The subjects control a bar on the monitor freely by extracting the information of direction from the prefrontal cortex, and that the subject’s prefrontal cortex is activated by giving them the movement of the bar as feedback. Furthermore, I will introduce an EEG-based wheelchair system using the cBMI concept. If we use the cBMI, it is possible to build a more intuitive BMI system. It could help improve the cognitive function of healthy people and help activate the area around the damaged area of the patients with prefrontal damage such as patients with dementia, autism, etc. by consistently activating their prefrontal cortex.
Adapting biomechanical simulation for physical ergonomics evaluation of new input methods (28 October, 2015)
Speaker: Myroslav Bachynskyi
Recent advances in sensor technology and computer vision allowed new computer input methods to rapidly emerge. These methods are often considered as more intuitive and easier to learn comparing to the conventional keyboard or mouse, however most of them are poorly assessed with respect to their physical ergonomics and health impact of their usage. The main reasons for this are large input spaces provided by these interfaces, absence of a reliable, cheap and easy-to-apply physical ergonomics assessment method and absence of biomechanics expertize in user interface designers. The goal of my research is to develop a physical ergonomics assessment method, which provides support to interface designers on all stages of the design process for low cost and without specialized knowledge. Our approach is to extend biomechanical simulation tools developed for medical and rehabilitation purposes to adapt them for Human-Computer Interaction setting. The talk gives an overview of problems related to the development of the method and shows answers to some of the fundamental questions.
Detecting Swipe Errors on Touchscreens using Grip Modulation (22 October, 2015)
Speaker: Faizuddin Mohd Noor
We show that when users make errors on mobile devices, they make immediate and distinct physical responses that can be observed with standard sensors. We used three
standard cognitive tasks (Flanker, Stroop and SART) to induce errors from 20 participants. Using simple low-resolution capacitive touch sensors placed around a standard device and a built-in accelerometer, we demonstrate that errors can be predicted using micro-adjustments to hand grip and movement in the period after swiping the touchscreen. In a per-user model, our technique predicted error with a mean AUC of 0.71 in Flanker and 0.60 in Stroop and SART using hand grip, while with the accelerometer the mean AUC in all tasks was ≥ 0.90. Using a pooled, non-user-specific, model, our technique achieved mean AUC of 0.75 in Flanker and 0.80 in Stroop and SART using hand grip and an AUC for all tasks > 0.90 for the accelerometer. When combining these features we achieved an AUC of 0.96 (with false accept and reject rates both below 10%). These results suggest that hand grip and movement provide strong and very low latency evidence for mistakes, and could be a valuable component in interaction error detection and correction systems.
A conceptual model of the future of input devices (14 October, 2015)
Speaker: John Williamson
Turning sensor engineering into advances into human computer interaction is slow, ad hoc and unsystematic. I'll discuss a fundamental approach to input device engineering, and illustrate how machine learning could have the exponentially-accelerating impact in HCI that it has had in other fields.
[caveat: This is a proposal: there are only words, not results!]
Haptic Gaze Interaction - EVENT CANCELLED (05 October, 2015)
Speaker: Poika Isokoski
Eye trackers that can be (somewhat) comfortably worn for long periods are now available. Thus, computing systems can track the gaze vector and it can be used in interactions with mobile and embedded computing systems together with other input and output
Eye trackers that can be (somewhat) comfortably worn for long periods are now available. Thus, computing systems can track the gaze vector and it can be used in interactions with mobile and embedded computing systems together with other input and output modalities. However, interaction techniques for these activities are largely missing. Furthermore, it is unclear how feedback from eye movements should be given to best support user's goals. This talk will give an overview of the results of our recent work in exploring haptic feedback on eye movements and building multimodal interaction techniques that utilize the gaze data. I will also discuss some possible future directions in this line of research.
Challenges in Metabolomics, and some Machine Learning Solutions (30 September, 2015)
Speaker: Simon Rogers
Large scale measurement of the metabolites present in an organism is very challenging, but potentially highly rewarding in the understanding of disease and the development of drugs. In this talk I will describe some of the challenges in analysis of data from Liquid Chromatography - Mass Spectrometry, one of the most popular platforms for metabolomics. I will present Statistical Machine Learning solutions to several of these challenges, including the alignment of spectra across experimental runs, the identification of metabolites within the spectra, and finish with some recent work on using text processing techniques to discover conserved metabolite substructures.
Engaging with Music Retrieval (09 September, 2015)
Speaker: Daniel Boland
Music collections available to listeners have grown at a dramatic pace, now spanning tens of millions of tracks. Interacting with a music retrieval system can thus be overwhelming, with users offered ‘too-much-choice’. The level of engagement required for such retrieval interactions can be inappropriate, such as in mobile or multitasking contexts. Using listening histories and work from music psychology, a set of engagement-stratified profiles of listening behaviour are developed. The challenge of designing music retrieval for different levels of user engagement is explored with a system allowing users to denote their level of engagement and thus the specificity of their music queries. The resulting interaction has since been adopted as a component in a commercial music system.
Building Effective and Efficient Information Retrieval Systems (26 June, 2015)
Speaker: Jimmy Lin
Machine learning has become the tool of choice for tackling challenges in a variety of domains, including information retrieval
Machine learning has become the tool of choice for tackling challenges in a variety of domains, including information retrieval. However, most approaches focus exclusively on effectiveness---that is, the quality of system output. Yet, real-world production systems need to search billions of documents in tens of milliseconds, which means that techniques also need to be efficient (i.e., fast). In this talk, I will discuss two approaches to building more effective and efficient information retrieval systems. The first is to directly learn ranking functions that are inherently more efficient---a thread of research dubbed "learning to efficiently rank". The second is through architectural optimizations that take advantage of modern processor architectures---by paying attention to low-level details such as cache misses and branch mispredicts. The combination of both approaches, in essence, allow us to "have our cake and eat it too" in building systems that are both fast and good.
Deep non-parametric learning with Gaussian processes (10 June, 2015)
Speaker: Andreas Damianou
http://staffwww.dcs.sheffield.ac.uk/people/A.Damianou/research/index.html#DeepGPs
This talk will discuss deep Gaussian process models, a recent approach to combining deep probabilistic structures with Bayesian nonparametrics. The obtained deep belief networks are constructed using continuous variables connected with Gaussian process mappings; therefore, the methodology used for training and inference deviates from traditional deep learning paradigms. The first part of the talk will thus outline the associated computational tools, revolving around variational inference. In the second part, we will discuss models obtained as special cases of the deep Gaussian process, namely dynamical / multi-view / dimensionality reduction models and nonparametric autoencoders. The above concepts and algorithms will be demonstrated with examples from computer vision (e.g. high-dimensional video, images) and robotics (motion capture data, humanoid robotics).
Intermittent Control in Man and Machine (30 April, 2015)
Speaker: Henrik Gollee
An intermittent controller generates a sequence of (continuous-time) parametrised trajectories whose parameters are adjusted intermittently, based on continuous observation. This concept is related to "ballistic" control and differs from i) discrete-time control in that the control is not constant between samples, and ii) continuous-time control in that the trajectories are reset intermittently. The Intermittent Control paradigm evolved separately in the physiological and engineering literature. The talk will give details on the experimental verification of intermittency in biological systems and its applications in engineering.
Advantages of intermittent control compared to the continuous paradigm in the context of adaptation and learning will be discussed.
Get A Grip: Predicting User Identity From Back-of-Device Sensing (19 March, 2015)
Speaker: Mohammad Faizuddin Md Noor
We demonstrate that users can be identified using back-of-device handgrip changes during the course of the interaction with mobile phone, using simple, low-resolution capacitive touch sensors placed around a standard device. As a baseline, we replicated the front-of-screen experiments of Touchalytics and compare with our results. We show that classifiers trained using back-of-device could match or exceed the performance of classifiers trained using the Touchalytics approach. Our technique achieved mean AUC, false accept rate and false reject rate of 0.9481, 3.52% and 20.66% for a vertical scrolling reading task and 0.9974, 0.85% and 2.62% for horizontal swiping game task. These results suggest that handgrip provides substantial evidence of user identity, and can be a valuable component of continuous authentication systems.
Towards Effective Non-Invasive Brain-Computer Interfaces Dedicated to Ambulatory Applications (19 March, 2015)
Speaker: Matthieu Duvinage
Disabilities affecting mobility, in particular, often lead to exacerbated isolation and thus fewer communication opportunities, resulting in a limited participation in social life. Additionally, as costs for the health-care system can be huge, rehabilitation-related devices and lower-limb prostheses (or orthoses) have been intensively studied so far. However, although many devices are now available, they rarely integrate the direct will of the patient. Indeed, they basically use motion sensors or the residual muscle activities to track the next move.
Therefore, to integrate a more direct control from the patient, Brain-Computer Interfaces
(BCIs) are here proposed and studied under ambulatory conditions. Basically, a BCI allows you to control any electric device without the need of activating muscles. In this work, the conversion of brain signals into a prosthesis kinematic control is studied following two approaches. First, the subject transmits his desired walking speed to the BCI. Then, this high-level command is converted into a kinematics signal thanks to a Central Pattern Generator (CPG)-based gait model, which is able to produce automatic gait patterns. Our work thus focuses on how BCIs do behave in ambulatory conditions. The second strategy is based on the assumption that the brain is continuously controlling the lower limb. Thus, a direct interpretation, i.e. decoding, from the brain signals is performed. Here, our work consists in determining which part of the brain signals can be used.
Gait analysis from a single ear-worn sensor (17 March, 2015)
Speaker: Delaram Jarchi
Objective assessment of detailed gait patterns is important for clinical applications. One common approach to clinical gait analysis is to use multiple optical or inertial sensors affixed to the patient body for detailed bio-motion and gait analysis. The complexity of sensor placement and issues related to consistent sensor placement have limited these methods only to dedicated laboratory settings, requiring the support of a highly trained technical team. The use of a single sensor for gait assessment has many advantages, particularly in terms of patient compliance, and the possibility of remote monitoring of patients in home environment. In this talk we look into the assessment of a single ear-worn sensor (e-AR sensor) for gait analysis by developing signal processing techniques and using a number of reference platforms inside and outside the gait laboratory. The results are provided considering two clinical applications such as post-surgical follow-up and rehabilitation of orthopaedic patients and investigating the gait changes of the Parkinson's Disease (PD) patients.
Imaging without cameras (05 March, 2015)
Speaker: Matthew Edgar
Conventional cameras rely upon a pixelated sensor to provide spatial resolution. An alternative approach replaces the sensor with a pixelated transmission mask encoded with a series of binary patterns. Combining knowledge of the series of patterns and the associated filtered intensities, measured by single-pixel detectors, allows an image to be deduced through data inversion. At Glasgow we have been extending the concept of a `single-pixel camera' to provide continuous real-time video in excess of 10 Hz, at non-visible wavelengths, using efficient computer algorithms. We have so far demonstrated some applications for our camera such as imaging through smoke, through tinted screens, and detecting gas leaks, whilst performing sub-Nyquist sampling. We are currently investigating the most effective image processing strategies and basis scanning procedures for increasing the image resolution and frame rates for single-pixel video systems.
Analysing UK Annual Report Narratives using Text Analysis and Natural Language Processing (23 February, 2015)
Speaker: Mahmoud El-Haj
In this presentation I will show the work we’ve done in our Corporate Financial Information Environment (CFIE) project.
In this presentation I will show the work we’ve done in our Corporate Financial Information Environment (CFIE) project. The Project, funded by ESRC and ICAEW, seeks to analyse UK financial narratives, their association with financial statement information, and their informativeness for investors using Computational Linguistics, heuristic Information Extraction (IE) and Natural Language Processing (NLP). We automatically collected and analysed a number of 14,000 UK annual reports covering a period between 2002 and 2014 for the UK largest firms listed on the London Stock Exchange. We developed software for this purpose which is available online for general use by academics. The talk includes a demo on the software that we developed and used in our analysis: Wmatrix-import and Wmatrix. Wmatrix-import is a web-based tool to automatically detect and parse the structure of UK annual reports; the tool provides sectioning, word frequency and readability metrics. The output from Wmatrix-import goes as input for further NLP and corpus linguistic analysis by Wmatrix - a web based corpus annotation and retrieval tool which currently supports the analysis of small to medium sized English corpora.
Links:
Wmatrix-import
http://ucrel.lancs.ac.uk/cfie/
Compositional Data Analysis (CoDA) approaches to distance in information retrieval (20 February, 2015)
Speaker: Dr Paul Thomas
Many techniques in information retrieval produce counts from a sample
Many techniques in information retrieval produce counts from a sample, and it is common to analyse these counts as proportions of the whole—term frequencies are a familiar example. Proportions carry only relative information and are not free to vary independently of one another: for the proportion of one term to increase, one or more others must decrease. These constraints are hallmarks of compositional data. While there has long been discussion in other fields of how such data should be analysed, to our knowledge, Compositional Data Analysis (CoDA) has not been considered in IR. In this work we explore compositional data in IR through the lens of distance measures, and demonstrate that common measures, naïve to compositions, have some undesirable properties which can be avoided with composition-aware measures. As a practical example, these measures are shown to improve clustering.
Users versus Models: What observation tells us about effectiveness metrics (16 February, 2015)
Speaker: Dr. Paul Thomas
This work explores the link between users and models by analysing the assumptions and implications of a number of effectiveness metrics, and exploring how these relate to observable user behaviours
Retrieval system effectiveness can be measured in two quite different ways: by monitoring the behaviour of users and gathering data about the ease and accuracy with which they accomplish certain specified information-seeking tasks; or by using numeric effectiveness metrics to score system runs in reference to a set of relevance judgements. In the second approach, the effectiveness metric is chosen in the belief that it predicts ease or accuracy.
This work explores that link, by analysing the assumptions and implications of a number of effectiveness metrics, and exploring how these relate to observable user behaviours. Data recorded as part of a user study included user self-assessment of search task difficulty; gaze position; and click activity. Our results show that user behaviour is influenced by a blend of many factors, including the extent to which relevant documents are encountered, the stage of the search process, and task difficulty. These insights can be used to guide development of batch effectiveness metrics.
Towards Effective Retrieval of Spontaneous Conversational Spoken Content (08 January, 2015)
Speaker: Gareth J. F. Jones
Spoken content retrieval (SCR) has been the focus of various research initiatives for more then 20 years.
Spoken content retrieval (SCR) has been the focus of various research initiatives for more then 20 years. Early research focused on retrieval of clear defined spoken documents principally from the broadcast news domain. The main focus of this work was spoken document retrieval (SDR) task at TREC-6-9. The end of which saw SDR declared a largely solved problem. However, this was soon found to be a premature conclusion relating to controlled recordings of professional news content and overlooking many of the potential challenges of searching more complex spoken content. Subsequent research has focused on more challenging tasks such as search of interview recordings and semi-professional internet content. This talk will begin by reviewing early work in SDR, explaining its successes and limitations, it will then move to outline work exploring SCR for more challenging tasks, such as identifying relevant elements in long spoken recordings such as meetings and presentations, provide a detailed analysis of the characteristics of retrieval behaviour of spoken content elements when indexed using manual and automatic transcripts, and conclude with a summary of the challenges of delivering effective SCR for complex spoken content and initial attempts to address these challenges.
On Inverted Index Compression for Search Engine Efficiency (01 September, 2014)
Speaker: Matteo Catena
Efficient access to the inverted index data structure is a key aspect for a search engine to achieve fast response times to users’ queries. While the performance of an information retrieval (IR) system can be enhanced through the compression of its posting lists, there is little recent work in the literature that thoroughly compares and analyses the performance of modern integer compression schemes across different types of posting information (document ids, frequencies, positions). In this talk, we show the benefit of compression for different types of posting information to the space- and time-efficiency of the search engine. Comprehensive experiments have been conducted on two large, widely used document corpora and large query sets; using different modern integer compression algorithms, integrated into a modern IR system, the Terrier IR platform. While reporting the compression scheme which results in the best query response times, the presented analysis will also show the impact of compression on frequency and position posting information in Web corpora that have large volumes of anchor text.
Interactive Visualisation of Big Music Data. (22 August, 2014)
Speaker: Beatrix Vad
Musical content can be described by a variety of features that are measured or inferred through the analysis of audio data. For a large music collection this establishes the possibility to retrieve information about its structure and underlying patterns. Dimensionality reduction techniques can be used to gain insight into such a high-dimensional dataset and to enable visualisation on two-dimensional screens. In this talk we investigate the usability of these techniques with respect to an interactive exploration interface for large music collections based on moods. A method employing Gaussian Processes to extend the visualisation with additional information about its composition is presented and evaluated
Behavioural Biometrics for Mobile Touchscreen Devices (22 August, 2014)
Speaker: Daniel Buschek
Inference in non‐linear dynamical systems – a machine learning perspective, (08 July, 2014)
Speaker: Carl Rasmussen
Inference in discrete-time non-linear dynamical systems is often done using the Extended Kalman Filtering and Smoothing (EKF) algorithm, which provides a Gaussian approximation to the posterior based on local linearisation of the dynamics. In challenging problems, when the non-linearities are significant and the signal to noise ratio is poor, the EKF performs poorly. In this talk we will discuss an alternative algorithm developed in the machine learning community which is based message passing in Factor Graphs and the Expectation Propagation (EP) approximation. We will show this method provides a consistent and accurate Gaussian approximation to the posterior enabling system identification using Expectation Maximisation (EM) even in cases when the EKF fails.
Adaptive Interaction (02 June, 2014)
Speaker: Professor Andrew Howes
A utility maximization approach to understanding human interaction with technology
This lecture describes a theoretical framework for the behavioural sciences that holds high promise for theory-driven research and design in Human-Computer Interaction. The framework is designed to tackle the adaptive, ecological, and bounded nature of human behaviour. It is designed to help scientists and practitioners reason about why people choose to behave as they do and to explain which strategies people choose in response to utility, ecology, and cognitive information processing mechanisms. A key idea is that people choose strategies so as to maximise utility given constraints. The framework is illustrated with a number of examples including pointing, multitasking, skim- reading, online purchasing, Signal-Detection Theory and diagnosis, and the influence of reputation on purchasing decisions. Importantly, these examples span from perceptual/motor coordination, through cognition to social interaction. Finally, the lecture discusses the challenging idea that people seek to find optimal strategies and also discusses the implications for behavioral investigation in HCI.
Web-scale Semantic Ranking (16 May, 2014)
Speaker: Dr Nick Craswell
Bing Ranking Techniques
Semantic ranking models score documents based on closeness in meaning to the query rather than by just matching keywords. To implement semantic ranking at Web-scale, we have designed and deployed a new multi-level ranking systems that combines the best of inverted index and forward index technologies. I will describe this infrastructure which is currently serving many millions of users and explore several types of semantic models: translation models, syntactic pattern matching and topical matching models. Our experiments demonstrate that these semantic ranking models significantly improve relevance over our existing baseline system. This is the repeat of a WWW2014 industry track talk.
Optimized Interleaving for Retrieval Evaluation (28 April, 2014)
Speaker: Filip Radlinski
Interleaving is an online evaluation technique for comparing the relative quality of information retrieval functions by combining their result lists and tracking clicks. A sequence of such algorithms have been proposed, each being shown to address problems in earlier algorithms. In this talk, I will formalize and generalize this process, while introducing a formal model: After identifying a set of desirable properties for interleaving, I will show that an interleaving algorithm can be obtained as the solution to an optimization problem within those constraints. This approach makes explicit the parameters of the algorithm, as well as assumptions about user behavior. Further, this approach leads to an unbiased and more efficient interleaving algorithm than any previous approach, as I will show a novel log-based analysis of user search behaviour.
Gaussian Processes for Big Data (03 April, 2014)
Speaker: Dr James Hensman
Gaussian Process (GP) models are widely applicable models of functions, and are used extensively in statistics and machine learning for regression, classification and as components of more complex models. Inference in a Gaussian process model usually costs O(n^3) operations, where n is the number of data. In the Big Data (tm) world, it would initially seem unlikely that GPs might contribute due to this computational requirement.
Parametric models have been successfully applied to Big Data (tm) using the Robbins-Monro gradient method, which allows data to be processed individually or in small batches. In this talk, I'll show how these ideas can be applied to Gaussian Processes. To do this, I'll form a variational bound on the marginal likelihood: we discuss the properties of this bound, including the conditions where we recover exact GP behaviour.
Our methods have allowed GP regression on hundreds of thousands of data, using a standard desktop machine. for more details, see http://auai.org/uai2013/prints/papers/244.pdf .
Composite retrieval of heterogeneous web search (24 March, 2014)
Speaker: Horatiu Bota
Traditional search systems generally present a ranked list of documents as answers to user queries. In aggregated search systems, results from different and increasingly diverse verticals (image, video, news, etc.) are returned to users. For instance, many such search engines return to users both images and web documents as answers to the query ``flower''. Aggregated search has become a very popular paradigm. In this paper, we go one step further and study a different search paradigm: composite retrieval. Rather than returning and merging results from different verticals, as is the case with aggregated search, we propose to return to users a set of "bundles", where a bundle is composed of "cohesive'' results from several verticals. For example, for the query "London Olympic'', one bundle per sport could be returned, each containing results extracted from news, videos, images, or Wikipedia. Composite retrieval can promote exploratory search in a way that helps users understand the diversity of results available for a specific query and decide what to explore in more detail.
We proposed and evaluated a variety of approaches to construct bundles that are relevant, cohesive and diverse. We also utilize both entitiy and term as a surrogate to represent items and demonstrate their effectiveness of bridging the "mismatch" gap among heterogeneous sources. Compared with three baselines (traditional "general web only'' ranking, federated search ranking and aggregated search), our evaluation results demonstrate significant performance improvement for a highly heterogeneous web collection.
Query Auto-completion & Composite retrieval (17 March, 2014)
Speaker: Stewart Whiting and Horatiu Bota
=Recent and Robust Query Auto-Completion by Stewart Whiting=
Query auto-completion (QAC) is a common interactive feature that assists users in formulating queries by providing completion suggestions as they type. In order for QAC to minimise the user’s cognitive and physical effort, it must: (i) suggest the user’s intended query after minimal input keystrokes, and (ii) rank the user’s intended query highly in completion suggestions. QAC must be both robust and time-sensitive – that is, able to sufficiently rank both consistently and recently popular queries in completion suggestions. Addressing this trade-off, we propose several practical completion suggestion ranking approaches, including: (i) a sliding window of query popularity evidence from the past 2-28 days, (ii) the query popularity distribution in the last N queries observed with a given prefix, and (iii) short-range query popularity prediction based on recently observed trends. Through real-time simulation experiments, we extensively investigated the parameters necessary to maximise QAC effectiveness for three openly available query log datasets with prefixes of 2-5 characters: MSN and AOL (both English), and Sogou 2008 (Chinese). Results demonstrate consistent and language-independent improvements of up to 9.2% over a non-temporal QAC baseline for all query logs with prefix lengths of 2-3 characters. Hence, this work is an important step towards more effective QAC approaches.
=Composite retrieval of heterogeneous web search by Horatiu Bota=
Traditional search systems generally present a ranked list of documents as answers to user queries. In aggregated search systems, results from different and increasingly diverse verticals (image, video, news, etc.) are returned to users. For instance, many such search engines return to users both images and web documents as answers to the query ``flower''. Aggregated search has become a very popular paradigm. In this paper, we go one step further and study a different search paradigm: composite retrieval. Rather than returning and merging results from different verticals, as is the case with aggregated search, we propose to return to users a set of "bundles", where a bundle is composed of "cohesive'' results from several verticals. For example, for the query "London Olympic'', one bundle per sport could be returned, each containing results extracted from news, videos, images, or Wikipedia. Composite retrieval can promote exploratory search in a way that helps users understand the diversity of results available for a specific query and decide what to explore in more detail.
We proposed and evaluated a variety of approaches to construct bundles that are relevant, cohesive and diverse. We also utilize both entitiy and term as a surrogate to represent items and demonstrate their effectiveness of bridging the "mismatch" gap among heterogeneous sources. Compared with three baselines (traditional "general web only'' ranking, federated search ranking and aggregated search), our evaluation results demonstrate significant performance improvement for a highly heterogeneous web collection.
Studying the performance of semi-structured p2p information retrieval (10 March, 2014)
Speaker: Rami Alkhawaldeh
In recent decades, retrieval systems deployed over peer-to-peer (P2P) overlay networks have been investigated as an alternative to centralised search engines. Although modern search engines provide efficient document retrieval, there are several drawbacks, including: a single point of failure, maintenance costs, privacy risks, information monopolies from search engines companies, and difficulty retrieving hidden documents in the web (i.e. the deep web). P2P information retrieval (P2PIR) systems promise an alternative distributed system to the traditional centralised search engine architecture. Users and creators of web content in such networks have full control over what information they wish to share as well as how they share it.
Researchers have been tackling several challenges to build effective P2PIR systems: (i) collection (peer) representation during indexing, (ii) peer selection during search to route queries to relevant peers and (iii) final peer result merging. Semi-structured P2P networks (i.e, a partially decentralised unstructured overlay network) offer an intermediate design that minimizes the weakness of both centralised and completely decentralised overlay networks and combines the advantages of those two topologies. So, an evaluation framework for this kind of network is necessary to compare the performance of different P2P approaches and to be a guide for developing new and more powerful approaches. In this work, we study the performance of three cluster-based semi-structured P2PIR models and explain the effectiveness of several important design considerations and parameters on retrieval performance, as well as the robustness of these types of network.
4pm @ Level 4
Inside The World’s Playlist (23 February, 2014)
Speaker: Manos Tsagkias
We describe the algorithms behind Streamwatchr, a real-time system for analyzing the music listening behavior of people around the world. Streamwatchr collects music-related tweets, extracts artists and songs, and visualises the results in two ways: (i)~currently trending songs and artists, and (ii)~newly discovered songs.
Machine Learning for Back-of-the-Device Multitouch Typing (17 December, 2013)
Speaker: Daniel Buschek
IDI Seminar: Machine Learning for Back-of-the-Device Multitouch Typing (17 December, 2013)
Speaker: Daniel Buscheck
Dublin City Search: An evolution of search to incorporate city data (24 November, 2013)
Speaker: Dr Veli Bicer, IBM Research Dublin
ors, devices, social networks, governmental applications, or service networks. In such a diversity of information, answering specific information needs of city inhabitants requires holistic information retrieval techniques, capable of harnessing differen
Dr Veli Bicer is a researcher at Smarter Cities Technology Center of IBM Research in Dublin. His research interests include semantic data management, semantic search, software engineering and statistical relational learning. He obtained his PhD from Karlsruhe Institute of Technology, Karlsruhe, Germany and B.Sc. and M.Sc. degrees in computer engineering from Middle East Technical University, Ankara,
IDI Seminar: Uncertain Text Entry on Mobile Devices (21 November, 2013)
Speaker: Daryl Weir
Modern mobile devices typically rely on touchscreen keyboards for input. Unfortunately, users often struggle to enter text accurately on virtual keyboards. We undertook a systematic investigation into how to best utilize probabilistic information to improve these keyboards. We incorporate a state-of-the-art touch model that can learn the tap idiosyncrasies of a particular user, and show in an evaluation that character error rate can be reduced by up to 7% over a baseline, and by up to 1.3% over a leading commercial keyboard. We furthermore investigate how users can explicitly control autocorrection via how hard they touch.
Economic Models of Search (18 November, 2013)
Speaker: Leif Azzopardi
TBA
Predicting Screen Touches From Back-of-Device Grip Changes (14 November, 2013)
Speaker: Faizuddin Mohd Noor
We demonstrate that front-of-screen targeting on mobile phones can be predicted from back-of-device grip manipulations. Using simple, low-resolution capacitive touch sensors placed around a standard phone, we outline a machine learning approach to modelling the grip modulation and inferring front-of-screen touch targets. We experimentally demonstrate that grip is a remarkably good predictor of touch, and we can predict touch position 200ms before contact with an accuracy of 18mm.
IDI Seminar: Predicting Screen Touches From Back-of-Device Grip Changes (14 November, 2013)
Speaker: Faizuddin Mohd Noor
We demonstrate that front-of-screen targeting on mobile phones can be predicted from back-of-device grip manipulations. Using simple, low-resolution capacitive touch sensors placed around a standard phone, we outline a machine learning approach to modelling the grip modulation and inferring front-of-screen touch targets. We experimentally demonstrate that grip is a remarkably good predictor of touch, and we can predict touch position 200ms before contact with an accuracy of 18mm.
Online Learning in Explorative Multi Period Information Retrieval (11 November, 2013)
Speaker: Marc Sloan
In Multi Period Information Retrieval we consider retrieval as a stochastic yet controllable process, the ranking action during the process continuously controls the retrieval system's dynamics and an optimal ranking policy is found in order to maximise the overall users' satisfaction. Different aspects of this process can be fixed giving rise to different search scenarios. One such application is to fix search intent and learn from a population of users over time. Here we use a multi-armed bandit algorithm and apply techniques from finance to learn optimally diverse and explorative search results for a query. We can also fix the user and dynamically model the search over multiple pages of results using relevance feedback. Likewise we are currently investigating using the same technique over session search using a Markov Decision Process.
Stopping Information Search: An fMRI Investigation (04 November, 2013)
Speaker: Eric Walden
Information search has become an increasingly important factor in people's use of information systems. In both personal and workplace environments, advances in information technology and the availability of information have enabled people to perform far more search and access much more information for decision making than in the very recent past. One consequence of this abundance of information has been an increasing need for people to develop better heuristic methods for stopping search, since information available for most decisions now overwhelms people's cognitive processing capabilities and in some cases is almost infinite. Information search has been studied in much past research, and cognitive stopping rules have also been investigated. The present research extends and expands on previous behavioral research by investigating brain activation during searching and stopping behavior using functional Magnetic Resonance Imaging (fMRI) techniques. We asked subjects to search for information about consumer products and to stop when they believed they had enough information to make a subsequent decision about whether to purchase that product. They performed these tasks while in an MRI machine. Brain scans were taken that measured brain activity throughout task performance. Results showed that different areas of the brain were active for searching and stopping, that different brain regions were used for several different self-reported stopping rules, that stopping is a neural correlate of inhibition, suggesting a generalized stopping mechanism in the brain, and that certain individual difference variables make no difference in brain regions active for stopping. The findings extend our knowledge of information search, stopping behavior, and inhibition, contributing to both the information systems and neuroscience literatures. Implications of our findings for theory and practice are discussed.
Towards Technically assisted Sensitivity Review of UK Digital Public Records (21 October, 2013)
Speaker: Tim Gollins
There are major difficulties involved in identifying sensitive information in digital public records. These difficulties, if not addressed, will together with the challenge of managing the risks of failing to identify sensitive documents, force government departments into the precautionary closure of large swaths of digital records. Such closures will inhibit timely, open and transparent access by citizens and others in civic society. Precautionary closures will also prevent social scientists’ and contemporary historians’ access to valuable qualitative information, and their ability to contextualise studies of emerging large scale quantitative data. Closely analogous problems exist in UK local authorities, the third sector, and in other countries which are covered by the same or similar legislation and regulation. In 2012, having conducted investigations and earlier research into this problem, and with new evidence of immediate need emerging from the 20 year rule transition process, The UK National Archives (TNA) highlighted this serious issue facing government departments in the UK Public Records system; the Abaca project is the response.
The talk will outline the role of TNA, the background to sensitivity review, the impact of the move to born digital records, the nature of the particular challenge of reviewing them for sensitivity, and the broad approach that the Abaca Project is taking.
Next Monday, 4pm at 423
Accelerating research on big datasets with Stratosphere (14 October, 2013)
Speaker: Moritz Schubotz
Stratosphere is a research project investigating new paradigms for scalable, complex analytics on massively-parallel data sets.
Stratosphere is a research project investigating new paradigms for scalable, complex analytics on massively-parallel data sets. The core concept of Stratosphere is the PACT programming model that extends MapReduce with second order functions like Match, CoGroup and Cross, which allows researchers to describe complex analytics task naturally. The result are directed acyclic that are optimized for parallel execution, by a cost based optimizer that incorporates user code properties, and executed by the Nephele Data Flow Engine. Nephele is a massively parallel data flow engine dealing with resource management, work scheduling, communication, and fault tolerance.
In the seminar session we introduce and showcase how researchers can set their working environment quickly and start doing research right away. As a proof of concept, we present how a simple java program parallelized optimized by Stratosphere obtained top results at the "exotic" Math search task at NTCIR-10. While other research groups optimized index structures and data formats and waited several hours for their indices to be build on high end hardware, we could focus on the essential program logic use basic data types and run the experiments on a heterogenous desktop cluster in several minutes.
IDI Seminar: Around-device devices: utilizing space and objects around the phone (07 October, 2013)
Speaker: Henning Pohl
For many people their phones have become their main everyday tool. While phones can fulfill many different roles, they also require users to (1) make do with affordance not specialized for the specific task, and (2) closely engage with the device itself. In this talk, I propose utilizing the space and objects around the phone to offer better task affordance and to create an opportunity for casual interactions. Around-device devices are a class of interactors, that do not require the user to bring special tangibles, but repurpose items already found in the user’s surroundings. I'll present a survey study, where we determined which places and objects are available to around-device devices. I'll also talk about a prototype implementation of hand interactions and object tracking for future mobiles with built-in depth sensing.
IDI Seminar: Extracting meaning from audio – a machine learning approach (03 October, 2013)
Speaker: Jan Larsen
Validity and Reliability in Cranfield-like Evaluation in Information Retrieval (23 September, 2013)
Speaker: Julián Urbano
The Cranfield paradigm to Information Retrieval evaluation has been used for half a century now as the means to compare retrieval techniques and advance the state of the art accordingly. However, this paradigm makes certain assumptions that remain a research problem in Information Retrieval and that may invalidate our experimental results.
In this talk I will approach the Cranfield paradigm as an statistical estimator of certain probability distributions that describe the final user experience. These distributions are estimated with a test collection, which actually computes system-related distributions that are assumed to be correlated with the target user-related distributions. From the point of view of validity, I will discuss the strength of that correlation and how it affects the conclusions we draw from an evaluation experiment. From the point of view of reliability, I will discuss on past and current practice to measure the reliability of test collections and review several of them accordingly.
Exploration and contextualization: towards reusable tools for the humanities. (16 September, 2013)
Speaker: Marc Bron
The introduction of new technologies, access to large electronic
cultural heritage repositories, and the availability of new
information channels continues to change the way humanities
researchers work and the questions they seek to answer. In this talk I
will discuss how the research cycle of humanities researchers has been
affected by these changes and argue for the continued development of
interactive information retrieval tools to support the research
practices of humanities researchers. Specifically, I will focus on two
phases in the humanities research cycle: the exploration phase and
contextualization phase. In the first part of the talk I discuss work
on the development and evaluation of search interfaces aimed at
supporting exploration. In the second part of the talk I will focus on
how information retrieval technology focused on identifying relations
between concepts may be used to develop applications that support
contextualization.
Quantum Language Models (19 August, 2013)
Speaker: Alessandro Sordoni
A joint analysis of both Vector Space and Language Models for IR
using the mathematical framework of Quantum Theory revealed how both
models allocate the space of density matrices. A density matrix is
shown to be a general representational tool capable of leveraging
capabilities of both VSM and LM representations thus paving the way
for a new generation of retrieval models. The new approach is called
Quantum Language Modeling (QLM) and has shown its efficiency and
effectiveness in modeling term dependencies for Information
Retrieval.
Toward Models and Measures of Findability (21 July, 2013)
Speaker: Colin Wilkie
A summary of the work being undertaken on Findability
In this 10 minute talk, I will provide an overview of the project I am working on, which is about Findability, and review some of the existing models and measures of findability, before outlining the models that I have working on.
How cost affects search behaviour (21 July, 2013)
Speaker: Leif Azzopardi
Find out about how microeconomic theory predicts user behaviour...
In this talk, I will run through the work I will be presenting at SIGIR on "How cost affects search behavior". The empirical analysis is motivated and underpinned using the Search Economic Theory that I proposed at SIGIR 2011.
[SICSA DVF] Language variation and influence in social media (15 July, 2013)
Speaker: Dr. Jacob Eisenstein
Dr. Eisenstein works on statistical natural language processing, focusing on social media analysis, discourse, and latent variable models
Languages vary by speaker and situation, and change over time. While variation and change are inhibited in written corpora such as news text, they are endemic to social media, enabling large-scale investigation of language's social and temporal dimensions. The first part of this talk will describe a method for characterizing group-level language differences, using the Sparse Additive Generative Model (SAGE). SAGE is based on a re-parametrization of the multinomial distribution that is amenable to sparsity-inducing regularization and facilitates joint modeling across many author characteristics. The second part of the talk concerns change and influence. Using a novel dataset of geotagged word counts, we induce a network of linguistic influence between cities, aggregating across thousands of words. We then explore the demographic and geographic factors that drive spread of new words between cities. This work is in collaboration with Amr Ahmed, Brendan O'Connor, Noah A. Smith, and Eric P. Xing.
Biography
Jacob Eisenstein is an Assistant Professor in the School of Interactive Computing at Georgia Tech. He works on statistical natural language processing, focusing on social media analysis, discourse, and latent variable models. Jacob was a Postdoctoral researcher at Carnegie Mellon and the University of Illinois. He completed his Ph.D. at MIT in 2008, winning the George M. Sprowls dissertation award.
The Use of Correspondence Analysis in Information Retrieval (11 July, 2013)
Speaker: Dr Taner Dincer
This presentation will introduce the application of Correspondence Analysis in Information Retrieval
This presentation will introduce the application of Correspondence Analysis (CA) to Information Retrieval. CA is a well-established multivariate, statistical, exploratory data analysis technique. Multivariate data analysis techniques usually operate on a rectangular array of real numbers called a data matrix whose rows represent r observations (for example, r terms/words in documents) and columns represent c variables (for the example, c documents, resulting in a rxc term-by-document matrix). Multivariate data analysis refers to analyze the data in a manner that takes into account the relationships among observations and also among variables. In contrast to univariate statistics, it is concerned with the joint nature of measurements. The objective of exploratory data analysis is to explore the relationships among objects and among variables over measurements for the purpose of visual inspection. In particular, by using CA one can visually study the “Divergence From Independence” (DFI) among observations and among variables.
For Information Retrieval (IR), CA can serve three different uses: 1) As an analysis tool to visually inspect the results of information retrieval experiments, 2) As a basis to unify the probabilistic approaches to term weighting problem such as Divergence From Randomness and Language Models, and 3) As a term weighting model itself, "term weighting based on measuring divergence from independence". In this presentation, the uses of CA for these three purposes are exemplified.
A study of Information Management in the Patient Surgical Pathway in NHS Scotland (03 June, 2013)
Speaker: Matt-Mouley Bouamrane
We conducted a study of information management processes across the patient surgical pathway in NHS Scotland. While the majority of General Practitioners (GPs) consider electronic information systems as an essential and integral part of their work during the patient consultation, many were not fully satisfied with the functionalities of these systems. A majority of GPs considered that the national eReferral system streamlined referral processes. Almost all GPs reported marked variability in the quality of discharge information. Preoperative processes vary significantly across Scotland, with most services using paper based systems. There is insufficient use made of information provided through the patient electronic referral and a considerable duplication of effort with the work already performed in primary care. Three health-boards have implemented electronic preoperative information systems. These have transformed clinical practices and facilitated communication and information-sharing among the multi-disciplinary team and within the health boards. Substantial progress has been made towards improving information transfer and sharing within the surgical pathway in recent years but there remains scope for further improvements at the interface between services.
Interdependence and Predictability of Human Mobility and Social Interactions (23 May, 2013)
Speaker: Mirco Musolesi
The study of the interdependence of human movement and social ties of individuals is one of the most interesting research areas in computational social science. Previous studies have shown that human movement is predictable to a certain extent at different geographic scales. One of the open problems is how to improve the prediction exploiting additional available information. In particular, one of the key questions is how to characterise and exploit the correlation between movements of friends and acquaintances to increase the accuracy of the forecasting algorithms.
In this talk I will discuss the results of our analysis of the Nokia Mobile Data Challenge dataset showing that, by means of multivariate nonlinear predictors, it is possible to exploit mobility data of friends in order to improve user movement forecasting. This can be seen as a process of discovering correlation patterns in networks of linked social and geographic data. I will also show how mutual information can be used to quantify this correlation; I will demonstrate how to use this quantity to select individuals with correlated mobility patterns in order to improve movement prediction. Finally, I will show how the exploitation of data related to friends improves dramatically the prediction with respect to the case of information of people that do not have social ties with the user.
Discovering, Modeling, and Predicting Task-by-Task Behaviour of Search Engine Users (20 May, 2013)
Speaker: Salvatore Orlando
Users of web search engines are increasingly issuing queries to accomplish their daily tasks (e.g., “finding a recipe”, “booking a flight”, “read- ing online news”, etc.). In this work, we propose a two-step methodology for discovering latent tasks that users try to perform through search engines. Firstly, we identify user tasks from individual user sessions stored in query logs. In our vision, a user task is a set of possibly non-contiguous queries (within a user search session), which refer to the same need. Secondly, we discover collective tasks by aggregating similar user tasks, possibly performed by distinct users. To discover tasks, we propose to adopt clustering algorithms based on novel query similarity functions, in turn obtained by exploiting specific features, and both unsupervised and supervised learning approaches. All the proposed solutions were evaluated on a manually-built ground-truth.
Furthermore, we introduce the the Task Relation Graph (TGR) as a representation of users' search behaviors on a task-by-task perspective, by exploiting the collective tasks obtained so far. The task-by-task behavior is captured by weighting the edges of TGR with a relatedness score computed between pairs of tasks, as mined from the query log. We validated our approach on a concrete application, namely a task recommender system, which suggests related tasks to users on the basis of the task predictions derived from the TGR. Finally, we showed that the task recommendations generated by our technique are beyond the reach of existing query suggestion schemes, and that our solution is able to recommend tasks that user will likely perform in the near future.
Work in collaboration with Claudio Lucchese, Gabriele Tolomei, Raffaele Perego, and Fabrizio Silvestri.
References:
[1] C. Lucchese, S. Orlando, R. Perego, F. Silvestri, G. Tolomei. "Identifying Task-based Sessions in Search Engine Query Logs". Forth ACM Int.l Conference on Web Search and Data Mining (WSDM 2011), Hong Kong, February 9-12, 2011
[2] C. Lucchese, S. Orlando, R. Perego, F. Silvestri, G. Tolomei. "Discovering Tasks from Search Engine Query Logs", To appear on ACM Transactions on Information Systems (TOIS).
[3] C. Lucchese, S. Orlando, R. Perego, F. Silvestri, G. Tolomei. "Modeling and Predicting the Task-by-Task Behavior of Search Engine Users". To appear in Proc. OAIR 2013, Int.l Conference in the RIAO series.
Personality Computing (13 May, 2013)
Speaker: Alessandro Vinciarelli
Personality is one of the driving factors behind everything we do and experience
in life. During the last decade, the computing community has been showing an ever
increasing interest for such a psychological construct, especially when it comes
to efforts aimed at making machines socially intelligent, i.e. capable of interacting with
people in the same way as people do. This talk will show the work being done in this
area at the School of Computing Science. After an introduction to the concept of
personality and its main applications, the presentation will illustrate experiments
on speech based automatic perception and recognition. Furthermore, the talk will
outline the main issues and challenges still open in the domain.
Fast and Reliable Online Learning to Rank for Information Retrieval (06 May, 2013)
Speaker: Katja Hoffman
Online learning to rank for information retrieval (IR) holds promise for allowing the development of "self-learning search engines" that can automatically adjust to their users. With the large amount of e.g., click data that can be collected in web search settings, such techniques could enable highly scalable ranking optimization. However, feedback obtained from user interactions is noisy, and developing approaches that can learn from this feedback quickly and reliably is a major challenge.
In this talk I will present my recent work, which addresses the challenges posed by learning from natural user interactions. First, I will detail a new method, called Probabilistic Interleave, for inferring user preferences from users' clicks on search results. I show that this method allows unbiased and fine-grained ranker comparison using noisy click data, and that this is the first such method that allows the effective reuse of historical data (i.e., collected for previous comparisons) to infer information about new rankers. Second, I show that Probabilistic Interleave enables new online learning to rank approaches that can reuse historical interaction data to speed up learning by several orders of magnitude, especially under high levels of noise in user feedback. I conclude with an outlook on research directions in online learning to rank for IR, that are opened up by our results.
Entity Linking for Semantic Search (29 April, 2013)
Speaker: Edgar Meij
Semantic annotations have recently received renewed interest with the explosive increase in the amount of textual data being produced, the advent of advanced NLP techniques, and the maturing of the web of data. Such annotations hold the promise for improving information retrieval algorithms and applications by providing means to automatically understand the meaning of a piece of text. Indeed, when we look at the level of understanding that is involved in modern-day search engines (on the web or otherwise), we come to the obvious conclusion that there is still a lot of room for improvement. Although some recent advances are pushing the boundaries already, information items are still retrieved and ordered mainly using their textual representation, with little or no knowledge of what they actually mean. In this talk I will present my recent and ongoing work, which addresses the challenges associated with leveraging semantic annotations for intelligent information access. I will introduce a recently proposed method for entity linking and show how it can be applied to several tasks related to semantic search on collections of different types, genres, and origins.
Flexible models for high-dimensional probability distributions (04 April, 2013)
Speaker: Iain Murray
Statistical modelling often involves representing high-dimensional probability distributions. The textbook baseline methods, such as mixture models (non-parametric Bayesian or not), often don’t use data efficiently. Whereas the machine learning literature has proposed methods, such as Gaussian process density models and undirected neural network models, that are often too computationally expensive to use. Using a few case-studies, I will argue for increased use of flexible autoregressive models as a strong baseline for general use.
Query Classification for a Digital Library (18 March, 2013)
Speaker: Deirdre Lungley
The motivation for our query classification is the insight it gives the digital content provider into what his users are searching for and hence how his collection could be extended. This talk details two query classification methodologies we have implemented as part of the GALATEAS project (http://www.galateas.eu/): one log-based, the other using wikified queries to learn a Labelled LDA model. An analysis of their respective classification errors indicates the method best suited to particular category groups.
Reusing Historical Interaction Data for Faster Online Learning to Rank for IR (12 March, 2013)
Speaker: Anne Schuth
Online learning to rank for information retrieval (IR) holds promise for allowing the development of ³self-learning² search engines that can automatically adjust to their users. With the large amount of e.g., click data that can be collected in web search settings, such techniques could enable highly scalable ranking optimization. However, feedback obtained from user interactions is noisy, and developing approaches that can learn from this feedback quickly and reliably is a major challenge.
In this paper we investigate whether and how previously collected (historical) interaction data can be used to speed up learning in online learning to rank for IR. We devise the first two methods that can utilize historical data (1) to make feedback available during learning more reliable and (2) to preselect candidate ranking functions to be evaluated in interactions with users of the retrieval system. We evaluate both approaches on 9 learning to rank data sets and find that historical data can speed up learning, leading to substantially and significantly higher online performance. In particular, our preselection method proves highly effective at compensating for noise in user feedback. Our results show that historical data can be used to make online learning to rank for IR much more effective than previously possible, especially when feedback is noisy.
Scientific Lenses over Linked Data: Identity Management in the Open PHACTS project (11 March, 2013)
Speaker: Alasdair Gray,
Scientific Lenses over Linked Data: Identity Management in the Open PHACTS project
Alasdair Gray, University of Manchester
The discovery of new medicines requires pharmacologists to interact with a number of information sources ranging from tabular data to scientific papers, and other specialized formats. The Open PHACTS project, a collaboration of research institutions and major pharmaceutical companies, has developed a linked data platform for integrating multiple pharmacology datasets that form the basis for several drug discovery applications. The functionality offered by the platform has been drawn from a collection of prioritised drug discovery business questions created as part of the Open PHACTS project. Key features of the linked data platform are:
1) Domain specific API making drug discovery linked data available for a diverse range of applications without requiring the application developers to become knowledgeable of semantic web standards such as SPARQL;
2) Just-in-time identity resolution and alignment across datasets enabling a variety of entry points to the data and ultimately to support different integrated views of the data;
3) Centrally cached copies of public datasets to support interactive response times for user-facing applications.
Within complex scientific domains such as pharmacology, operational equivalence between two concepts is often context-, user- and task-specific. Existing linked data integration procedures and equivalence services do not take the context and task of the user into account. We enable users of the Open PHACTS platform to control the notion of operational equivalence by applying scientific lenses over linked data. The scientific lenses vary the links that are activated between the datasets which affects the data returned to the user
Bio
Alasdair is a researcher in the MyGrid team at the University of Manchester. He is currently working on the Open PHACTS project which is building an Open Pharmacological Space to integrate drug discovery data. Alasdair gained his PhD from Heriot-Watt University, Edinburgh, and then worked as a post-doctoral researcher in the Information Retrieval Group at the University of Glasgow. He has spent the last 10 years working on novel knowledge management projects investigating issues of relating data sets.
http://www.cs.man.ac.uk/~graya/
Modelling Time & Demographics in Search Logs (01 March, 2013)
Speaker: Milad Shokouhi
Knowing users' context offers a great potential for personalizing web search results or related services such as query suggestion and query completion. Contextual features cover a wide range of signals; query time, user’s location, search history and demographics can all be regarded as contextual features that can be used for search personalization.
In this talk, we’ll focus on two main questions:
1) How can we use the existing contextual features, in particular time, for improving search results (Shokouhi & Radinsky, SIGIR’12).
2) How can we infer missing contextual features, in particular user-demographics, based on search history (Bi et al., WWW2013).
Our results confirm that (1) contextual features matter and (2) that many of them can be inferred from search history.
Pre-interaction Identification By Dynamic Grip Classification (28 February, 2013)
Speaker: Faizuddin Mohd Noor
We present a novel authentication method to identify users at they pick up a mobile device. We use a combination of back-of-device capacitive sensing and accelerometer measurements to perform classification, and obtain increased performance compared to previous accelerometer-only approaches. Our initial results suggest that users can be reliably identified during the pick-up movement before interaction commences.
Time-Biased Gain (21 February, 2013)
Speaker: Charlie Clark
Time-biased gain provides a unifying framework for information retrieval evaluation
Time-biased gain provides a unifying framework for information retrieval evaluation, generalizing many traditional effectiveness measures while accommodating aspects of user behavior not captured by these measures. By using time as a basis for calibration against actual user data, time-biased gain can reflect aspects of the search process that directly impact user experience, including document length, near-duplicate documents, and summaries. Unlike traditional measures, which must be arbitrarily normalized for averaging purposes, time-biased gain is reported in meaningful units, such as the total number of relevant documents seen by the user. In work reported at SIGIR 2012, we proposed and validated a closed-form equation for estimating time-biased gain, explored its properties, and compared it to standard approaches. In work reported at CIKM 2012, we used stochastic simulation to numerically approximate time-biased gain, an approach that provides greater flexibility, allowing us to accommodate different types of user behavior and increases the realism of the effectiveness measure. In work reported at HCIR 2012, we extended our stochastic simulation to model the variation between users. In this talk, I will provide an overview of time-biased gain, and outline our ongoing and future work, including extensions to evaluate query suggestion, diversity, and whole-page relevance. This is joint work with Mark Smucker.
Evaluating Bad Query Abandonment in an Iterative SMS-Based FAQ Retrieval System (14 February, 2013)
Speaker: Edwin Thuma
We investigate how many iterations users are willing to tolerate in an iterative Frequently Asked Question (FAQ) system that provides information on HIV/AIDS. This is part of work in progress that aims to develop an automated Frequently Asked Question system that can be used to provide answers on HIV/AIDS related queries to users in Botswana. Our system engages the user in the question answering process by following an iterative interaction approach in order to avoid giving inappropriate answers to the user. Our findings provide us with an indication of how long users are willing to engage with the system. We subsequently use this to develop a novel evaluation metric to use in future developments of the system. As an additional finding, we show that the previous search experience of the users has a significant effect on their future behaviour.
[IR] Searching the Temporal Web: Challenges and Current Approaches (04 February, 2013)
Speaker: Nattiya Kanhabua
In this talk, we will give a survey of current approaches to searching the
temporal web. In such a web collection, the contents are created and/or
edited over time, and examples are web archives, news archives, blogs,
micro-blogs, personal emails and enterprise documents. Unfortunately,
traditional IR approaches based on term-matching only can give
unsatisfactory results when searching the temporal web. The reason for this
is multifold: 1) the collection is strongly time-dependent, i.e., with
multiple versions of documents, 2) the contents of documents are about
events happened at particular time periods, 3) the meanings of semantic
annotations can change over time, and 4) a query representing an information
need can be time-sensitive, so-called a temporal query.
Several major challenges in searching the temporal web will be discussed,
namely, 1) How to understand temporal search intent represented by
time-sensitive queries? 2) How to handle the temporal dynamics of queries
and documents? and 3) How to explicitly model temporal information in
retrieval and ranking models? To this end, we will present current
approaches to the addressed problems as well as outline the directions for
future research.
Probabilistic rule-based argumentation for norm-governed learning agents (28 January, 2013)
Speaker: Sebastian Riedel
There is a vast and ever-increasing amount of unstructured textual data at our disposal. The ambiguity, variability and expressivity of language makes this data difficult to analyse, mine, search, visualise, and, ultimately, base decisions on. These challenges have motivated efforts to enable machine reading: computers that can read text and convert it into semantic representations, such as the Google Knowledge Graph for general facts, or pathway databases in the biomedical domain. This representations can then be harnessed by machines and humans alike. At the heart of machine reading is relation extraction: reading text to create a semantic network of entities and their relations, such as employeeOf(Person,Company), regulates(Protein,Protein) or causes(Event,Event).
In this talk I will present a series of graphical models and matrix factorisation techniques that can learn to extract relations. I will start by contrasting a fully supervised approach with one that leverages pre-existing semantic knowledge (for example, in the Freebase database) to reduce annotation costs. I will then present ways to extract additional relations that are not yet part of the schema, and for which no pre-existing semantic knowledge is available. I will show that by doing so we cannot only extract richer knowledge, but also improve extraction quality of relations within the original schema. This helps to improve over previous state-of-the-art by more than 10% points mean average precision.
IDI Seminar (29 November, 2012)
Speaker: Konstantinos Georgatzis
Efficient Optimisation for Data Visualisation as an Information Retrieval Task
Visualisation of multivariate data sets is often done by mapping data onto a low-dimensional display with nonlinear dimensionality reduction (NLDR) methods. We have introduced a formalism where NLDR for visualisation is treated as an information retrieval task, and a novel NLDR method called the Neighbor Retrieval
Visualiser (NeRV) which outperforms previous methods. The remaining concern is that NeRV has quadratic computational complexity with respect to the number of data. We introduce an efficient learning algorithm for NeRV where relationships between data are approximated through mixture modeling, yielding efficient computation with near-linear computational complexity with respect to the number of data. The method is much faster to optimise as the number of data grows, and it maintains good visualisation performance.
Context data in lifelog retrieval (19 November, 2012)
Speaker: Liadh Kelly
Context data in lifelog retrieval
Advances in digital technologies for information capture combined with
massive increases in the capacity of digital storage media mean that it is
now possible to capture and store much of one's life experiences in a
personal lifelog. Information can be captured from a myriad of personal
information devices including desktop computers, mobile phones, digital
cameras, and various sensors, including GPS, Bluetooth, and biometric
devices. This talk centers on the investigation of the challenges of
retrieval in this emerging domain and on the examination of the utility of
several implicitly recorded and derived context types in meeting these
challenges. For these investigations unique rich multimodal personal
lifelog collections of 20 months duration are used. These collections
contain all items accessed on subjects' PCs and laptops (email, web pages,
word documents, etc), passively captured images depicting subjects' lives
using the SenseCam device (http://research.microsoft.com/sensecam), and
mobile text messages sent and received. Items are annotated with several
rich sources of automatically derived context data types including
biometric data (galvanic skin response, heart rate, etc), geo-location
(captured using GPS data), people present (captured using Bluetooth data),
weather conditions, light status, and several context types related to the
dates and times of accesses to items.
From Search to Adaptive Search (12 November, 2012)
Speaker: Udo Kruschwitz
Generating good query modification suggestions or alternative queries to assist a searcher remains however a challenging issue
Modern search engines have been moving away from very simplistic interfaces that aimed at satisfying a user's need with a single-shot query. Interactive features such as query suggestions and faceted search are now integral parts of Web search engines. Generating good query modification suggestions or alternative queries to assist a searcher remains however a challenging issue. Query log analysis is one of the major strands of work in this direction. While much research has been performed on query logs collected on the Web as a whole, query log analysis to enhance search on smaller and more focused collections (such as intranets, digital libraries and local Web sites) has attracted less attention. The talk will look at a number of directions we have explored at the University of Essex in addressing this problem by automatically acquiring continuously updated domain models using query and click logs (as well as other sources).